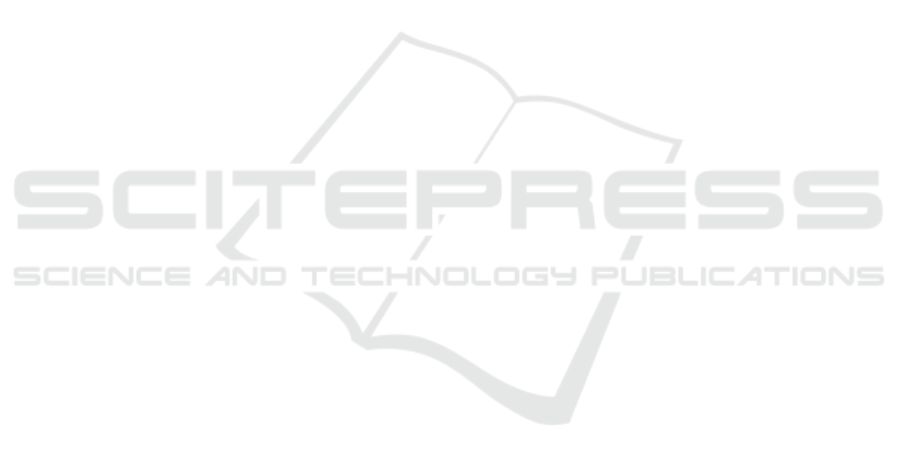
Khan, A., Khan, U., Waleed, M., Khan, A., Kamal, T., Mar-
wat, S. N. K., Maqsood, M., and Aadil, F. (2018).
Remote sensing: an automated methodology for olive
tree detection and counting in satellite images. IEEE
Access, 6:77816–77828.
Koc-San, D., Selim, S., Aslan, N., and San, B. T. (2018).
Automatic citrus tree extraction from uav images and
digital surface models using circular hough transform.
Computers and electronics in agriculture, 150:289–
301.
Li, W., Guo, Q., Jakubowski, M. K., and Kelly, M. (2012).
A new method for segmenting individual trees from
the lidar point cloud. Photogrammetric Engineering
& Remote Sensing, 78(1):75–84.
Long, J., Shelhamer, E., and Darrell, T. (2015). Fully con-
volutional networks for semantic segmentation. In
Proceedings of the IEEE conference on computer vi-
sion and pattern recognition, pages 3431–3440.
Marques, P., P
´
adua, L., Ad
˜
ao, T., Hru
ˇ
ska, J., Peres, E.,
Sousa, A., and Sousa, J. J. (2019). Uav-based auto-
matic detection and monitoring of chestnut trees. Re-
mote Sensing, 11(7):855.
Mubin, N. A., Nadarajoo, E., Shafri, H. Z. M., and Ha-
medianfar, A. (2019). Young and mature oil palm tree
detection and counting using convolutional neural net-
work deep learning method. International Journal of
Remote Sensing, 40(19):7500–7515.
Ocer, N. E., Kaplan, G., Erdem, F., Kucuk Matci, D., and
Avdan, U. (2020). Tree extraction from multi-scale
uav images using mask r-cnn with fpn. Remote sens-
ing letters, 11(9):847–856.
Osco, L. P., De Arruda, M. d. S., Junior, J. M., Da Silva,
N. B., Ramos, A. P. M., Moryia,
´
E. A. S., Imai, N. N.,
Pereira, D. R., Creste, J. E., Matsubara, E. T., et al.
(2020). A convolutional neural network approach for
counting and geolocating citrus-trees in uav multi-
spectral imagery. ISPRS Journal of Photogrammetry
and Remote Sensing, 160:97–106.
Otsu, N. (1979). A threshold selection method from gray-
level histograms. IEEE transactions on systems, man,
and cybernetics, 9(1):62–66.
Panagiotakis, C. and Argyros, A. (2016). Parameter-free
modelling of 2d shapes with ellipses. Pattern Recog-
nition, 53:259–275.
Panagiotakis, C. and Argyros, A. (2020). Region-based
fitting of overlapping ellipses and its application to
cells segmentation. Image and Vision Computing,
93:103810.
Panagiotakis, C. and Argyros, A. A. (2018). Cell segmen-
tation via region-based ellipse fitting. In 2018 25th
IEEE International Conference on Image Processing
(ICIP), pages 2426–2430. IEEE.
Qiu, L., Jing, L., Hu, B., Li, H., and Tang, Y. (2020).
A new individual tree crown delineation method for
high resolution multispectral imagery. Remote Sens-
ing, 12(3):585.
Ren, S., He, K., Girshick, R., and Sun, J. (2015). Faster
r-cnn: Towards real-time object detection with region
proposal networks. Advances in neural information
processing systems, 28.
Rizeei, H. M., Shafri, H. Z., Mohamoud, M. A., Pradhan,
B., and Kalantar, B. (2018). Oil palm counting and age
estimation from worldview-3 imagery and lidar data
using an integrated obia height model and regression
analysis. Journal of Sensors, 2018.
Ronneberger, O., Fischer, P., and Brox, T. (2015). U-net:
Convolutional networks for biomedical image seg-
mentation. In International Conference on Medical
image computing and computer-assisted intervention,
pages 234–241. Springer.
Salam
´
ı, E., Gallardo, A., Skorobogatov, G., and Barrado, C.
(2019). On-the-fly olive tree counting using a uas and
cloud services. Remote Sensing, 11(3):316.
Stefanovi
´
c, B., Stojni
´
c, D., and Danilovi
´
c, M. (2016).
Multi-criteria forest road network planning in fire-
prone environment: a case study in serbia. Jour-
nal of Environmental Planning and Management,
59(5):911–926.
Sun, Y., Li, Z., He, H., Guo, L., Zhang, X., and Xin, Q.
(2022). Counting trees in a subtropical mega city us-
ing the instance segmentation method. International
Journal of Applied Earth Observation and Geoinfor-
mation, 106:102662.
Tang, P., Wang, X., Bai, S., Shen, W., Bai, X., Liu, W.,
and Yuille, A. (2018). Pcl: Proposal cluster learning
for weakly supervised object detection. IEEE trans-
actions on pattern analysis and machine intelligence,
42(1):176–191.
Tong, P., Han, P., Li, S., Li, N., Bu, S., Li, Q., and Li,
K. (2021). Counting trees with point-wise supervised
segmentation network. Engineering Applications of
Artificial Intelligence, 100:104172.
Vibha, L., Shenoy, P. D., Venugopal, K., and Patnaik, L.
(2009). Robust technique for segmentation and count-
ing of trees from remotely sensed data. In 2009 IEEE
International Advance Computing Conference, pages
1437–1442. IEEE.
Wagner, F. H., Ferreira, M. P., Sanchez, A., Hirye, M. C.,
Zortea, M., Gloor, E., Phillips, O. L., de Souza Filho,
C. R., Shimabukuro, Y. E., and Arag
˜
ao, L. E. (2018).
Individual tree crown delineation in a highly diverse
tropical forest using very high resolution satellite im-
ages. ISPRS journal of photogrammetry and remote
sensing, 145:362–377.
Wan, F., Liu, C., Ke, W., Ji, X., Jiao, J., and Ye, Q. (2019).
C-mil: Continuation multiple instance learning for
weakly supervised object detection. In Proceedings
of the IEEE/CVF Conference on Computer Vision and
Pattern Recognition, pages 2199–2208.
Weinstein, B. G., Marconi, S., Aubry-Kientz, M., Vincent,
G., Senyondo, H., and White, E. P. (2020). Deep-
forest: A python package for rgb deep learning tree
crown delineation. Methods in Ecology and Evolu-
tion, 11(12):1743–1751.
Wu, X., Shen, X., Cao, L., Wang, G., and Cao, F. (2019).
Assessment of individual tree detection and canopy
cover estimation using unmanned aerial vehicle based
light detection and ranging (uav-lidar) data in planted
forests. Remote Sensing, 11(8):908.
Yan, W., Guan, H., Cao, L., Yu, Y., Li, C., and Lu, J. (2020).
Unsupervised Tree Detection and Counting via Region-Based Circle Fitting
105