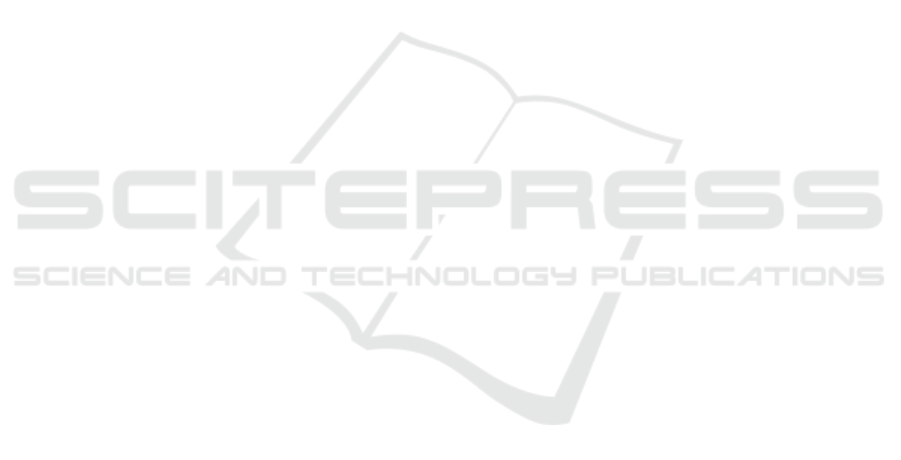
of superconducting qubits. Physical Review Applied,
10(2):024052.
Grover, L. K. (1996). A fast quantum mechanical algorithm
for database search. In Proceedings of the twenty-
eighth annual ACM symposium on Theory of comput-
ing, pages 212–219.
Gupta, A., Roy, P. P., and Dutt, V. (2021). Evaluation
of instance-based learning and q-learning algorithms
in dynamic environments. IEEE Access, 9:138775–
138790.
Harrow, A. W., Hassidim, A., and Lloyd, S. (2009). Quan-
tum algorithm for linear systems of equations. Physi-
cal review letters, 103(15):150502.
Heimann, D., Hohenfeld, H., Wiebe, F., and Kirch-
ner, F. (2022). Quantum deep reinforcement learn-
ing for robot navigation tasks. arXiv preprint
arXiv:2202.12180.
Jerbi, S., Gyurik, C., Marshall, S., Briegel, H., and Dunjko,
V. (2021). Parametrized quantum policies for rein-
forcement learning. Advances in Neural Information
Processing Systems, 34:28362–28375.
Johnson, P. D., Romero, J., Olson, J., Cao, Y., and Aspuru-
Guzik, A. (2017). Qvector: an algorithm for device-
tailored quantum error correction. arXiv preprint
arXiv:1711.02249.
Kandala, A., Mezzacapo, A., Temme, K., Takita, M.,
Brink, M., Chow, J. M., and Gambetta, J. M. (2017).
Hardware-efficient variational quantum eigensolver
for small molecules and quantum magnets. Nature,
549(7671):242–246.
Khadke, A., Agarwal, A., Mohseni-Kabir, A., and Schwab,
D. (2019). Exploration with expert policy advice.
Kullback, S. and Leibler, R. A. (1951). On information
and sufficiency. The annals of mathematical statistics,
22(1):79–86.
Liu, Y., Arunachalam, S., and Temme, K. (2021). A rig-
orous and robust quantum speed-up in supervised ma-
chine learning. Nature Physics, 17(9):1013–1017.
Lockwood, O. and Si, M. (2020). Reinforcement learn-
ing with quantum variational circuit. In Proceedings
of the AAAI Conference on Artificial Intelligence and
Interactive Digital Entertainment, volume 16, pages
245–251.
Meyer, D. A. and Wallach, N. R. (2002). Global entangle-
ment in multiparticle systems. Journal of Mathemati-
cal Physics, 43(9):4273–4278.
Mnih, V., Kavukcuoglu, K., Silver, D., Graves, A.,
Antonoglou, I., Wierstra, D., and Riedmiller, M.
(2013). Playing atari with deep reinforcement learn-
ing. arXiv preprint arXiv:1312.5602.
Mnih, V., Kavukcuoglu, K., Silver, D., Rusu, A. A., Ve-
ness, J., Bellemare, M. G., et al. (2015). Human-level
control through deep reinforcement learning. nature,
518(7540):529–533.
Niraula, D., Jamaluddin, J., Matuszak, M. M., Haken, R.
K. T., and Naqa, I. E. (2021). Quantum deep rein-
forcement learning for clinical decision support in on-
cology: application to adaptive radiotherapy. Scien-
tific reports, 11(1):1–13.
P
´
erez-Salinas, A., Cervera-Lierta, A., Gil-Fuster, E., and
Latorre, J. I. (2020). Data re-uploading for a universal
quantum classifier. Quantum, 4:226.
Popova, M., Isayev, O., and Tropsha, A. (2018). Deep rein-
forcement learning for de novo drug design. Science
advances, 4(7):eaap7885.
Raffin, A., Hill, A., Gleave, A., Kanervisto, A., Ernestus,
M., and Dormann, N. (2021). Stable-baselines3: Reli-
able reinforcement learning implementations. Journal
of Machine Learning Research.
Romero, J., Olson, J. P., and Aspuru-Guzik, A. (2017).
Quantum autoencoders for efficient compression of
quantum data. Quantum Science and Technology,
2(4):045001.
Schuld, M., Bocharov, A., Svore, K. M., and Wiebe, N.
(2020). Circuit-centric quantum classifiers. Physical
Review A, 101(3):032308.
Schulman, J., Wolski, F., Dhariwal, P., Radford, A., and
Klimov, O. (2017). Proximal policy optimization al-
gorithms. arXiv preprint arXiv:1707.06347.
Sim, S., Johnson, P. D., and Aspuru-Guzik, A. (2019).
Expressibility and entangling capability of parame-
terized quantum circuits for hybrid quantum-classical
algorithms. Advanced Quantum Technologies,
2(12):1900070.
Sousa, P. B. and Ramos, R. V. (2006). Universal quantum
circuit for n-qubit quantum gate: A programmable
quantum gate. arXiv preprint quant-ph/0602174.
Steckelmacher, D., Plisnier, H., Roijers, D. M., and Now
´
e,
A. (2019). Sample-efficient model-free reinforce-
ment learning with off-policy critics. In Joint Euro-
pean Conference on Machine Learning and Knowl-
edge Discovery in Databases, pages 19–34. Springer.
Sutton, R. S. and Barto, A. G. (2018). Reinforcement learn-
ing: An introduction. MIT press.
Van Hasselt, H., Guez, A., and Silver, D. (2016). Deep re-
inforcement learning with double q-learning. In Pro-
ceedings of the AAAI conference on artificial intelli-
gence, volume 30.
Wilson, C., Otterbach, J., Tezak, N., Smith, R., Polloreno,
A., Karalekas, P. J., Heidel, S., Alam, M. S., Crooks,
G., and da Silva, M. (2018). Quantum kitchen sinks:
An algorithm for machine learning on near-term quan-
tum computers. arXiv preprint arXiv:1806.08321.
Zhu, Y., Mottaghi, R., Kolve, E., Lim, J. J., Gupta, A., Fei-
Fei, L., and Farhadi, A. (2017). Target-driven visual
navigation in indoor scenes using deep reinforcement
learning. In 2017 IEEE international conference on
robotics and automation (ICRA), pages 3357–3364.
IEEE.
ICAART 2023 - 15th International Conference on Agents and Artificial Intelligence
210