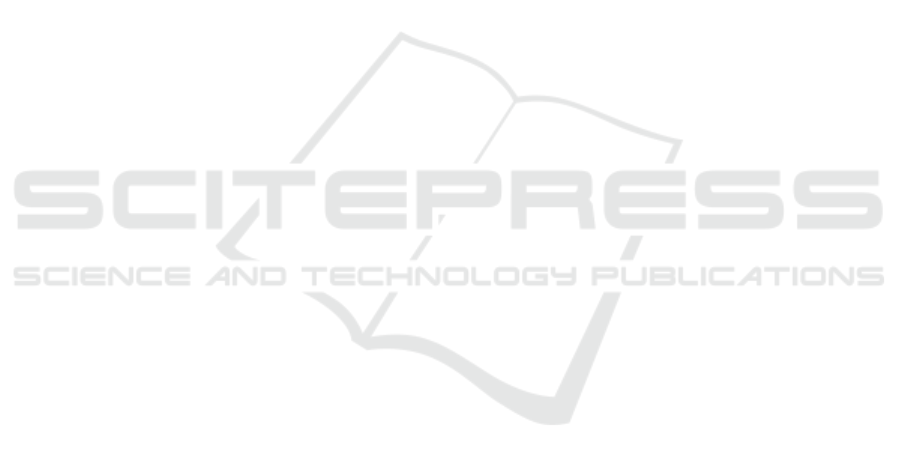
ing that the use of multiple cameras proposed in this
work significantly improved this type of environment.
5 CONCLUSIONS
We proposed a new approach for 3D pedestrian track-
ing in multi-camera environments in this work. Our
method uses the MPNN architecture to associate de-
tections that belong to the same pedestrian and to
trace their spatial-temporal trajectory. By carrying
out experiments on the WILDTRACK database, we
showed that the technique reaches up to 77.1% of
MOTA when trained with the tracking result of Lyra
et al. (2022) and 62.3% of MOTA in 10-fold-cross-
validation. In addition, the time required to track
pedestrians is 40 fps, which is twice the most accu-
rate competing solution (Lyra et al. (2022)).
The results obtained considering only 2 frames are
worse than those obtained with 15 frames because
there are more identity changes, so it would be in-
teresting to study how to reduce these changes so that
the performance using 2 frames is as good as the one
when using 15 frames.
Furthermore, this work evaluated the use of a pos-
sible approach to training the neural network without
the need for ground truth annotations. However, sev-
eral unsupervised training techniques could be tried
in this scenario.
REFERENCES
Badue, C., Guidolini, R., Carneiro, R. V., Azevedo, P., Car-
doso, V. B., Forechi, A., Jesus, L., Berriel, R., Paix
˜
ao,
T. M., Mutz, F., de Paula Veronese, L., Oliveira-
Santos, T., and De Souza, A. F. (2021). Self-driving
cars: A survey. Expert Systems with Applications,
165:113816.
Bergmann, P., Meinhardt, T., and Leal-Taixe, L. (2019).
Tracking without bells and whistles. In Proceedings of
the IEEE/CVF International Conference on Computer
Vision (ICCV).
Bernardin, K. and Stiefelhagen, R. (2008). Evaluating mul-
tiple object tracking performance: the clear mot met-
rics. EURASIP Journal on Image and Video Process-
ing, 2008:1–10.
Bras
´
o, G. and Leal-Taix
´
e, L. (2020). Learning a neural
solver for multiple object tracking. In Proceedings
of the IEEE/CVF Conference on Computer Vision and
Pattern Recognition (CVPR), pages 6247–6257.
Chavdarova, T., Baqu
´
e, P., Bouquet, S., Maksai, A., Jose,
C., Bagautdinov, T., Lettry, L., Fua, P., Van Gool, L.,
and Fleuret, F. (2018). Wildtrack: A multi-camera
hd dataset for dense unscripted pedestrian detection.
In Proceedings of the IEEE Conference on Computer
Vision and Pattern Recognition (CVPR), pages 5030–
5039.
Deng, J., Dong, W., Socher, R., Li, L.-J., Li, K., and Fei-Fei,
L. (2009). Imagenet: A large-scale hierarchical image
database. In 2009 IEEE conference on computer vi-
sion and pattern recognition (CVPR), pages 248–255.
IEEE.
Gan, Y., Han, R., Yin, L., Feng, W., and Wang, S. (2021).
Self-supervised multi-view multi-human association
and tracking. In Proceedings of the 29th ACM Inter-
national Conference on Multimedia, MM ’21, page
282–290, New York, NY, USA. Association for Com-
puting Machinery.
He, K., Zhang, X., Ren, S., and Sun, J. (2016). Deep resid-
ual learning for image recognition. In Proceedings of
the IEEE conference on computer vision and pattern
recognition (CVPR), pages 770–778.
Li, J., Wang, C., Zhu, H., Mao, Y., Fang, H.-S., and Lu,
C. (2019). Crowdpose: Efficient crowded scenes pose
estimation and a new benchmark. In Proceedings of
the IEEE/CVF Conference on Computer Vision and
Pattern Recognition (CVPR), pages 10863–10872.
Lima, J. P., Roberto, R., Figueiredo, L., Simoes, F., and
Teichrieb, V. (2021). Generalizable multi-camera 3d
pedestrian detection. In Proceedings of the IEEE/CVF
Conference on Computer Vision and Pattern Recogni-
tion (CVPR), pages 1232–1240.
Lyra., V., de Andrade., I., Lima., J., Roberto., R.,
Figueiredo., L., Teixeira., J., Thomas., D., Uchiyama.,
H., and Teichrieb., V. (2022). Generalizable online
3d pedestrian tracking with multiple cameras. In Pro-
ceedings of the 17th International Joint Conference
on Computer Vision, Imaging and Computer Graphics
Theory and Applications - Volume 5: VISAPP,, pages
820–827. INSTICC, SciTePress.
Milan, A., Leal-Taix
´
e, L., Reid, I., Roth, S., and Schindler,
K. (2016). Mot16: A benchmark for multi-object
tracking. arXiv preprint arXiv:1603.00831.
Redmon, J. and Farhadi, A. (2018). Yolov3: An incremental
improvement. arXiv.
Sun, Z., Chen, J., Chao, L., Ruan, W., and Mukherjee, M.
(2020). A survey of multiple pedestrian tracking based
on tracking-by-detection framework. IEEE Transac-
tions on Circuits and Systems for Video Technology,
31(5):1819–1833.
Vo, M., Yumer, E., Sunkavalli, K., Hadap, S., Sheikh, Y.,
and Narasimhan, S. G. (2021). Self-supervised multi-
view person association and its applications. IEEE
Transactions on Pattern Analysis and Machine Intel-
ligence, 43(8):2794–2808.
You, Q. and Jiang, H. (2020). Real-time 3d deep multi-
camera tracking. arXiv preprint arXiv:2003.11753.
Zhang, X., Yu, Q., and Yu, H. (2018). Physics inspired
methods for crowd video surveillance and analysis: a
survey. IEEE Access, 6:66816–66830.
Zhou, X., Koltun, V., and Kr
¨
ahenb
¨
uhl, P. (2020). Tracking
objects as points. In Vedaldi, A., Bischof, H., Brox, T.,
and Frahm, J.-M., editors, Computer Vision – ECCV
2020, pages 474–490, Cham. Springer International
Publishing.
Multi-Camera 3D Pedestrian Tracking Using Graph Neural Networks
981