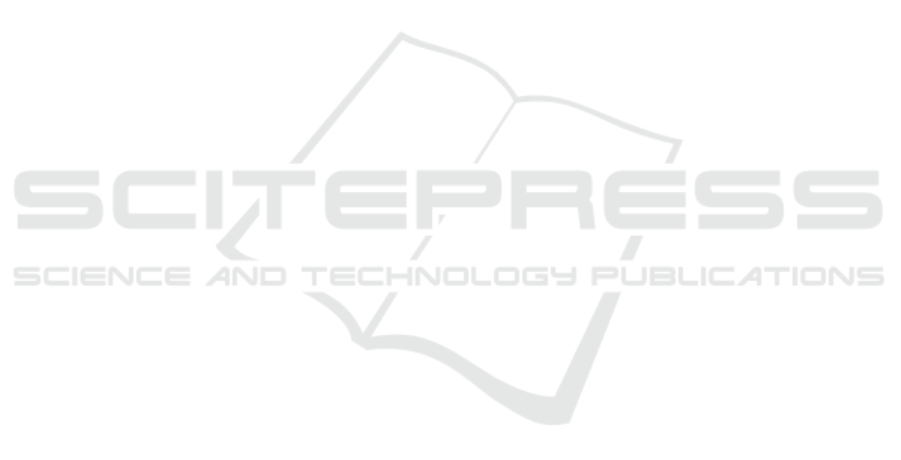
Liu, S., Qi, L., Qin, H., Shi, J., and Jia, J. (2018). Path ag-
gregation network for instance segmentation. In Pro-
ceedings of the IEEE conference on computer vision
and pattern recognition, pages 8759–8768.
Liu, W., Anguelov, D., Erhan, D., Szegedy, C., Reed, S.,
Fu, C.-Y., and Berg, A. C. (2016). Ssd: Single shot
multibox detector. In European conference on com-
puter vision, pages 21–37. Springer.
Liu, Z., Hu, H., Lin, Y., Yao, Z., Xie, Z., Wei, Y., Ning,
J., Cao, Y., Zhang, Z., Dong, L., et al. (2022). Swin
transformer v2: Scaling up capacity and resolution.
In Proceedings of the IEEE/CVF Conference on Com-
puter Vision and Pattern Recognition, pages 12009–
12019.
Liu, Z., Lin, Y., Cao, Y., Hu, H., Wei, Y., Zhang, Z., Lin,
S., and Guo, B. (2021). Swin transformer: Hierarchi-
cal vision transformer using shifted windows. In Pro-
ceedings of the IEEE/CVF International Conference
on Computer Vision, pages 10012–10022.
Peng, C., Xiao, T., Li, Z., Jiang, Y., Zhang, X., Jia, K.,
Yu, G., and Sun, J. (2018). Megdet: A large mini-
batch object detector. In Proceedings of the IEEE con-
ference on Computer Vision and Pattern Recognition,
pages 6181–6189.
Polyak, B. T. and Juditsky, A. B. (1992). Acceleration of
stochastic approximation by averaging. SIAM journal
on control and optimization, 30(4):838–855.
Qiu, X., Sun, T., Xu, Y., Shao, Y., Dai, N., and Huang,
X. (2020). Pre-trained models for natural language
processing: A survey. Science China Technological
Sciences, 63(10):1872–1897.
Radosavovic, I., Kosaraju, R. P., Girshick, R., He, K., and
Doll
´
ar, P. (2020). Designing network design spaces.
In Proceedings of the IEEE/CVF conference on com-
puter vision and pattern recognition, pages 10428–
10436.
Raffel, C., Shazeer, N., Roberts, A., Lee, K., Narang, S.,
Matena, M., Zhou, Y., Li, W., Liu, P. J., et al. (2020).
Exploring the limits of transfer learning with a uni-
fied text-to-text transformer. J. Mach. Learn. Res.,
21(140):1–67.
Redmon, J., Divvala, S., Girshick, R., and Farhadi, A.
(2016). You only look once: Unified, real-time object
detection. In Proceedings of the IEEE conference on
computer vision and pattern recognition, pages 779–
788.
Ren, S., He, K., Girshick, R., and Sun, J. (2015). Faster
r-cnn: Towards real-time object detection with region
proposal networks. Advances in neural information
processing systems, 28.
Ronneberger, O., Fischer, P., and Brox, T. (2015). U-net:
Convolutional networks for biomedical image seg-
mentation. In International Conference on Medical
image computing and computer-assisted intervention,
pages 234–241. Springer.
Russakovsky, O., Deng, J., Su, H., Krause, J., Satheesh, S.,
Ma, S., Huang, Z., Karpathy, A., Khosla, A., Bern-
stein, M., et al. (2015). Imagenet large scale visual
recognition challenge. International journal of com-
puter vision, 115(3):211–252.
Simonyan, K. and Zisserman, A. (2014). Very deep con-
volutional networks for large-scale image recognition.
arXiv preprint arXiv:1409.1556.
Strudel, R., Garcia, R., Laptev, I., and Schmid, C. (2021).
Segmenter: Transformer for semantic segmentation.
In Proceedings of the IEEE/CVF International Con-
ference on Computer Vision, pages 7262–7272.
Sun, P., Zhang, R., Jiang, Y., Kong, T., Xu, C., Zhan,
W., Tomizuka, M., Li, L., Yuan, Z., Wang, C.,
et al. (2021). Sparse r-cnn: End-to-end object detec-
tion with learnable proposals. In Proceedings of the
IEEE/CVF conference on computer vision and pattern
recognition, pages 14454–14463.
Sutskever, I., Vinyals, O., and Le, Q. V. (2014). Sequence
to sequence learning with neural networks. Advances
in neural information processing systems, 27.
Szegedy, C., Liu, W., Jia, Y., Sermanet, P., Reed, S.,
Anguelov, D., Erhan, D., Vanhoucke, V., and Rabi-
novich, A. (2015). Going deeper with convolutions.
In Proceedings of the IEEE conference on computer
vision and pattern recognition, pages 1–9.
Tan, M. and Le, Q. (2019). Efficientnet: Rethinking model
scaling for convolutional neural networks. In Interna-
tional conference on machine learning, pages 6105–
6114. PMLR.
Touvron, H., Cord, M., Douze, M., Massa, F., Sablayrolles,
A., and J
´
egou, H. (2021). Training data-efficient
image transformers & distillation through attention.
In International Conference on Machine Learning,
pages 10347–10357. PMLR.
Vaswani, A., Shazeer, N., Parmar, N., Uszkoreit, J., Jones,
L., Gomez, A. N., Kaiser, Ł., and Polosukhin, I.
(2017). Attention is all you need. Advances in neural
information processing systems, 30.
Wang, W., Xie, E., Li, X., Fan, D.-P., Song, K., Liang, D.,
Lu, T., Luo, P., and Shao, L. (2021). Pyramid vi-
sion transformer: A versatile backbone for dense pre-
diction without convolutions. In Proceedings of the
IEEE/CVF International Conference on Computer Vi-
sion, pages 568–578.
Xie, S., Girshick, R., Doll
´
ar, P., Tu, Z., and He, K. (2017).
Aggregated residual transformations for deep neural
networks. In Proceedings of the IEEE conference on
computer vision and pattern recognition, pages 1492–
1500.
Zhang, P., Dai, X., Yang, J., Xiao, B., Yuan, L., Zhang, L.,
and Gao, J. (2021). Multi-scale vision longformer:
A new vision transformer for high-resolution image
encoding. In Proceedings of the IEEE/CVF Interna-
tional Conference on Computer Vision, pages 2998–
3008.
Zhang, Z., Zhang, X., Peng, C., Xue, X., and Sun, J. (2018).
Exfuse: Enhancing feature fusion for semantic seg-
mentation. In Proceedings of the European conference
on computer vision (ECCV), pages 269–284.
Zhou, B., Zhao, H., Puig, X., Xiao, T., Fidler, S., Barriuso,
A., and Torralba, A. (2019). Semantic understanding
of scenes through the ade20k dataset. International
Journal of Computer Vision, 127(3):302–321.
VISAPP 2023 - 18th International Conference on Computer Vision Theory and Applications
590