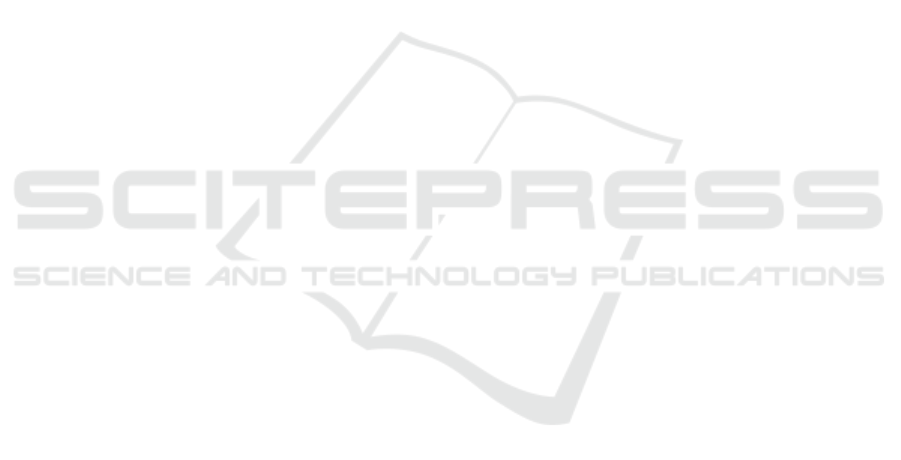
7 CONCLUSIONS
In this study, we proposed a GAN Inversion us-
ing StyleMap, a spatial extension of the latent code
that controls image properties in StyleGAN. We
found that a simple extension of existing encoders to
StyleMap improves reconstruction quality, but signif-
icantly degrades editability. Therefore, we added reg-
ularization to improve editability. Even though the
use of StyleMap is out of consideration in the design
of StyleGAN, we confirmed that our method is com-
parable to existing methods in image editing. In addi-
tion, we showed that StyleMap allows local editing of
arbitrary images. Notably, our method is comparable
in performance to SOTA methods, even though it em-
ploys a strategy independent of PTI. In other words,
performance can be improved by incorporating PTI’s
strategy into our method. In the future, we would like
to adopt a PTI strategy and experiment with a wide
range of data sets.
ACKNOWLEDGEMENTS
This work was supported by JSPS KAKENHI Grant
Numbers JP21H03496, JP22K12157.
REFERENCES
Abdal, R., Qin, Y., and Wonka, P. (2019). Image2stylegan:
How to embed images into the stylegan latent space?
In Proceedings of the IEEE international conference
on computer vision.
Alaluf, Y., Patashnik, O., and Cohen-Or, D. (2021a).
Restyle: A residual-based stylegan encoder via iter-
ative refinement. In Proceedings of the IEEE/CVF In-
ternational Conference on Computer Vision (ICCV).
Alaluf, Y., Tov, O., Mokady, R., Gal, R., and Bermano,
A. H. (2021b). Hyperstyle: Stylegan inversion with
hypernetworks for real image editing.
Deng, J., Guo, J., Niannan, X., and Zafeiriou, S. (2019).
Arcface: Additive angular margin loss for deep face
recognition. In CVPR.
Dinh, T. M., Tran, A. T., Nguyen, R., and Hua, B.-S. (2022).
Hyperinverter: Improving stylegan inversion via hy-
pernetwork. In Proceedings of the IEEE/CVF Con-
ference on Computer Vision and Pattern Recognition
(CVPR).
Goodfellow, I., Pouget-Abadie, J., Mirza, M., Xu, B.,
Warde-Farley, D., Ozair, S., Courville, A., and Ben-
gio, Y. (2014). Generative adversarial nets. In Ghahra-
mani, Z., Welling, M., Cortes, C., Lawrence, N., and
Weinberger, K. Q., editors, Advances in Neural Infor-
mation Processing Systems, volume 27, pages 2672–
2680. Curran Associates, Inc.
Hong, S., Arjovsky, M., Barnhart, D., and Thompson, I.
(2020). Low distortion block-resampling with spa-
tially stochastic networks. In Larochelle, H., Ranzato,
M., Hadsell, R., Balcan, M. F., and Lin, H., editors,
Advances in Neural Information Processing Systems,
volume 33, pages 4441–4452. Curran Associates, Inc.
Isola, P., Zhu, J.-Y., Zhou, T., and Efros, A. A. (2017).
Image-to-image translation with conditional adversar-
ial networks. CVPR.
Karras, T., Aila, T., Laine, S., and Lehtinen, J. (2018). Pro-
gressive growing of gans for improved quality, sta-
bility, and variation. In International Conference on
Learning Representations.
Karras, T., Laine, S., and Aila, T. (2019). A style-based
generator architecture for generative adversarial net-
works. In Proceedings of the IEEE/CVF Conference
on Computer Vision and Pattern Recognition (CVPR).
Karras, T., Laine, S., Aittala, M., Hellsten, J., Lehtinen, J.,
and Aila, T. (2020). Analyzing and improving the im-
age quality of StyleGAN. In Proc. CVPR.
Kim, H., Choi, Y., Kim, J., Yoo, S., and Uh, Y. (2021).
Exploiting spatial dimensions of latent in gan for real-
time image editing. In Proceedings of the IEEE Con-
ference on Computer Vision and Pattern Recognition.
Park, T., Liu, M.-Y., Wang, T.-C., and Zhu, J.-Y. (2019).
Semantic image synthesis with spatially-adaptive nor-
malization. In Proceedings of the IEEE Conference
on Computer Vision and Pattern Recognition.
Pinkney, J. and Adler, D. (2020). Resolution dependant gan
interpolation for controllable image synthesis between
domains.
Richardson, E., Alaluf, Y., Patashnik, O., Nitzan, Y., Azar,
Y., Shapiro, S., and Cohen-Or, D. (2021a). Encoding
in style: a stylegan encoder for image-to-image trans-
lation. In IEEE/CVF Conference on Computer Vision
and Pattern Recognition (CVPR).
Richardson, E., Alaluf, Y., Patashnik, O., Nitzan, Y., Azar,
Y., Shapiro, S., and Cohen-Or, D. (2021b). Encoding
in style: a stylegan encoder for image-to-image trans-
lation. https://github.com/eladrich/pixel2style2pixel#
additional-applications.
Roich, D., Mokady, R., Bermano, A. H., and Cohen-Or, D.
(2021). Pivotal tuning for latent-based editing of real
images. ACM Trans. Graph.
Shen, Y., Gu, J., Tang, X., and Zhou, B. (2020). Interpreting
the latent space of gans for semantic face editing. In
CVPR.
Tov, O., Alaluf, Y., Nitzan, Y., Patashnik, O., and Cohen-Or,
D. (2021). Designing an encoder for stylegan image
manipulation. arXiv preprint arXiv:2102.02766.
Zhang, R., Isola, P., Efros, A. A., Shechtman, E., and Wang,
O. (2018). The unreasonable effectiveness of deep
features as a perceptual metric. In CVPR.
Zhu, J., Shen, Y., Zhao, D., and Zhou, B. (2020a). In-
domain gan inversion for real image editing. In Pro-
ceedings of European Conference on Computer Vision
(ECCV).
Zhu, P., Abdal, R., Qin, Y., Femiani, J., and Wonka, P.
(2020b). Improved stylegan embedding: Where are
the good latents?
ICAART 2023 - 15th International Conference on Agents and Artificial Intelligence
396