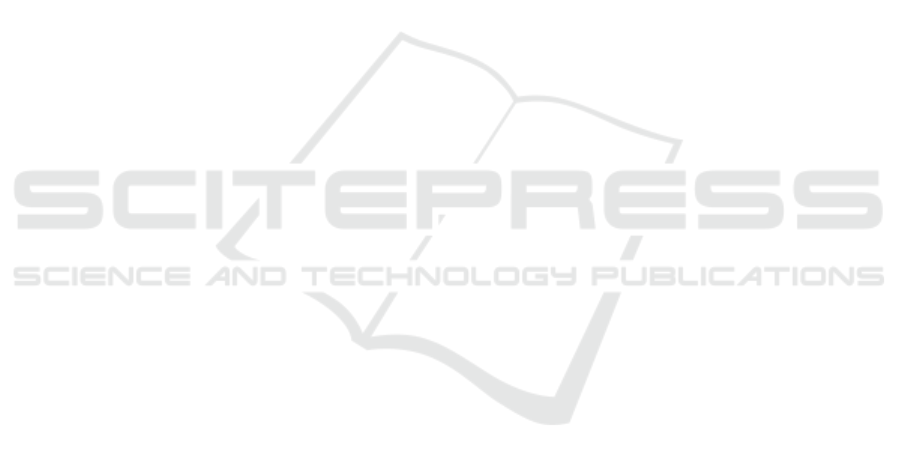
der picking process is collected, allowing for thor-
ough analyses. In this work, an approach for analyz-
ing this data to generate a prediction of order picking
times is presented. The approach is based on explain-
able AI methods that learn the dependencies between
the features of historic picking orders and the time re-
quired. A comparison of LASSO and Decision Tree
Regression discusses the benefits and drawbacks of
the individual approaches. We highlight the poten-
tials this prediction harbors for industrial production:
it enables the identification of features affecting the
order picking time significantly or just marginally, it
allows for optimization of the order picking process
itself, and it enables the optimization of overall pro-
duction as the prediction of order picking time can be
integrated into global optimization approaches.
ACKNOWLEDGEMENTS
This work was funded by research grant “005-2001-
0031” of the MWIDE NRW in form of the Spitzen-
cluster it’s OWL intitiative in the research project
IMAGINE. We thank the Wilo SE for providng the
application case.
REFERENCES
Ahmed, I., Jeon, G., and Piccialli, F. (2022). From artificial
intelligence to explainable artificial intelligence in in-
dustry 4.0: a survey on what, how, and where. IEEE
Transactions on Industrial Informatics, 18(8):5031–
5042.
Balzereit, K., Diedrich, A., Kubus, D., Ginster, J., and
Bunte, A. (2022). Generating causal hypotheses
for explaining black-box industrial processes. 5th
IEEE International Conference on Industrial Cyber-
Physical Systems (ICPS).
Balzereit, K., Maier, A., Barig, B., Hutschenreuther, T., and
Niggemann, O. (2019). Data-driven identification of
causal dependencies in cyber-physical production sys-
tems. In 11th International Conference on Agents and
Artificial Intelligence.
Breiman, L., Friedman, J. H., Olshen, R. A., and Stone,
C. J. (2017). Classification and regression trees. Rout-
ledge.
Burinskiene, A. (2010). Order picking process at ware-
houses. International Journal of Logistics Systems
and Management - Int J Logist Syst Manag, 6.
Burkart, N. and Huber, M. F. (2021). A survey on the ex-
plainability of supervised machine learning. Journal
of Artificial Intelligence Research, 70:245–317.
Do
ˇ
silovi
´
c, F. K., Br
ˇ
ci
´
c, M., and Hlupi
´
c, N. (2018). Ex-
plainable artificial intelligence: A survey. In 2018 41st
International convention on information and commu-
nication technology, electronics and microelectronics
(MIPRO), pages 0210–0215. IEEE.
Ene, S. and
¨
Ozt
¨
urk, N. (2012). Storage location assignment
and order picking optimization in the automotive in-
dustry. The international journal of advanced manu-
facturing technology, 60(5):787–797.
European Factories of the Future Research Association
(2019). EFFRA Vision for a manufacturing partner-
ship in Horizon Europe. 2021-2027.
Grzeszick, R., Lenk, J. M., Rueda, F. M., Fink, G. A., Feld-
horst, S., and Ten Hompel, M. (2017). Deep neural
network based human activity recognition for the or-
der picking process. In Proceedings of the 4th inter-
national Workshop on Sensor-based Activity Recogni-
tion and Interaction, pages 1–6.
Hastie, T., Tibshirani, R., Friedman, J. H., and Friedman,
J. H. (2009). The elements of statistical learning: data
mining, inference, and prediction, volume 2. Springer.
Holzinger, A., Langs, G., Denk, H., Zatloukal, K., and
M
¨
uller, H. (2019). Causability and explainability of
artificial intelligence in medicine. Wiley Interdisci-
plinary Reviews: Data Mining and Knowledge Dis-
covery, 9(4):e1312.
Lu, Y. (2019). Artificial intelligence: a survey on evolu-
tion, models, applications and future trends. Journal
of Management Analytics, 6(1):1–29.
Moeller, K. (2011). Increasing warehouse order picking
performance by sequence optimization. Procedia-
Social and Behavioral Sciences, 20:177–185.
Monostori, L. (2014). Cyber-physical production systems:
Roots, expectations and r&d challenges. Procedia
Cirp, 17:9–13.
Nor, A. K. M., Pedapati, S. R., Muhammad, M., and
Leiva, V. (2021). Overview of explainable artificial
intelligence for prognostic and health management of
industrial assets based on preferred reporting items
for systematic reviews and meta-analyses. Sensors,
21(23):8020.
Panzer, M., Bender, B., and Gronau, N. (2021). Deep re-
inforcement learning in production planning and con-
trol: A systematic literature review. In Conference on
Production Systems and Logistics (CPSL).
SAP. Sequence planning - documentation of sap manufac-
turing exection.
Tierney, K., Balzereit, K., Bunte, A., and Nieh
¨
orster, O.
(2022). Explaining solutions to multi-stage stochas-
tic optimization problems to decision makers. In
2022 IEEE 27th International Conference on Emerg-
ing Technologies and Factory Automation (ETFA),
pages 1–4.
Towill, D. R. (2010). Industrial engineering the toyota pro-
duction system. Journal of Management History.
Utgoff, P. E. (1989). Incremental induction of decision
trees. Machine learning, 4(2):161–186.
World Intellectual Property Organization (2019). WIPO
technology trends. Artificial Intelligence.
Yang, T., Kuo, Y., Su, C.-T., and Hou, C.-L. (2015). Lean
production system design for fishing net manufactur-
ing using lean principles and simulation optimization.
Journal of Manufacturing Systems, 34:66–73.
ICAART 2023 - 15th International Conference on Agents and Artificial Intelligence
412