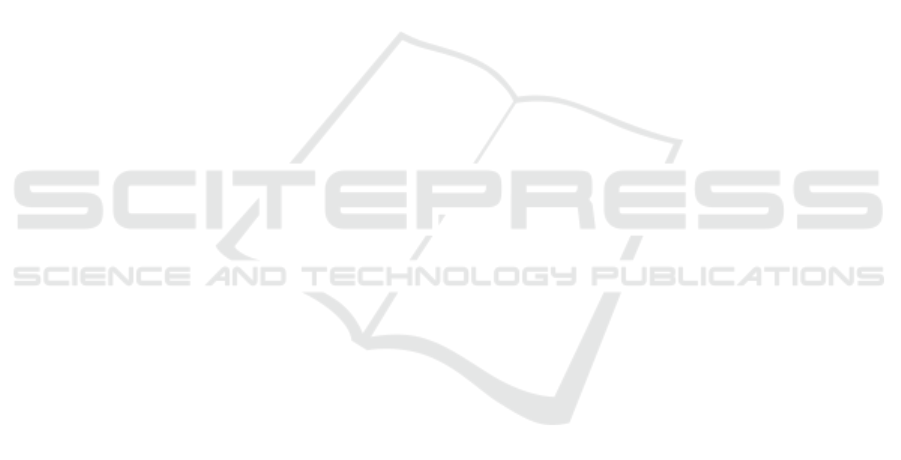
assessment of the severity of cervical dystonia, where
the head posture is not only determined by three
degrees of freedom. Previous head pose estimation
algorithms do not address the necessary complexity
of head poses that are symptoms of cervical dystonia.
By generating a large data set to train the neural
network, we see the possibility to address the lack
of large data sets from real subjects with good
quality annotations regarding head posture. While
the network may have achieved good results on the
synthesized avatar data, this may not necessarily
translate to real-world situations where the input
data may be more varied and complex. Testing the
network on real images will allow us to assess how
well it can handle these variations. However, the
extent to which this generalization can also be applied
to real subject images is a question that we want to
address on the basis of this work in the future.
ACKNOWLEDGEMENTS
We acknowledge financial support by the BMBF
(01ZZ2007). Additionally we acknowledge the AI-
Lab L
¨
ubeck, that has provided us with computational
resources for training the neural networks.
REFERENCES
Albanese, A., Bhatia, K., Bressman, S. B., DeLong, M. R.,
Fahn, S., Fung, V. S. C., Hallett, M., Jankovic, J.,
Jinnah, H. A., Klein, C., Lang, A. E., Mink, J. W., and
Teller, J. K. (2013). Phenomenology and classification
of dystonia: A consensus update. Mov. Disord.,
28(7):863–873.
Ansari, S. A., Nijhawan, R., Bansal, I., and Mohanty, S.
(2021). Cervical dystonia detection using facial and
eye feature. In 2021 10th International Conference on
System Modeling & Advancement in Research Trends
(SMART), pages 43–48.
Boyce, M. J., Canning, C. G., Mahant, N., Morris, J.,
Latimer, J., and Fung, V. S. C. (2012). The toronto
western spasmodic torticollis rating scale: reliability
in neurologists and physiotherapists. 18(5):635–637.
Comella, C. L., Leurgans, S., Wuu, J., Stebbins, G. T.,
Chmura, T., and and The Dystonia Study Group
(2003). Rating scales for dystonia: a multicenter
assessment. Mov. Disord., 18(3):303–312.
Deng, J., Dong, W., Socher, R., Li, L.-J., Li, K., and Fei-Fei,
L. (2009). Imagenet: A large-scale hierarchical image
database. In 2009 IEEE conference on computer
vision and pattern recognition, pages 248–255. Ieee.
Fanelli, G., Dantone, M., Gall, J., Fossati, A., and Van Gool,
L. (2013). Random forests for real time 3d face
analysis. Int. J. Comput. Vision, 101(3):437–458.
Finsterer, J., Maeztu, C., Revuelta, G. J., Reichel, G.,
and Truong, D. (2015). Collum-caput (COL-CAP)
concept for conceptual anterocollis, anterocaput, and
forward sagittal shift. J. Neurol. Sci., 355(1-2):37–43.
Gonzalez Franco, M., Ofek, E., Pan, Y., Antley, A.,
Steed, A., Spanlang, B., Maselli, A., Banakou,
D., Pelechano, N., Orts-Escolano, S., Orvalho, V.,
Trutoiu, L., Wojcik, M., Sanchez-Vives, M. V.,
Bailenson, J., Slater, M., and Lanier, J. (2020).
The rocketbox library and the utility of freely
available rigged avatars. Frontiers in Virtual
Reality. TECHNOLOGY AND CODE ARTICLE
Front. Virtual Real. — frvir.2020.561558.
Hempel, T., Abdelrahman, A. A., and Al-Hamadi, A.
(2022). 6D rotation representation for unconstrained
head pose estimation.
Nakamura, T., Sekimoto, S., Oyama, G., Shimo, Y., Hattori,
N., and Kajimoto, H. (2019). Pilot feasibility study of
a semi-automated three-dimensional scoring system
for cervical dystonia. PLOS ONE, 14:e0219758.
Tan, M. and Le, Q. V. (2019). EfficientNet: Rethinking
model scaling for convolutional neural networks.
Tobin, J., Fong, R., Ray, A., Schneider, J., Zaremba, W.,
and Abbeel, P. (2017). Domain randomization for
transferring deep neural networks from simulation to
the real world.
Valle, R., Buenaposada, J. M., and Baumela, L. (2021).
Multi-Task head pose estimation in-the-wild. IEEE
Trans. Pattern Anal. Mach. Intell., 43(8):2874–2881.
You, Y., Li, J., Reddi, S., Hseu, J., Kumar, S., Bhojanapalli,
S., Song, X., Demmel, J., Keutzer, K., and Hsieh, C.-
J. (2019). Large batch optimization for deep learning:
Training BERT in 76 minutes.
Zhang, Z., Cisneros, E., Lee, H. Y., Vu, J. P., Chen, Q.,
Benadof, C. N., Whitehill, J., Rouzbehani, R., Sy,
D. T., Huang, J. S., Sejnowski, T. J., Jankovic, J.,
Factor, S., Goetz, C. G., Barbano, R. L., Perlmutter,
J. S., Jinnah, H. A., Berman, B. D., Richardson, S. P.,
Stebbins, G. T., Comella, C. L., and Peterson, D. A.
(2022). Hold that pose: capturing cervical dystonia’s
head deviation severity from video. Ann. Clin. Transl.
Neurol., 9(5):684–694.
Zhou, Y. and Gregson, J. (2020). WHENet: Real-time fine-
grained estimation for wide range head pose.
Zhu, X., Lei, Z., Liu, X., Shi, H., and Li, S. Z. (2015). Face
alignment across large poses: A 3D solution.
Extended Head Pose Estimation on Synthesized Avatars for Determining the Severity of Cervical Dystonia
359