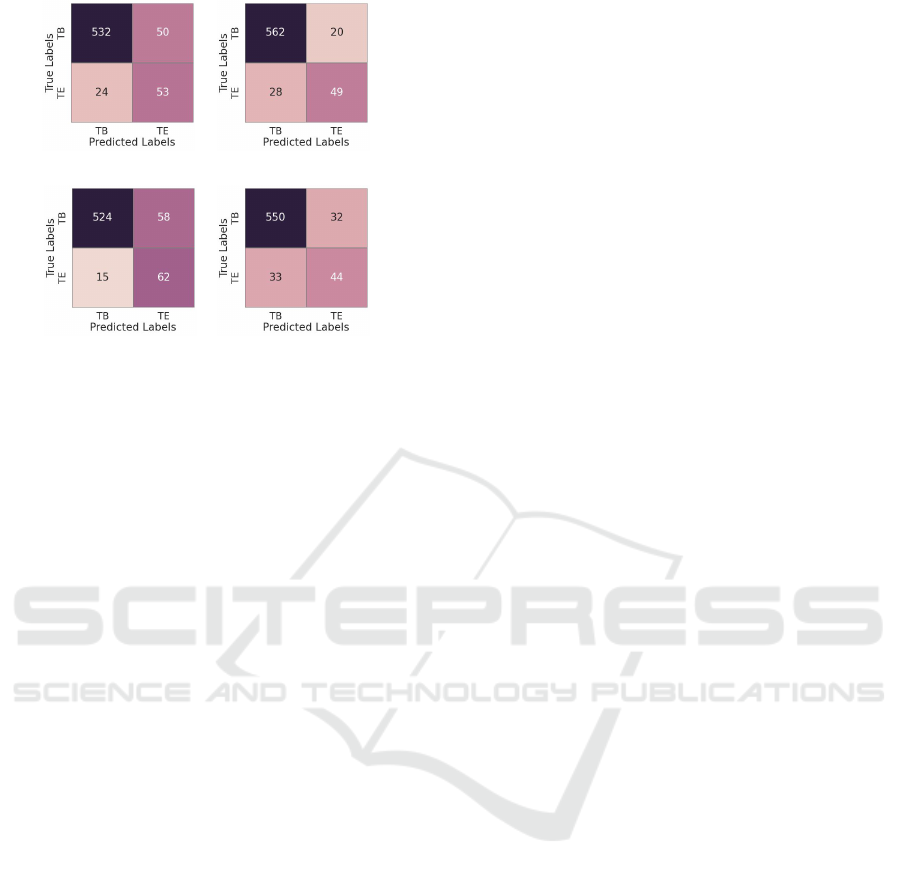
ViT-FL
ViT-MSA-FL
ViT-CE ViT-MSA-CE
Figure 7: Comparative Analysis of the confusion metrics
obtained with the baseline model and with the addition of
the proposed attention module. The experimental values
from the two objective functions experimented with have
also been visually demonstrated, and have been tabulated
in Table 3. The values are on the testing dataset, which
consists of 582 samples belonging to the ‘TB’ class and 77
samples belonging to the ‘TE’ class.
preparation module to extract the ROIs (i.e., the wasp
inidividuals) from the high-resolution images. We
have also obtained SOTA results in the classification
subtask, as compared to other existing methods. This
can be attributed to our classification pipeline, espe-
cially the MSA block, which can extract subtle visual
cues to distinguish between wasp individuals. As evi-
dent from the results reported, this is a robust pipeline
that can be used in other similar wasp detection and
classification problems, or tiny object detection and
classification problems in general.
ACKNOWLEDGEMENTS
This work has been supported by the French government,
through the 3IA C
ˆ
ote d’Azur Investments in the Future
project managed by the National Research Agency (ANR)
with the reference number ANR-19-P3IA-0002. The au-
thors are also grateful to the OPAL infrastructure from Uni-
versit
´
e C
ˆ
ote d’Azur for providing resources and support.
REFERENCES
Aharon, N., Orfaig, R., and Bobrovsky, B.-Z. (2022). Bot-
sort: Robust associations multi-pedestrian tracking.
arXiv preprint arXiv:2206.14651.
Cao, J., Weng, X., Khirodkar, R., Pang, J., and Kitani,
K. (2022). Observation-centric sort: Rethinking
sort for robust multi-object tracking. arXiv preprint
arXiv:2203.14360.
Dat, T., Nguyen, V.-T., and Tran, M.-T. (2018). Lightweight
deep convolutional network for tiny object recogni-
tion. pages 675–682.
Deng, J., Dong, W., Socher, R., Li, L.-J., Li, K., and Fei-
Fei, L. (2009). Imagenet: A large-scale hierarchical
image database. In 2009 IEEE conference on com-
puter vision and pattern recognition, pages 248–255.
Ieee.
Dosovitskiy, A., Beyer, L., Kolesnikov, A., Weissenborn,
D., Zhai, X., Unterthiner, T., Dehghani, M., Minderer,
M., Heigold, G., Gelly, S., et al. (2020). An image is
worth 16x16 words: Transformers for image recogni-
tion at scale. arXiv preprint arXiv:2010.11929.
Du, Y., Song, Y., Yang, B., and Zhao, Y. (2022). Strong-
sort: Make deepsort great again. arXiv preprint
arXiv:2202.13514.
Ge, Z., Liu, S., Wang, F., Li, Z., and Sun, J. (2021).
Yolox: Exceeding yolo series in 2021. arXiv preprint
arXiv:2107.08430.
Gong, Y., Yu, X., Ding, Y., Peng, X., Zhao, J., and Han,
Z. (2021). Effective fusion factor in fpn for tiny ob-
ject detection. In Proceedings of the IEEE/CVF winter
conference on applications of computer vision, pages
1160–1168.
Han, J., Ding, J., Xue, N., and Xia, G.-S. (2021). Redet:
A rotation-equivariant detector for aerial object detec-
tion. In Proceedings of the IEEE/CVF Conference
on Computer Vision and Pattern Recognition, pages
2786–2795.
He, K., Zhang, X., Ren, S., and Sun, J. (2016). Deep resid-
ual learning for image recognition. In Proceedings of
the IEEE conference on computer vision and pattern
recognition, pages 770–778.
Howard, A. G., Zhu, M., Chen, B., Kalenichenko, D.,
Wang, W., Weyand, T., Andreetto, M., and Adam,
H. (2017). Mobilenets: Efficient convolutional neu-
ral networks for mobile vision applications. arXiv
preprint arXiv:1704.04861.
Hu, J., Shen, L., and Sun, G. (2018). Squeeze-and-
excitation networks. In Proceedings of the IEEE con-
ference on computer vision and pattern recognition,
pages 7132–7141.
Kong, F. and Henao, R. (2022). Efficient classification of
very large images with tiny objects. In Proceedings
of the IEEE/CVF Conference on Computer Vision and
Pattern Recognition, pages 2384–2394.
Krizhevsky, A., Sutskever, I., and Hinton, G. E. (2017). Im-
agenet classification with deep convolutional neural
networks. Commun. ACM, 60(6):84–90.
Lee, C., Park, S., Song, H., Ryu, J., Kim, S., Kim, H.,
Pereira, S., and Yoo, D. (2022). Interactive multi-
class tiny-object detection. In Proceedings of the
IEEE/CVF Conference on Computer Vision and Pat-
tern Recognition, pages 14136–14145.
Pani, V., Bernet, M., Calcagno, V., van Oudenhove, L.,
and Bremond, F. F. (2021). TrichTrack: Multi-Object
Tracking of Small-Scale Trichogramma Wasps. In
AVSS 2021 - 17th IEEE International Conference on
Advanced Video and Signal-based Surveillance, Vir-
tual, United States.
TrichANet: An Attentive Network for Trichogramma Classification
871