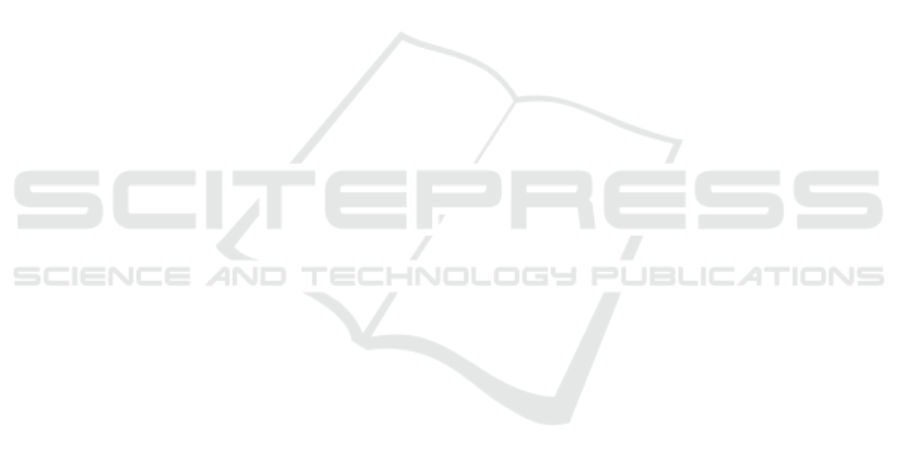
sults is shown in Table 4 for TMC dataset. Features
from KimiaNet were fed to the attention MIL with
clustering, attaining an average test AUC of 0.759.
When SimCLR was used as an feature extractor we
achieved average test AUC of 0.753. These results
show that using feature extractors trained on pathol-
ogy data is not always advantageous, especially if
the feature extractor is trained on a different magni-
fication and for an easier task – KimiaNet is trained
on 20x for organ and histological subtype detection,
while we were performing mutation detection at 40x.
5 CONCLUSION AND
DISCUSSION
We demonstrated our pipeline’s effectiveness for tu-
mor biomarker discovery from WSIs. The tumor
classification model in our pipeline predicts whether
the examined tissue is tumorous or normal with very
high accuracy in line with previous results. Fur-
ther, the histological subtype detection model in our
pipeline can differentiate lung cancer sub-types of
the TCGA dataset with high accuracy as well, again
in line with previous results. Although no morpho-
logical signal is directly visible to a pathologist for
detecting EGFR mutation, we show that appropriate
pipelines with color normalization and weakly super-
vised deep learning models can predict EGFR muta-
tion with an encouraging AUC for both TCGA and
the TMC datasets. For the TCGA dataset, we were
able to outperform previous studies on EGFR detec-
tion (Coudray et al., 2018). Our ablation study found
that KimiaNet pre-trained feature extractor models
do not outperform conventional ResNet50 models
pre-trained on ImageNet. The observation remained
unchanged for the SimCLR-based feature extractor
model as well.
We also performed a few additional experiments.
In one of our experiments, we explicitly filtered out
patches with fewer nuclei from our framework to en-
hance the feature learning in our training scheme.
However, the performance in these cases was worse
compared to our reported results. Further, we also
tried to apply our model, trained on TCGA, on the
TMC dataset and vice versa for EGFR prediction. We
observed substantial performance degradation in both
cases, affirming strong distribution shift between the
datasets. Between the two datasets, we noticed sig-
nificant differences due to variations in tissue prepa-
ration and staining, tar deposits, and cancer stage.
Although we employed color jitter and normaliza-
tion methods to reduce the distribution differences be-
tween the datasets, the performance did not improve
much. In the future, we would like to experiment with
and develop domain adaptation and domain general-
ization techniques to counter this problem.
REFERENCES
American Cancer Society — Information and Resources
about for Cancer: Breast, Colon, Lung, Prostate, Skin.
Anand, D., Ramakrishnan, G., and Sethi, A. (2019). Fast
gpu-enabled color normalization for digital pathology.
In 2019 International Conference on Systems, Sig-
nals and Image Processing (IWSSIP), pages 219–224.
IEEE.
Anand, D., Yashashwi, K., Kumar, N., Rane, S., Gann,
P. H., and Sethi, A. (2021). Weakly supervised learn-
ing on unannotated h&e-stained slides predicts braf
mutation in thyroid cancer with high accuracy. The
Journal of pathology, 255(3):232–242.
Chen, T., Kornblith, S., Norouzi, M., and Hinton, G. (2020).
A simple framework for contrastive learning of visual
representations. In International conference on ma-
chine learning, pages 1597–1607. PMLR.
Coudray, N., Ocampo, P. S., Sakellaropoulos, T., Narula,
N., Snuderl, M., Feny
¨
o, D., Moreira, A. L., Raza-
vian, N., and Tsirigos, A. (2018). Classification and
mutation prediction from non–small cell lung cancer
histopathology images using deep learning. Nature
medicine, 24(10):1559–1567.
Feng, J. and Zhou, Z.-H. (2017). Deep miml network. In
Thirty-First AAAI conference on artificial intelligence.
Hou, L., Samaras, D., Kurc, T. M., Gao, Y., Davis, J. E., and
Saltz, J. H. (2016). Patch-based convolutional neural
network for whole slide tissue image classification. In
Proceedings of the IEEE conference on computer vi-
sion and pattern recognition, pages 2424–2433.
Ilse, M., Tomczak, J., and Welling, M. (2018). Attention-
based deep multiple instance learning. In Interna-
tional conference on machine learning, pages 2127–
2136. PMLR.
Kandemir, M., Haußmann, M., Diego, F., Rajamani, K. T.,
Van Der Laak, J., and Hamprecht, F. A. (2016). Vari-
ational weakly supervised gaussian processes. In
BMVC, pages 71–1.
Lu, M. Y., Williamson, D. F., Chen, T. Y., Chen, R. J., Bar-
bieri, M., and Mahmood, F. (2021). Data-efficient
and weakly supervised computational pathology on
whole-slide images. Nature biomedical engineering,
5(6):555–570.
Maron, O. and Lozano-P
´
erez, T. (1997). A framework for
multiple-instance learning. Advances in neural infor-
mation processing systems, 10.
Pappas, N. and Popescu-Belis, A. (2014). Explaining the
stars: Weighted multiple-instance learning for aspect-
based sentiment analysis. In Proceedings of the 2014
Conference on Empirical Methods In Natural Lan-
guage Processing (EMNLP), pages 455–466.
Pinheiro, P. O. and Collobert, R. (2015). From image-level
to pixel-level labeling with convolutional networks. In
BIOIMAGING 2023 - 10th International Conference on Bioimaging
108