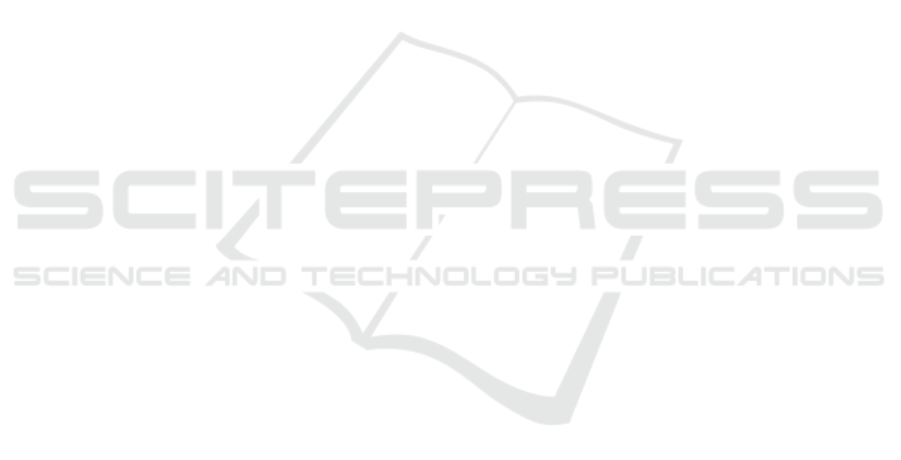
Statistical Methods in Medical Research, 17(6):543–
554.
Boughorbel, S., Jarray, F., and El-Anbari, M. (2017). Op-
timal classifier for imbalanced data using Matthews
Correlation Coefficient metric. PLOS ONE,
12(6):e0177678.
Bousquet, N. (2008). Diagnostics of prior-data agree-
ment in applied bayesian analysis. Journal of Applied
Statistics, 35(9):1011–1029.
Cabitza, F., Campagner, A., and Famiglini, L. (2022).
Global interpretable calibration index, a new metric
to estimate machine learning models’ calibration. In
International Cross-Domain Conference for Machine
Learning and Knowledge Extraction, pages 82–99.
Springer.
Cabitza, F., Campagner, A., and Sconfienza, L. (2020). As
if sand were stone. New concepts and metrics to probe
the ground on which to build trustable AI. BMC Med-
ical Informatics and Decision Making, 20(1).
Cabitza, F., Campagner, A., Soares, F., et al. (2021). The
importance of being external. methodological insights
for the external validation of machine learning mod-
els in medicine. Computer Methods and Programs in
Biomedicine, 208:106288.
Cabitza, F. and Zeitoun, J.-D. (2019). The proof of the pud-
ding: in praise of a culture of real-world validation for
medical artificial intelligence. Annals of translational
medicine, 7(8).
Campagner, A., Sternini, F., and Cabitza, F. (2022). De-
cisions are not all equal. introducing a utility met-
ric based on case-wise raters’ perceptions. Computer
Methods and Programs in Biomedicine, page 106930.
Carrington, A. M., Manuel, D. G., Fieguth, P., et al. (2022).
Deep ROC Analysis and AUC as Balanced Average
Accuracy, for Improved Classifier Selection, Audit
and Explanation. IEEE Transactions on Pattern Anal-
ysis and Machine Intelligence, pages 1–1.
Chicco, D. and Jurman, G. (2020). The advantages of the
Matthews correlation coefficient (MCC) over F1 score
and accuracy in binary classification evaluation. BMC
Genomics, 21(1):6.
Chicco, D., T
¨
otsch, N., and Jurman, G. (2021). The
Matthews correlation coefficient (MCC) is more re-
liable than balanced accuracy, bookmaker informed-
ness, and markedness in two-class confusion matrix
evaluation. BioData Mining, 14(1):13.
Coiera, E. (2016). A new informatics geography. Yearbook
of Medical Informatics, 25(01):251–255.
Friedman, C. P. (2009). A “fundamental theorem” of
biomedical informatics. Journal of the American
Medical Informatics Association, 16(2):169–170.
Hayes, A. F. and Krippendorff, K. (2007). Answering the
call for a standard reliability measure for coding data.
Communication methods and measures, 1(1):77–89.
Hoff, K. A. and Bashir, M. (2015). Trust in automation: In-
tegrating empirical evidence on factors that influence
trust. Human factors, 57(3):407–434.
Holstein, K., Wortman Vaughan, J., Daum
´
e III, H., et al.
(2019). Improving fairness in machine learning sys-
tems: What do industry practitioners need? In Pro-
ceedings of the 2019 CHI conference on human fac-
tors in computing systems, pages 1–16.
Huang, Y., Li, W., Macheret, F., et al. (2020). A tutorial on
calibration measurements and calibration models for
clinical prediction models. Journal of the American
Medical Informatics Association, 27(4):621–633.
Hutson, M. (2018). Artificial intelligence faces repro-
ducibility crisis. Science, 359(6377):725–726.
Kohn, S. C., De Visser, E. J., Wiese, E., et al. (2021). Mea-
surement of trust in automation: A narrative review
and reference guide. Frontiers in Psychology, 12.
Landis, J. R. and Koch, G. G. (1977). The measurement of
observer agreement for categorical data. biometrics,
pages 159–174.
Lee, J. D. and See, K. A. (2004). Trust in automation:
Designing for appropriate reliance. Human factors,
46(1):50–80.
Li, J., Liu, L., Le, T., et al. (2020). Accurate data-driven
prediction does not mean high reproducibility. Nature
Machine Intelligence, 2(1):13–15.
Matthews, B. (1975). Comparison of the predicted and
observed secondary structure of T4 phage lysozyme.
Biochimica et Biophysica Acta (BBA) - Protein Struc-
ture, 405(2):442–451.
Mayer, R. C., Davis, J. H., and Schoorman, F. D. (1995). An
integrative model of organizational trust. Academy of
management review, 20(3):709–734.
McDermott, M. B., Wang, S., Marinsek, N., et al. (2021).
Reproducibility in machine learning for health re-
search: Still a ways to go. Science Translational
Medicine, 13(586):eabb1655.
OECD Network of Experts on AI (2020). Tools for trust-
worthy ai. a framework to compare implementation
tools for trustworthy ai systems. Technical Report
DSTI/CDEP(2020)14/FINAL, OECD.
Rasch, G. (1980). Probabilistic models for some intelli-
gence and attainment tests. 1960. Copenhagen, Den-
mark: Danish Institute for Educational Research.
Riley, R. D., Debray, T. P., Collins, G. S., et al. (2021). Min-
imum sample size for external validation of a clinical
prediction model with a binary outcome. Statistics in
Medicine.
Saal, F. E., Downey, R. G., and Lahey, M. A. (1980). Rat-
ing the ratings: Assessing the psychometric quality of
rating data. Psychological bulletin, 88(2):413.
Tschandl, P., Rinner, C., Apalla, Z., et al. (2020). Human–
computer collaboration for skin cancer recognition.
Nature Medicine, 26(8):1229–1234.
Vickers, A. J., Van Calster, B., and Steyerberg, E. W.
(2016). Net benefit approaches to the evaluation of
prediction models, molecular markers, and diagnostic
tests. bmj, 352.
Youden, W. J. (1950). Index for rating diagnostic tests. Can-
cer, 3(1):32–35.
A Question of Trust: Old and New Metrics for the Reliable Assessment of Trustworthy AI
143