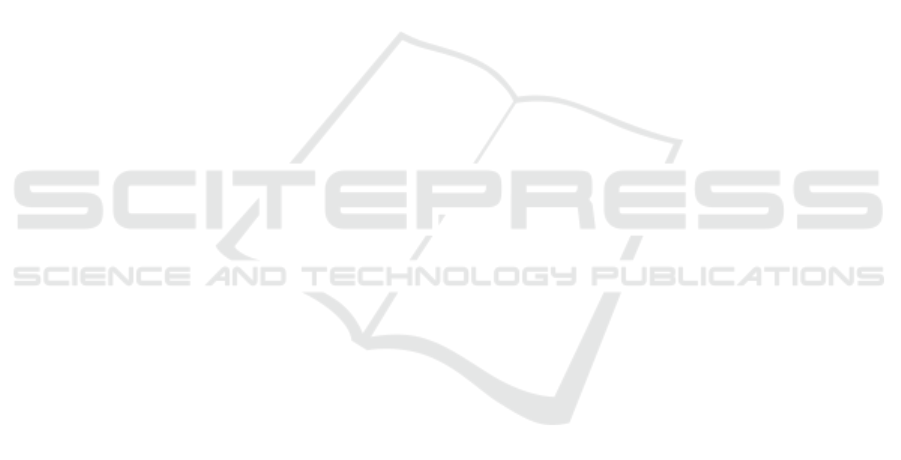
the separation of epistemic and aleatoric uncertainty.
It is also possible to infer the role of uncertainty as a
valuable method under dataset shift conditions and in
strategies such classification with rejection option and
active learning approaches.
Thus, the development of uncertainty aware mod-
els will provide healthcare professionals with access
to the model’s confidence in its predictions but also
refrain the model from delivering classifications with
high uncertainty. Furthermore, samples that have dif-
ferent characteristics and distributions than the ones
learned by the models have higher uncertainty asso-
ciated with their classifications and, therefore, can be
used to retrain the ML models and improve its gen-
eralization and robustness. The active learning ap-
proach is a reliable method for this purpose, demon-
strating that it is a technique capable to self-regulate
the learning of the models in a real life setting, with a
reduction in computational cost as well as in the cost
of labelling the data usually required. Despite the en-
couraging results, much more research is needed in
the area of clinical data uncertainty, particularly in
multi-label data.
To conclude, data with different characteristics
and distributions from those learnt by the ML mod-
els will always exist, so it is imperative that AI sys-
tems possess uncertainty associated methods as safety
mechanisms to produce reliable models to implement
as a decision support system in clinical settings.
REFERENCES
Alday, E. A. P., Gu, A., Shah, A. J., Robichaux, C., Wong,
A.-K. I., Liu, C., Liu, F., Rad, A. B., Elola, A., Seyedi,
S., et al. (2020). Classification of 12-lead ecgs: the
physionet/computing in cardiology challenge 2020.
Physiological measurement, 41(12):124003.
Aseeri, A. O. (2021). Uncertainty-aware deep learning-
based cardiac arrhythmias classification model of
electrocardiogram signals. Computers, 10(6):82.
Barandas, M., Folgado, D., Santos, R., Sim
˜
ao, R., and Gam-
boa, H. (2022). Uncertainty-based rejection in ma-
chine learning: Implications for model development
and interpretability. Electronics, 11(3).
Benjamens, S., Dhunnoo, P., and Mesk
´
o, B. (2020).
The state of artificial intelligence-based fda-approved
medical devices and algorithms: an online database.
NPJ digital medicine, 3(1):1–8.
Chen, M. and Decary, M. (2020). Artificial intelligence
in healthcare: An essential guide for health leaders.
Healthcare Management Forum, 33(1):10–18. PMID:
31550922.
Chen, T.-M., Huang, C.-H., Shih, E. S., Hu, Y.-F., and
Hwang, M.-J. (2020). Detection and classification of
cardiac arrhythmias by a challenge-best deep learning
neural network model. Iscience, 23(3):100886.
Chow, C. (1970). On optimum recognition error and reject
tradeoff. IEEE Transactions on Information Theory,
16(1):41–46.
Depeweg, S., Hernandez-Lobato, J.-M., Doshi-Velez, F.,
and Udluft, S. (2018). Decomposition of uncer-
tainty in Bayesian deep learning for efficient and risk-
sensitive learning. In Dy, J. and Krause, A., editors,
Proceedings of the 35th International Conference on
Machine Learning, volume 80 of Proceedings of Ma-
chine Learning Research, pages 1184–1193. PMLR.
Gal, Y. et al. (2016). Uncertainty in deep learning.
H
¨
ullermeier, E. and Waegeman, W. (2021). Aleatoric and
epistemic uncertainty in machine learning: An intro-
duction to concepts and methods. Machine Learning,
110(3):457–506.
Malinin, A., Band, N., Chesnokov, G., Gal, Y., Gales,
M. J., Noskov, A., Ploskonosov, A., Prokhorenkova,
L., Provilkov, I., Raina, V., et al. (2021). Shifts:
A dataset of real distributional shift across multiple
large-scale tasks. arXiv preprint arXiv:2107.07455.
Mi, L., Wang, H., Tian, Y., and Shavit, N. (2019). Training-
free uncertainty estimation for neural networks.
Nadeem, M. S. A., Zucker, J.-D., and Hanczar, B. (2009).
Accuracy-rejection curves (arcs) for comparing clas-
sification methods with a reject option. In D
ˇ
zeroski,
S., Guerts, P., and Rousu, J., editors, Proceedings of
the third International Workshop on Machine Learn-
ing in Systems Biology, volume 8 of Proceedings of
Machine Learning Research, pages 65–81, Ljubljana,
Slovenia. PMLR.
Settles, B. (2009). Active learning literature survey. Com-
puter Sciences Technical Report 1648, University of
Wisconsin–Madison.
Shaker, M. H. and H
¨
ullermeier, E. (2020). Aleatoric and
epistemic uncertainty with random forests. In Interna-
tional Symposium on Intelligent Data Analysis, pages
444–456. Springer.
St
˚
ahl, N., Falkman, G., Karlsson, A., and Mathiason, G.
(2020). Evaluation of uncertainty quantification in
deep learning. In International Conference on Infor-
mation Processing and Management of Uncertainty in
Knowledge-Based Systems, pages 556–568. Springer.
Vranken, J. F., van de Leur, R. R., Gupta, D. K.,
Juarez Orozco, L. E., Hassink, R. J., van der Harst, P.,
Doevendans, P. A., Gulshad, S., and van Es, R. (2021).
Uncertainty estimation for deep learning-based auto-
mated analysis of 12-lead electrocardiograms. Euro-
pean Heart Journal-Digital Health, 2(3):401–415.
Study of Uncertainty Quantification Using Multi-Label ECG in Deep Learning Models
259