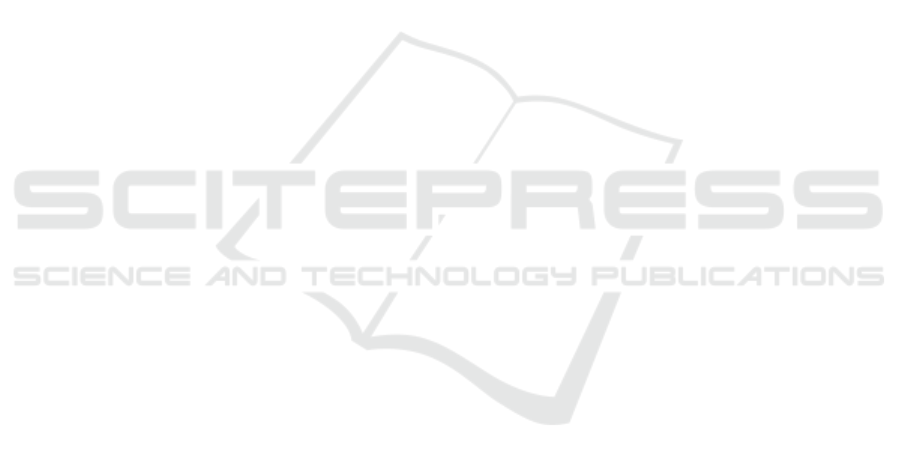
jectives, which were formed with the aid of domain
experts. The results suggested that the proposed inte-
grated MOEA-MLFCM successfully managed to cap-
ture the dynamics behind the decision for migrating to
microservices.
Future research will concentrate on the following:
First, more objectives and scenarios will be investi-
gated so as to form a more complete experimental pic-
ture in terms of factors and inter-dependencies. Sec-
ond, automation of the selection of the most appropri-
ate MOEA will be pursued for each multi-objective
scenario formed in each problem dealt.
REFERENCES
Andreou, A., Mateou, N., and Zombanakis, G. A. (2003).
Evolutionary fuzzy cognitive maps: A hybrid system
for crisis management and political decision making.
Balalaie, A., Heydarnoori, A., and Jamshidi, P. (2016). Mi-
croservices architecture enables devops: Migration to
a cloud-native architecture. IEEE Software, 33(3):42–
52.
Bueno, S. and Salmeron, J. L. (2009). Benchmarking main
activation functions in fuzzy cognitive maps. Expert
Systems with Applications, 36(3):5221–5229.
Chen, Y., Mazlack, L. J., Minai, A. A., and Lu, L. J. (2015).
Inferring causal networks using fuzzy cognitive maps
and evolutionary algorithms with application to gene
regulatory network reconstruction. Applied Soft Com-
puting, 37:667–679.
Christoforou, A. and Andreou, A. S. (2017). A framework
for static and dynamic analysis of multi-layer fuzzy
cognitive maps. Neurocomputing, 232:133–145.
Christoforou, A., Andreou, A. S., Garriga, M., and
Baresi, L. (2022). Adopting microservice architec-
ture: A decision support model based on genetically
evolved multi-layer fcm. Applied Soft Computing,
114:108066.
Deb, K., Pratap, A., Agarwal, S., and Meyarivan, T. (2002).
A fast and elitist multiobjective genetic algorithm:
Nsga-ii. IEEE transactions on evolutionary compu-
tation, 6(2):182–197.
Froelich, W. and Juszczuk, P. (2009). Predictive capabilities
of adaptive and evolutionary fuzzy cognitive maps-a
comparative study. In Intelligent systems for knowl-
edge management, pages 153–174. Springer.
Gunning, D. and Aha, D. (2019). Darpa’s explainable
artificial intelligence (xai) program. AI magazine,
40(2):44–58.
Kosko, B. (1992). Neural networks and fuzzy systems:
a dynamical systems approach to machine intelli-
gence/book and disk. Vol. 1Prentice hall.
Koulouriotis, D., Diakoulakis, I., and Emiris, D. (2001).
Learning fuzzy cognitive maps using evolution strate-
gies: a novel schema for modeling and simulating
high-level behavior. In Proceedings of the 2001
Congress on Evolutionary Computation (IEEE Cat.
No. 01TH8546), volume 1, pages 364–371. IEEE.
Kukkonen, S. and Lampinen, J. (2005). Gde3: The third
evolution step of generalized differential evolution.
In 2005 IEEE congress on evolutionary computation,
volume 1, pages 443–450. IEEE.
Mateou, N., Andreou, A. S., and Stylianou, C. (2008).
A new traversing and execution algorithm for multi-
layered fuzzy cognitive maps. In 2008 IEEE Inter-
national Conference on Fuzzy Systems (IEEE World
Congress on Computational Intelligence), pages
2216–2223. IEEE.
Mateou, N. H. and Andreou, A. S. (2005). Tree-structured
multi-layer fuzzy cognitive maps for modelling large
scale, complex problems. In International Conference
on Intelligent Agents, Web Technologies and Internet
Commerce, volume 2, pages 131–139. IEEE.
Meske, C. and Bunde, E. (2020). Transparency and trust in
human-ai-interaction: The role of model-agnostic ex-
planations in computer vision-based decision support.
In International Conference on Human-Computer In-
teraction, pages 54–69. Springer.
Pedrycz, W. (2010). The design of cognitive maps: A
study in synergy of granular computing and evolution-
ary optimization. Expert systems with applications,
37(10):7288–7294.
Poczeta, K., Kubu
´
s, Ł., and Yastrebov, A. (2019). Analysis
of an evolutionary algorithm for complex fuzzy cog-
nitive map learning based on graph theory metrics and
output concepts. Biosystems, 179:39–47.
Poczeta, K., Yastrebov, A., and Papageorgiou, E. I. (2015).
Learning fuzzy cognitive maps using structure opti-
mization genetic algorithm. In 2015 federated con-
ference on computer science and information systems
(FedCSIS), pages 547–554. IEEE.
Rey, D. and Neuh
¨
auser, M. (2011). Wilcoxon-signed-rank
test.
Sierra, M. R. and Coello Coello, C. A. (2005). Improving
pso-based multi-objective optimization using crowd-
ing, mutation and dominance. In International con-
ference on evolutionary multi-criterion optimization,
pages 505–519. Springer.
Stach, W., Kurgan, L., Pedrycz, W., and Reformat, M.
(2005). Genetic learning of fuzzy cognitive maps.
Fuzzy sets and systems, 153(3):371–401.
Van Veldhuizen, D. A. and Lamont, G. B. (2000). Multiob-
jective evolutionary algorithms: Analyzing the state-
of-the-art. Evolutionary computation, 8(2):125–147.
Wootton, B. (2014). Microservices: a definition of this new
architectural term. Retrieved from:.
Zitzler, E., Laumanns, M., and Thiele, L. (2001). Spea2:
Improving the strength pareto evolutionary algorithm.
TIK-report, 103.
Zitzler, E. and Thiele, L. (1999). Multiobjective evolu-
tionary algorithms: a comparative case study and the
strength pareto approach. IEEE transactions on Evo-
lutionary Computation, 3(4):257–271.
ICAART 2023 - 15th International Conference on Agents and Artificial Intelligence
420