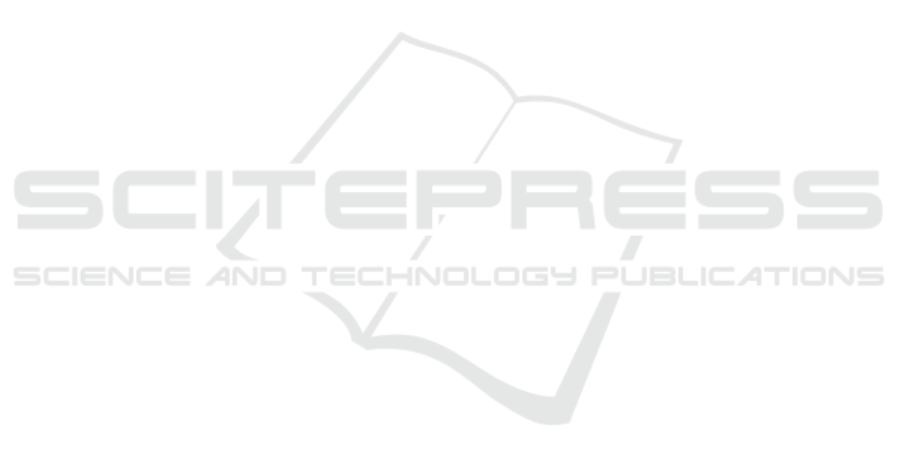
are not related to the ErrP-stimulated response, but to
background EEG. Thus, not only the method seems to
give a good estimate of the sweep of interest, but also
seems appropriate to estimate the background EEG
from the second before the onset of the stimulus.
5 CONCLUSION
In this paper we presented a study on the effect of
applying subspace regularization method to ErrP in
terms of signal processing and of classification met-
rics using a Convolutional Neural Network for distin-
guishing between ErrP and Non–ErrP realizations.
The proposed pre-processing method enhances the
main characteristics of the ErrP signal and improves
the classification performance in each subject and for
each evaluated metric.
Since the subspace regularization method is fast in
terms of computational time, it can be adopted also in
real time BCI classification based on the ErrP Evoked
Potential. Moreover, the proposed method can be ap-
plied also to enhance asynchronous classification of
ErrP events (or in general of Evoked Potentials).
REFERENCES
Abhang, P., Gawali, B., and Mehrotra, S. (2016). Techno-
logical basics of eeg recording and operation of appa-
ratus. Introduction to EEG- and Speech-Based Emo-
tion Recognition.
Belcher, M., I.Hwuang, S.Bhattacharya, Hairston, W., and
Metcalfe, J. (2022). Eeg-based prediction of driv-
ing events from passenger cognitive state using morlet
wavelet and evoked responses. Transportation Engi-
neering, 8:100–107.
BNCI (2020). http://bnci-horizon-2020.eu/database/data-
set.
Chavarriaga, R. and Millan, J. d. R. (2010). Learning
from eeg error-related potentials in noninvasive brain-
computer interfaces. IEEE Transactions on Neural
Systems and Rehabilitation Engineering, 18(4):381–
388.
Falkenstein, M., Hoormann, J., Christ, S., and J.Hohnsbein
(2000). Erp components on reaction errors and their
functional significance: a tutorial. Biological psychol-
ogy, 51(2-3):87–107.
Farabbi, A., Aloia, V., and Mainardi, L. (2021). Arx-based
eeg data balancing for error potential bci. Journal of
Neural ENgineering, 19(3):1741–2552.
Ferrez, W. and del R. Millan, J. (2008). Error-related eeg
potentials generated during simulated brain-computer
interaction. ieee transactions on biomedical engineer-
ing. IEEE transactions on bio-medical engineering,
55.3:923–929.
Gramfort, A., Luessi, M., Larson, E., Engemann, D.,
Strohmeier, D., Brodbeck, C., Goj, R., Jas, M.,
Brooks, T., Parkkonen, L., and H
¨
am
¨
al
¨
ainen, M.
(2013). MEG and EEG data analysis with MNE-
Python. Frontiers in Neuroscience, 7(267):1–13.
Holroyd and M.Coles (2002). The neural basis of human
error processing: Reinforcement learning, dopamine
and the error-related negativity. Psychological Re-
view, pages 679–709.
Karjalainen, P., Kaipio, J., Koistinen, A., and Vauhkonen,
M. (1999). Subspace regularization method for the
single-trial estimation of evoked potentials. IEEE
Transactions on Biomedical Engineering, 46:849–
860.
Lawhern, V. J., Solon, A. J., Waytowich, N. R., Gordon,
S. M., Hung, C. P., and Lance, B. J. (2018). Eegnet: a
compact convolutional neural network for eeg-based
brain-computer interfaces. Journal of Neural Engi-
neering, 15(5).
Lotte, F. (2012). On the need for alternative feedback train-
ing approaches for bci. Berlin Brain-Computer Inter-
face Workshop.
Orrite, C., Rodr
´
ıguez, M., Francisco, Mart
´
ınez, and
Michael Fairhurst, M. (2008). Classifier ensemble
generation for the majority vote rule. Progress in Pat-
tern Recognition, Image Analysis and Applications,
5197:340–347.
Seno, B. D., Matteucci, M., and Mainardi, L. (2010). Online
detection of p300 and error potentials in a bci speller.
Computational intelligence and neuroscience, 2010.
Spuler, M. and Niethammer, C. (2015). Error-related poten-
tials during continuous feedback: using eeg to detect
errors of different type and severity. Frontiers in hu-
man neuroscience, 9:155.
Wolpaw, J., Birbaumer, N., Heetderks, W., D.J.McFarland,
Peckham, P., Schalk, G., Donchin, E., Quatrano,
L., Robinson, C., and Vaughan, T. (2000). Brain-
computer interface technology: a review of the first
international meeting. IEEE transactions on rehabil-
itation engineering : a publication of the IEEE En-
gineering in Medicine and Biology Society, 8(2):164–
173.
Z.Cao (2020). A review of artificial intelligence for
eeg based brain computer interfaces and applications.
Brain Science Advances, 6(3):162–170.
BIOSIGNALS 2023 - 16th International Conference on Bio-inspired Systems and Signal Processing
264