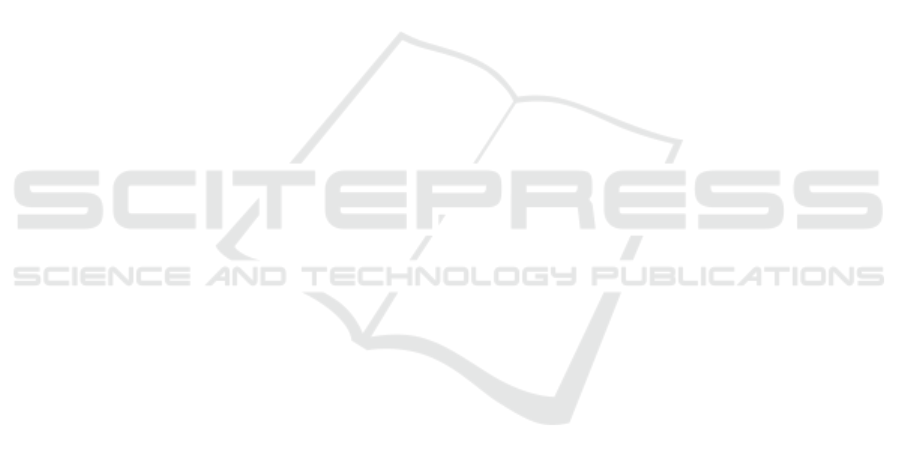
Mant, J., Fitzmaurice, D. A., Hobbs, F. R., Jowett, S., Mur-
ray, E. T., Holder, R., Davies, M., and Lip, G. Y.
(2007). Accuracy of diagnosing atrial fibrillation on
electrocardiogram by primary care practitioners and
interpretative diagnostic software: analysis of data
from screening for atrial fibrillation in the elderly
(safe) trial. Bmj, 335(7616):380.
Mark, R. and Moody, G. (1988). Mit-bih arrhythmia
database directory. Cambridge: Massachusetts Insti-
tute of Technology.
Martis, R. J., Acharya, U. R., Adeli, H., Prasad, H., Tan,
J. H., Chua, K. C., Too, C. L., Yeo, S. W. J., and Tong,
L. (2014). Computer aided diagnosis of atrial arrhyth-
mia using dimensionality reduction methods on trans-
form domain representation. Biomedical signal pro-
cessing and control, 13:295–305.
McAloon, C. J., Boylan, L. M., Hamborg, T., Stallard, N.,
Osman, F., Lim, P. B., and Hayat, S. A. (2016). The
changing face of cardiovascular disease 2000–2012:
An analysis of the world health organisation global
health estimates data. International journal of car-
diology, 224:256–264.
Murray, N. and Perronnin, F. (2014). Generalized max
pooling. In Proceedings of the IEEE conference on
computer vision and pattern recognition, pages 2473–
2480.
Nair, V. and Hinton, G. E. (2010). Rectified linear units
improve restricted boltzmann machines. In Icml.
Nwankpa, C., Ijomah, W., Gachagan, A., and Marshall, S.
(2018). Activation functions: Comparison of trends in
practice and research for deep learning. arXiv preprint
arXiv:1811.03378.
Pollock, K. G., Sekelj, S., Johnston, E., Sandler, B., Hill,
N. R., Ng, F. S., Khan, S., Nassar, A., and Farooqui, U.
(2020). Application of a machine learning algorithm
for detection of atrial fibrillation in secondary care.
IJC Heart & Vasculature, 31:100674.
S
´
anchez, F. R. and Cervera, J. G. (2019). ECG classification
using artificial neural networks. In Journal of Physics:
Conference Series, volume 1221, page 012062. IOP
Publishing.
Schwab, P., Scebba, G. C., Zhang, J., Delai, M., and Karlen,
W. (2017). Beat by beat: Classifying cardiac arrhyth-
mias with recurrent neural networks. In 2017 Com-
puting in Cardiology (CinC), pages 1–4. IEEE.
Sellami, A. and Hwang, H. (2019). A robust deep convo-
lutional neural network with batch-weighted loss for
heartbeat classification. Expert Systems with Applica-
tions, 122:75–84.
Shi, H., Wang, H., Zhang, F., Huang, Y., Zhao, L., and Liu,
C. (2019). Inter-patient heartbeat classification based
on region feature extraction and ensemble classifier.
Biomedical Signal Processing and Control, 51:97–
105.
Shoemaker, M. B., Shah, R. L., Roden, D. M., and Perez,
M. V. (2020). How will genetics inform the clini-
cal care of atrial fibrillation? Circulation research,
127(1):111–127.
Srivastava, N., Hinton, G., Krizhevsky, A., Sutskever, I.,
and Salakhutdinov, R. (2014). Dropout: a simple way
to prevent neural networks from overfitting. The jour-
nal of machine learning research, 15(1):1929–1958.
Uchida, K., Tanaka, M., and Okutomi, M. (2018). Cou-
pled convolution layer for convolutional neural net-
work. Neural Networks, 105:197–205.
Xiang, Y., Luo, J., Zhu, T., Wang, S., Xiang, X., and Meng,
J. (2018). ECG-based heartbeat classification using
two-level convolutional neural network and rr interval
difference. IEICE TRANSACTIONS on Information
and Systems, 101(4):1189–1198.
Xie, Q., Wang, X., Sun, H., Zhang, Y., and Lu, X.
(2021). ECG signal detection and classification of
heart rhythm diseases based on ResNet and LSTM.
Advances in Mathematical Physics, 2021.
Xiong, Z., Stiles, M. K., and Zhao, J. (2017). Robust ecg
signal classification for detection of atrial fibrillation
using a novel neural network. In 2017 Computing in
Cardiology (CinC), pages 1–4. IEEE.
Xu, Q., Zhang, M., Gu, Z., and Pan, G. (2019). Over-
fitting remedy by sparsifying regularization on fully-
connected layers of cnns. Neurocomputing, 328:69–
74.
Xu, X. and Liu, H. (2020). ECG heartbeat classification
using convolutional neural networks. IEEE Access,
8:8614–8619.
Zhai, X. and Tin, C. (2018). Automated ECG classification
using dual heartbeat coupling based on convolutional
neural network. IEEE Access, 6:27465–27472.
Zhiqiang, W. and Jun, L. (2017). A review of object de-
tection based on convolutional neural network. In
2017 36th Chinese control conference (CCC), pages
11104–11109. IEEE.
Zhu, H., Cheng, C., Yin, H., Li, X., Zuo, P., Ding, J.,
Lin, F., Wang, J., Zhou, B., Li, Y., et al. (2020).
Automatic multilabel electrocardiogram diagnosis of
heart rhythm or conduction abnormalities with deep
learning: a cohort study. The Lancet Digital Health,
2(7):e348–e357.
ICPRAM 2023 - 12th International Conference on Pattern Recognition Applications and Methods
362