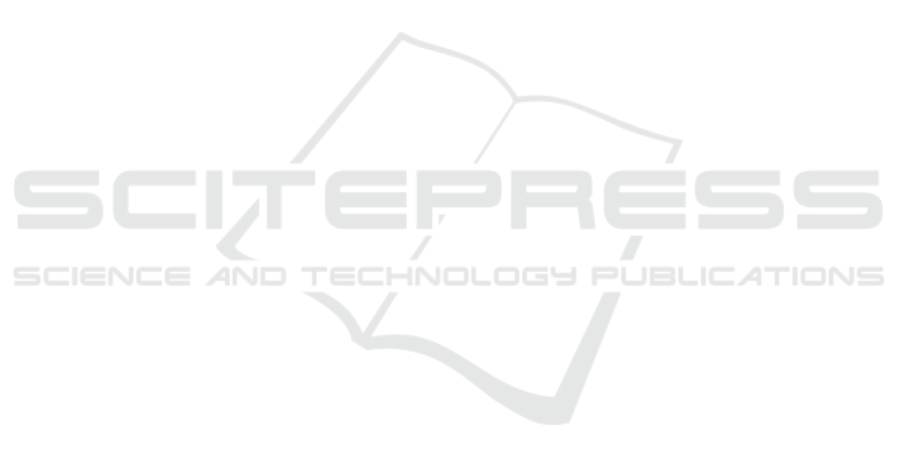
other hand, the deep learning-based approach for im-
age classification used MobileNetV2 as a base model
for the overall structure and a different feature space
resulting in a facial mesh. Looking at our results,
we achieved an accuracy of approximately 98.93%,
which shows that the model outperformed all the pre-
vious studies mentioned in the article and our ini-
tial approach. Developing such incremental and im-
proved methods results in higher reliability and accu-
racy in medical diagnostic systems. These methods
can also serve as the basis for forming standardised
tools for medical assessments, treatment, and moni-
toring.
REFERENCES
Barbosa, J., Seo, W.-K., and Kang, J. (2019). parafacetest:
an ensemble of regression tree-based facial features
extraction for efficient facial paralysis classification.
BMC Medical Imaging, 19(1):1–14.
Boyko, N., Basystiuk, O., and Shakhovska, N. (2018). Per-
formance evaluation and comparison of software for
face recognition, based on dlib and opencv library. In
2018 IEEE Second International Conference on Data
Stream Mining & Processing (DSMP), pages 478–
482. IEEE.
Davis, J. and Goadrich, M. (2006). The relationship be-
tween precision-recall and roc curves. In Proceed-
ings of the 23rd international conference on Machine
learning, pages 233–240.
Grishchenko, I., Ablavatski, A., Kartynnik, Y., Raveen-
dran, K., and Grundmann, M. (2020). Attention mesh:
High-fidelity face mesh prediction in real-time. arXiv
preprint arXiv:2006.10962.
Guarin, D. L., Dusseldorp, J., Hadlock, T. A., and Jowett, N.
(2018). A machine learning approach for automated
facial measurements in facial palsy. JAMA facial plas-
tic surgery, 20(4):335–337.
Hassaballah, M. and Hosny, K. M. (2019). Recent advances
in computer vision. Studies in computational intelli-
gence, 804:1–84.
Hato, N., Matsumoto, S., Kisaki, H., Takahashi, H., Wak-
isaka, H., Honda, N., Gyo, K., Murakami, S., and
Yanagihara, N. (2003). Efficacy of early treatment
of bell’s palsy with oral acyclovir and prednisolone.
Otology & neurotology, 24(6):948–951.
Jiang, C., Wu, J., Zhong, W., Wei, M., Tong, J., Yu, H.,
and Wang, L. (2020). Automatic facial paralysis as-
sessment via computational image analysis. Journal
of Healthcare Engineering, 2020.
Jison Hsu, G.-S., Huang, W.-F., and Kang, J.-H. (2018). Hi-
erarchical network for facial palsy detection. In Pro-
ceedings of the IEEE Conference on Computer Vision
and Pattern Recognition Workshops, pages 580–586.
Khan, M., Chakraborty, S., Astya, R., and Khepra, S.
(2019). Face detection and recognition using opencv.
In 2019 International Conference on Computing,
Communication, and Intelligent Systems (ICCCIS),
pages 116–119. IEEE.
Kim, H. S., Kim, S. Y., Kim, Y. H., and Park, K. S. (2015).
A smartphone-based automatic diagnosis system for
facial nerve palsy. Sensors, 15(10):26756–26768.
Panetta, K., Wan, Q., Agaian, S., Rajeev, S., Kamath, S.,
Rajendran, R., Rao, S. P., Kaszowska, A., Taylor,
H. A., Samani, A., et al. (2018). A comprehensive
database for benchmarking imaging systems. IEEE
transactions on pattern analysis and machine intelli-
gence, 42(3):509–520.
Parra-Dominguez, G. S., Sanchez-Yanez, R. E., and Garcia-
Capulin, C. H. (2021). Facial paralysis detection on
images using key point analysis. Applied Sciences,
11(5):2435.
Sandler, M., Howard, A., Zhu, M., Zhmoginov, A., and
Chen, L.-C. (2018). Mobilenetv2: Inverted residu-
als and linear bottlenecks. In Proceedings of the IEEE
conference on computer vision and pattern recogni-
tion, pages 4510–4520.
Tiemstra, J. D. and Khatkhate, N. (2007). Bell’s palsy: di-
agnosis and management. American family physician,
76(7):997–1002.
Wang, T., Zhang, S., Dong, J., Liu, L., and Yu, H.
(2016). Automatic evaluation of the degree of facial
nerve paralysis. Multimedia Tools and Applications,
75(19):11893–11908.
Wu, Y., Hassner, T., Kim, K., Medioni, G., and Natarajan, P.
(2017). Facial landmark detection with tweaked con-
volutional neural networks. IEEE transactions on pat-
tern analysis and machine intelligence, 40(12):3067–
3074.
ICPRAM 2023 - 12th International Conference on Pattern Recognition Applications and Methods
888