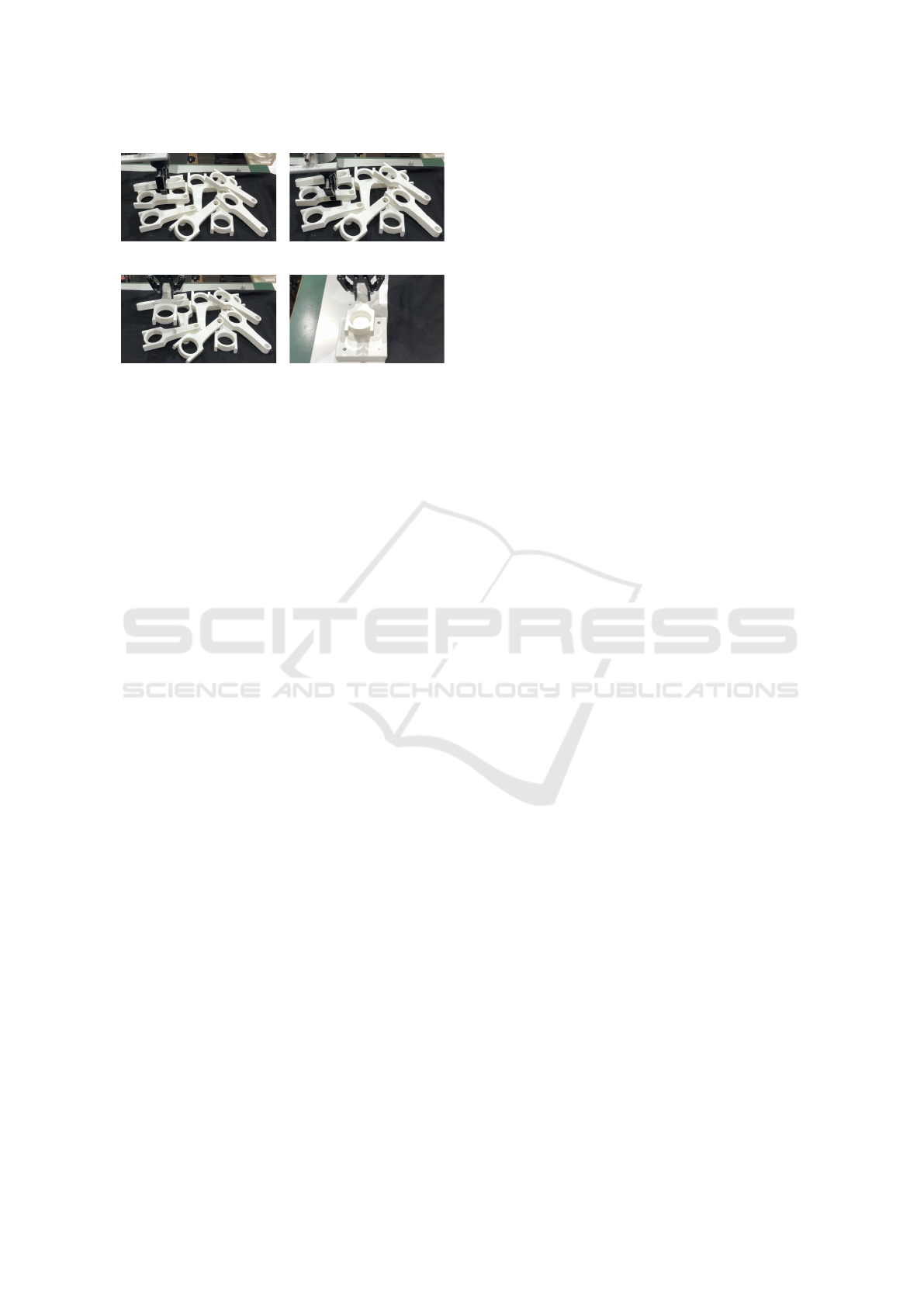
(a) Approach part
(b) Grasp
(c) Pick up
(d) Insert
Figure 9: State of object assembled by robot. (a) Robot
approaches the grasping point estimated by the proposed
method. (b) The part is grasped by the robot. (c) Robot
pick up the part. (d) The robot inserts the carried parts into
the shaft.
a “function” such as “to be grasped” or “to be assem-
bled with other parts” for each region. We defined a
novel idea of functional labels and their consistency in
industrial parts. Functional consistency is used in the
proposed method as a cue, robot motion parameters
are estimated on the basis of relationship between pa-
rameters and functions. In an experiment using con-
necting rods, the average success rate was 81.5%. The
effectiveness of the proposed method was confirmed
from the ablation studies and comparison with related
work. The proposed method has a higher success rate
than methods that do not use function and functional
consistency, these are especially important concepts.
In future work, we will propose a method for estimat-
ing grasping points for parts that are easy to assemble
from a bin scene.
ACKNOWLEDGEMENTS
This paper is based on results obtained from a
project, JPNP20006, commissioned by the New En-
ergy and Industrial Technology Development Organi-
zation (NEDO).
REFERENCES
Akizuki, S. and Hashimoto, M. (2020). Detection of seman-
tic grasping-parameter using part-affordance recogni-
tion. In In International Joint Conference on Com-
puter Vision, Imaging and Computer Graphics Theory
and Applications(VISAPP)., pages 470–475.
Araki, R., Hasegawa, T., Yamauchi, Y., Yamashita, T., Fu-
jiyoshi, H., Domae, Y., Kawanishi, R., and Seki, M.
(2018). Grasping detection using deep convolutional
neural network with graspability. In Journal of the
Robotics Society of Japan, volume 36, pages 559–566.
Ardon, P., Pairet, E., Petrick, R., Ramamoorthy, S., and Lo-
han, K. (2020). Self-assessment of grasp affordance
transfer. In Proceedings of IEEE/RSJ International
Conference on Intelligent Robots and Systems (IROS),
pages 9385–9392.
Chu, F.-J., Xu, R., and Vela, P. (2019a). Learning affordance
segmentation for real-world robotic manipulation via
synthetic images. In IEEE Robotics and Automation
Letters, volume 4, pages 1140–1147.
Chu, F.-J., Xu, R., and Vela, P. (2019b). Toward affordance
detection and ranking on novel objects for real-world
robotic manipulation. In IEEE Robotics and Automa-
tion Letters, volume 4, pages 4070–4077.
Domae, Y., Okuda, H., Taguchi, Y., Sumi, K., and Hi-
rai, T. (2014). Fast graspability evaluation on sin-
gle depth maps for bin picking with general grippers.
In Proceedings of IEEE International Conference on
Robotics and Automation (ICRA), pages 1997–2004.
Hamalainen, A., Arndt, K., Ghadirzadeh, A., and Kyrki,
V. (2019). Affordance learning for end-to-end visuo-
motor robot control. In Proceedings of IEEE/RSJ In-
ternational Conference on Intelligent Robots and Sys-
tems (IROS), pages 1781–1788.
He, K., Gkioxari, G., Dollar, P., and Girshick, R. (2017).
Mask r-cnn. In Proceedings of the IEEE international
conference on computer vision, pages 2961–2969.
Iizuka, M. and Hashimoto, M. (2018). Detection of seman-
tic grasping-parameter using part-affordance recog-
nition. In Proceedings of International Conference
on Research and Education in Mechatronics (REM),
pages 136–140.
Kokic, M., Stork, J., Haustein, J., and Kragic, D. (2017).
Affordance detection for task-specific grasping using
deep learning. In Proceedings of IEEE-RAS Interna-
tional Conference on Humanoid Robotics, pages 91–
98.
Liu, W., Anguelov, D., Erhan, D., Szegedy, C., Reed, S., Fu,
C., and Berg, A. (2016). Ssd: Single shot multibox
detector. In Proceedings of European conference on
computer vision (ECCV), pages 21–37.
Liu, W., Daruna, A., and Chernova, S. (2020). Cage:
Context-aware grasping engine. In Proceedings of
IEEE International Conference on Robotics and Au-
tomation (ICRA), pages 2550–2556.
Minh, C., Gilani, S., Islam, S., and Suter, D. (2020). Learn-
ing affordance segmentation: An investigative study.
In Proceedings of International Conference on Dig-
ital Image Computing: Techniques and Applications
(DICTA), pages 2870–2877.
Myers, A., Teo, C., Ferm
¨
uller, C., and Aloimonos, Y.
(2015). Affordance detection of tool parts from
geometric features. In Proceedings of IEEE In-
ternational Conference on Robotics and Automation
(ICRA), pages 1374–1381.
Qin, Z., Fang, K., Zhu, Y., Fei-Fei, L., and Savarese,
S. (2020). Keto:learning keypoint representations
for tool manipulation. In Proceedings of IEEE In-
Estimation of Robot Motion Parameters Based on Functional Consistency for Randomly Stacked Parts
527