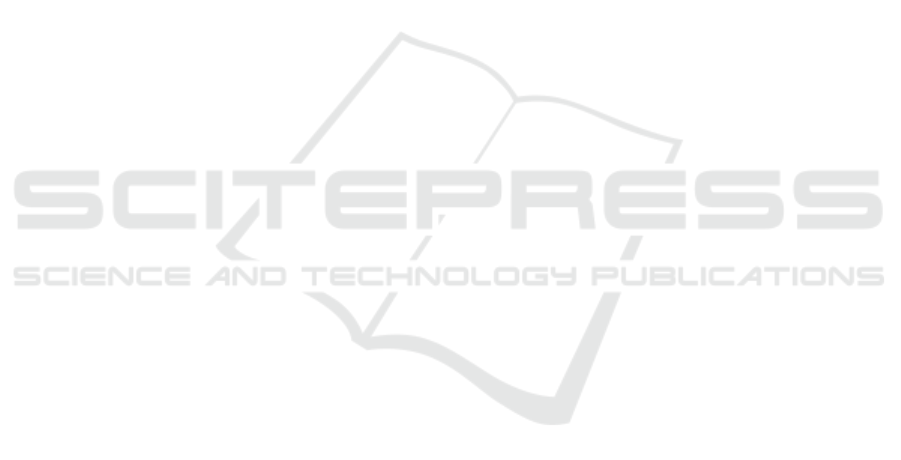
ference on computer vision and pattern recognition,
pages 7132–7141.
Huang, G., Liu, Z., Van Der Maaten, L., and Weinberger,
K. Q. (2017). Densely connected convolutional net-
works. In Proceedings of the IEEE conference on
computer vision and pattern recognition, pages 4700–
4708.
Huang, S.-C., Cheng, F.-C., and Chiu, Y.-S. (2012). Ef-
ficient contrast enhancement using adaptive gamma
correction with weighting distribution. IEEE trans-
actions on image processing, 22(3):1032–1041.
Kaul, C., Manandhar, S., and Pears, N. (2019). Focus-
net: An attention-based fully convolutional network
for medical image segmentation. In 2019 IEEE 16th
international symposium on biomedical imaging (ISBI
2019), pages 455–458. IEEE.
Litjens, G., Kooi, T., Bejnordi, B. E., Setio, A. A. A.,
Ciompi, F., Ghafoorian, M., Van Der Laak, J. A.,
Van Ginneken, B., and S
´
anchez, C. I. (2017). A survey
on deep learning in medical image analysis. Medical
image analysis, 42:60–88.
Long, J., Shelhamer, E., and Darrell, T. (2015). Fully con-
volutional networks for semantic segmentation. In
Proceedings of the IEEE conference on computer vi-
sion and pattern recognition, pages 3431–3440.
Mnih, V., Heess, N., Graves, A., et al. (2014). Recurrent
models of visual attention. Advances in neural infor-
mation processing systems, 27.
Nachbar, F., Stolz, W., Merkle, T., Cognetta, A. B., Vogt,
T., Landthaler, M., Bilek, P., Braun-Falco, O., and
Plewig, G. (1994). The abcd rule of dermatoscopy:
high prospective value in the diagnosis of doubtful
melanocytic skin lesions. Journal of the American
Academy of Dermatology, 30(4):551–559.
Oktay, O., Schlemper, J., Folgoc, L. L., Lee, M., Heinrich,
M., Misawa, K., Mori, K., McDonagh, S., Hammerla,
N. Y., Kainz, B., et al. (2018). Attention u-net: Learn-
ing where to look for the pancreas. arXiv preprint
arXiv:1804.03999.
Organization, W. H. et al. (2017). Radiation: Ultraviolet
(uv) radiation and skin cancer. Published October, 16.
Pereira, S., Pinto, A., Alves, V., and Silva, C. A. (2016).
Brain tumor segmentation using convolutional neural
networks in mri images. IEEE transactions on medi-
cal imaging, 35(5):1240–1251.
Powers, D. M. (2020). Evaluation: from precision, recall
and f-measure to roc, informedness, markedness and
correlation. arXiv preprint arXiv:2010.16061.
Ronneberger, O., Fischer, P., and Brox, T. (2015). U-net:
Convolutional networks for biomedical image seg-
mentation. In International Conference on Medical
image computing and computer-assisted intervention,
pages 234–241. Springer.
Tschandl, P., Rosendahl, C., and Kittler, H. (2018). The
ham10000 dataset, a large collection of multi-source
dermatoscopic images of common pigmented skin le-
sions. Scientific data, 5(1):1–9.
Vesal, S., Ravikumar, N., and Maier, A. (2018). Skin-
net: A deep learning framework for skin lesion seg-
mentation. In 2018 IEEE Nuclear Science Sympo-
sium and Medical Imaging Conference Proceedings
(NSS/MIC), pages 1–3. IEEE.
Wang, X., Girshick, R., Gupta, A., and He, K. (2018). Non-
local neural networks. In Proceedings of the IEEE
conference on computer vision and pattern recogni-
tion, pages 7794–7803.
Wibowo, A., Purnama, S. R., Wirawan, P. W., and Rasyidi,
H. (2021). Lightweight encoder-decoder model for
automatic skin lesion segmentation. Informatics in
Medicine Unlocked, 25:100640.
Woo, S., Park, J., Lee, J.-Y., and Kweon, I. S. (2018). Cbam:
Convolutional block attention module. In Proceed-
ings of the European conference on computer vision
(ECCV), pages 3–19.
Xie, S., Girshick, R., Doll
´
ar, P., Tu, Z., and He, K. (2017).
Aggregated residual transformations for deep neural
networks. In Proceedings of the IEEE conference on
computer vision and pattern recognition, pages 1492–
1500.
Yuan, Y. and Lo, Y.-C. (2017). Improving dermoscopic
image segmentation with enhanced convolutional-
deconvolutional networks. IEEE journal of biomed-
ical and health informatics, 23(2):519–526.
Zafar, K., Gilani, S. O., Waris, A., Ahmed, A., Jamil, M.,
Khan, M. N., and Sohail Kashif, A. (2020). Skin
lesion segmentation from dermoscopic images using
convolutional neural network. Sensors, 20(6):1601.
Zhou, Z., Siddiquee, M. M. R., Tajbakhsh, N., and Liang, J.
(2019). Unet++: Redesigning skip connections to ex-
ploit multiscale features in image segmentation. IEEE
transactions on medical imaging, 39(6):1856–1867.
BIOINFORMATICS 2023 - 14th International Conference on Bioinformatics Models, Methods and Algorithms
100