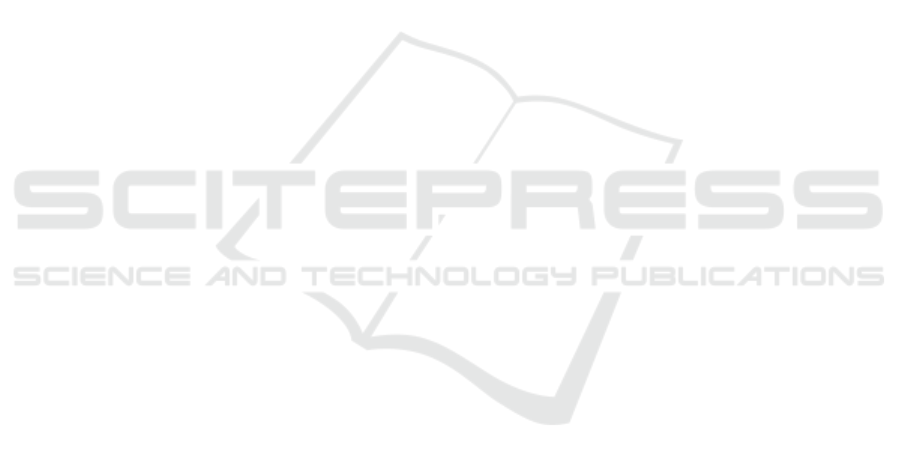
Hu, G., Hua, Y., Yuan, Y., Zhang, Z., Lu, Z., Mukherjee,
S. S., Hospedales, T. M., Robertson, N. M., and Yang,
Y. (2017). Attribute-enhanced face recognition with
neural tensor fusion networks. In 2017 IEEE Interna-
tional Conference on Computer Vision (ICCV), pages
3764–3773.
Lu, B., Chen, J., Castillo, C. D., and Chellappa, R.
(2018). An experimental evaluation of covariates
effects on unconstrained face verification. CoRR,
abs/1808.05508.
Maze, B., Adams, J., Duncan, J. A., Kalka, N., Miller, T.,
Otto, C., Jain, A. K., Niggel, W. T., Anderson, J., Ch-
eney, J., and Grother, P. (2018). Iarpa janus bench-
mark - c: Face dataset and protocol. In 2018 Inter-
national Conference on Biometrics (ICB), pages 158–
165.
Meng, Q., Zhao, S., Huang, Z., and Zhou, F. (2021).
Magface: A universal representation for face recog-
nition and quality assessment. In Proceedings of the
IEEE/CVF Conference on Computer Vision and Pat-
tern Recognition, pages 14225–14234.
O’Toole, A. J., Castillo, C. D., Parde, C. J., Hill, M. Q., and
Chellappa, R. (2018). Face space representations in
deep convolutional neural networks. Trends in Cogni-
tive Sciences, 22(9):794–809.
Paszke, A., Gross, S., Massa, F., Lerer, A., Bradbury, J.,
Chanan, G., Killeen, T., Lin, Z., Gimelshein, N.,
Antiga, L., Desmaison, A., Kopf, A., Yang, E., De-
Vito, Z., Raison, M., Tejani, A., Chilamkurthy, S.,
Steiner, B., Fang, L., Bai, J., and Chintala, S. (2019).
Pytorch: An imperative style, high-performance deep
learning library. In Wallach, H., Larochelle, H.,
Beygelzimer, A., d'Alch
´
e-Buc, F., Fox, E., and Gar-
nett, R., editors, Advances in Neural Information Pro-
cessing Systems 32, pages 8024–8035. Curran Asso-
ciates, Inc.
Ranjan, R., Patel, V. M., and Chellappa, R. (2019). Hy-
perface: A deep multi-task learning framework for
face detection, landmark localization, pose estimation,
and gender recognition. IEEE Transactions on Pattern
Analysis and Machine Intelligence, 41(1):121–135.
Ranjan, R., Sankaranarayanan, S., Castillo, C. D., and Chel-
lappa, R. (2016). An all-in-one convolutional neural
network for face analysis. CoRR, abs/1611.00851.
Rudd, E. M., G
¨
unther, M., and Boult, T. E. (2016). MOON:
A mixed objective optimization network for the recog-
nition of facial attributes. CoRR, abs/1603.07027.
Samangouei, P. and Chellappa, R. (2016). Convolutional
neural networks for attribute-based active authentica-
tion on mobile devices. In 2016 IEEE 8th Interna-
tional Conference on Biometrics Theory, Applications
and Systems (BTAS), pages 1–8.
Sankaran, N., Mohan, D. D., Tulyakov, S., Setlur, S., and
Govindaraju, V. (2021). Tadpool: Target adaptive
pooling for set based face recognition. In 2021 16th
IEEE International Conference on Automatic Face
and Gesture Recognition (FG 2021), pages 1–8.
Schroff, F., Kalenichenko, D., and Philbin, J. (2015).
Facenet: A unified embedding for face recognition
and clustering. CoRR, abs/1503.03832.
Taherkhani, F., Nasrabadi, N. M., and Dawson, J. M.
(2018). A deep face identification network enhanced
by facial attributes prediction. CoRR, abs/1805.00324.
Terh
¨
orst, P., F
¨
ahrmann, D., Damer, N., Kirchbuchner, F.,
and Kuijper, A. (2020a). Beyond identity: What infor-
mation is stored in biometric face templates? CoRR,
abs/2009.09918.
Terh
¨
orst, P., F
¨
ahrmann, D., Kolf, J. N., Damer, N., Kirch-
buchner, F., and Kuijper, A. (2020b). Maad-face: A
massively annotated attribute dataset for face images.
CoRR, abs/2012.01030.
Wang, Z., He, K., Fu, Y., Feng, R., Jiang, Y.-G., and Xue, X.
(2017). Multi-task deep neural network for joint face
recognition and facial attribute prediction. In Proceed-
ings of the 2017 ACM on International Conference on
Multimedia Retrieval, ICMR ’17, page 365–374, New
York, NY, USA. Association for Computing Machin-
ery.
Zhang, K., Zhang, Z., Li, Z., and Qiao, Y. (2016). Joint
face detection and alignment using multitask cascaded
convolutional networks. IEEE Signal Processing Let-
ters, 23(10):1499–1503.
VISAPP 2023 - 18th International Conference on Computer Vision Theory and Applications
172