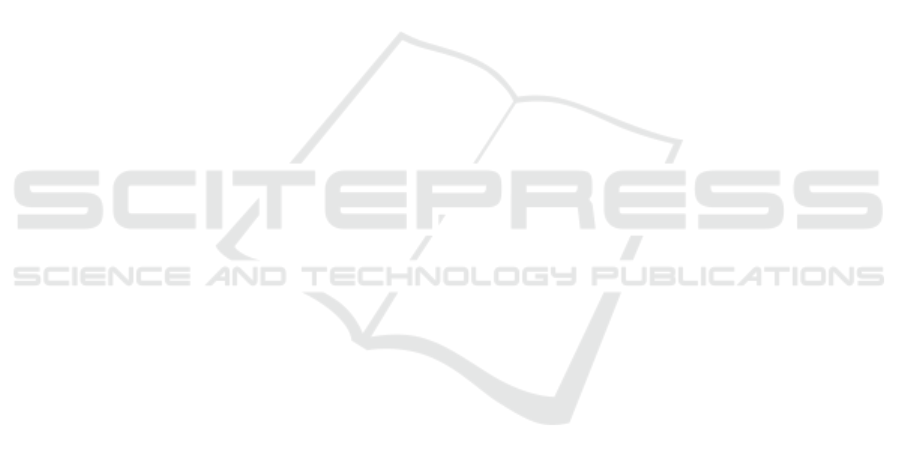
line learning based on the previous approach (Matsui,
2019). For our first investigation, we concentrated on
a case where agents have complete observation of an
environment like in the previous study. On the other
hand, the decomposition of learning tables caused the
aliasing similar to partial observation that affected the
learning process. As a result, a relatively large per-
turbation was caused in the learning process. Since
the solutions of the investigated class of problems re-
quire relatively accurate expected future cost values,
the noise due to the aliasing should be avoided as
much as possible. Mitigation of the influence of the
aliasing and analysis of the allowable range of noise
are a directions of future studies.
To improve the stability of the learning process,
several approaches, including dynamic tuning of the
learning parameters, extending the exploration strate-
gies and filtering the policies, might be effective.
Since the conventional simple aggregation of esti-
mated future cost values mixes different policies,
some analysis of the influence of such an aggrega-
tion is required to improve the learning rules. More-
over, there might be appropriate exploration strategies
to optimize fairness among the agents’ policies.
While we investigated a case of decomposed
learning tables aiming the class of multiagent rein-
forcement learning where agents select their joint ac-
tion by cooperatively solving an optimization prob-
lem, there are different cooperation approaches in-
cluding reward shaping techniques (Agogino and
Tumer, 2004; Devlin et al., 2014). How such tech-
niques including game theoretic approaches can be
applied to our investigated problem will be an inter-
esting issue.
6 CONCLUSION
We investigated the decomposition of multi-objective
reinforcement learning that considers fairness and the
worst case among agents’ action costs toward decen-
tralized multiagent reinforcement learning. Our ex-
perimental results identified the possibility of our pro-
posed approach and revealed the influence of decom-
posed learning tables on the stability of learning. Our
future work will include a detailed and theoretical
analysis of the learning process and improving our
proposed method for more stable learning with dis-
tributed protocols among agents.
ACKNOWLEDGEMENTS
This work was supported in part by JSPS KAKENHI
Grant Number JP22H03647.
REFERENCES
Agogino, A. K. and Tumer, K. (2004). Unifying tempo-
ral and structural credit assignment problems. In the
Third International Joint Conference on Autonomous
Agents and Multiagent Systems, volume 2, pages 980–
987.
Awheda, M. D. and Schwartz, H. M. (2016). Exponen-
tial moving average based multiagent reinforcement
learning algorithms. Artificial Intelligence Review,
45(3):299–332.
Bouveret, S. and Lema
ˆ
ıtre, M. (2009). Computing leximin-
optimal solutions in constraint networks. Artificial In-
telligence, 173(2):343–364.
Devlin, S., Yliniemi, L., Kudenko, D., and Tumer, K.
(2014). Potential-based difference rewards for mul-
tiagent reinforcement learning. In the 13th Interna-
tional Conference on Autonomous Agents and Multia-
gent System, pages 165–172.
Fioretto, F., Pontelli, E., and Yeoh, W. (2018). Distributed
constraint optimization problems and applications: A
survey. Journal of Artificial Intelligence Research,
61:623–698.
Greco, G. and Scarcello, F. (2013). Constraint satisfac-
tion and fair multi-objective optimization problems:
Foundations, complexity, and islands of tractability.
In Proc. 23th International Joint Conference on Arti-
ficial Intelligence, pages 545–551.
Hu, J. and Wellman, M. P. Nash Q-learning for General-
sum Stochastic Games. Journal of Machine Learning
Research.
Hu, Y., Gao, Y., and An, B. (2015). Multiagent reinforce-
ment learning with unshared value functions. IEEE
Transactions on Cybernetics, 45(4):647–662.
Liu, C., Xu, X., and Hu, D. (2015). Multiobjective rein-
forcement learning: A comprehensive overview. IEEE
Transactions on Systems, Man, and Cybernetics: Sys-
tems, 45(3):385–398.
Matsui, T. (2019). A Study of Joint Policies Considering
Bottlenecks and Fairness. In Proc. 11th International
Conference on Agents and Artificial Intelligence, vol-
ume 1, pages 80–90.
Matsui, T. (2022). Study on Applying Decentralized Evo-
lutionary Algorithm to Asymmetric Multi-objective
DCOPs with Fairness and Worst Case. In Proc. 14th
International Conference on Agents and Artificial In-
telligence, volume 1, pages 417–424.
Matsui, T., Matsuo, H., Silaghi, M., Hirayama, K., and
Yokoo, M. (2018). Leximin asymmetric multiple
objective distributed constraint optimization problem.
Computational Intelligence, 34(1):49–84.
Moffaert, K. V., Drugan, M. M., and Now
´
e, A. (2013).
Scalarized multi-objective reinforcement learning:
ICAART 2023 - 15th International Conference on Agents and Artificial Intelligence
276