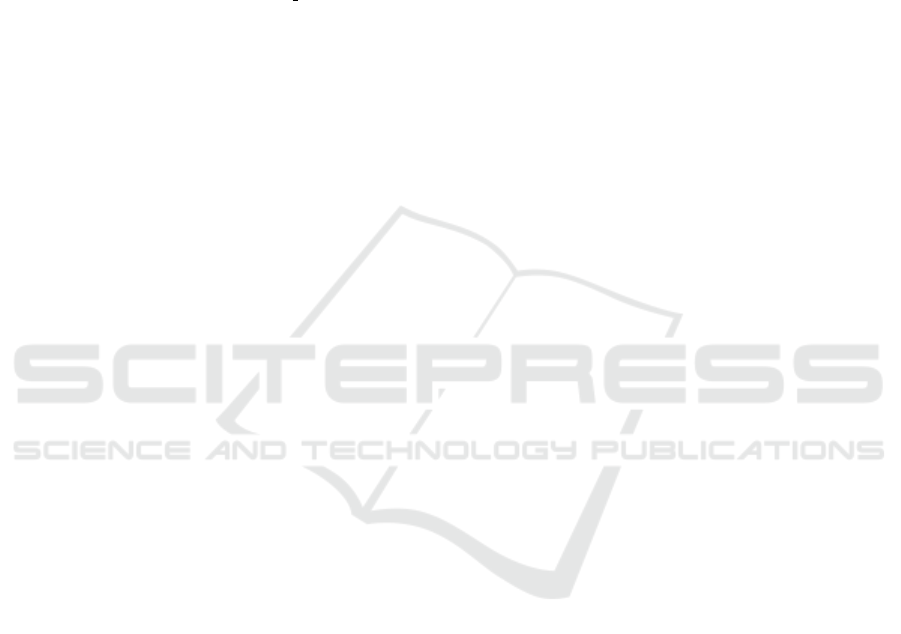
REFERENCES
Akmandor, A. O. and Jha, N. K. (2017). Keep the stress
away with soda: Stress detection and alleviation sys-
tem. IEEE Transactions on Multi-Scale Computing
Systems, 3(4):269–282.
Alberdi, A., Aztiria, A., and Basarab, A. (2016). Towards
an automatic early stress recognition system for office
environments based on multimodal measurements: A
review. Journal of biomedical informatics, 59:49–75.
Biosignals LIBPhys-UNL (2020). Biosignals - prevoccu-
pai. http://biosi.gnals.org/projects pages/prevocupai/
prevocupai.html. Accessed: 2022-10-20.
Bobade, P. and Vani, M. (2020). Stress detection with ma-
chine learning and deep learning using multimodal
physiological data. In 2020 Second International Con-
ference on Inventive Research in Computing Applica-
tions (ICIRCA), pages 51–57. IEEE.
Bolliger, L., Lukan, J., Colman, E., Boersma, L., Lu
ˇ
strek,
M., De Bacquer, D., and Clays, E. (2022). Sources
of occupational stress among office workers—a focus
group study. International Journal of Environmental
Research and Public Health, 19(3):1075.
Boonnithi, S. and Phongsuphap, S. (2011). Comparison of
heart rate variability measures for mental stress detec-
tion. In 2011 Computing in Cardiology, pages 85–88.
IEEE.
Brouwer, A.-M. and Hogervorst, M. A. (2014). A new
paradigm to induce mental stress: the sing-a-song
stress test (ssst). Frontiers in neuroscience, 8:224.
Can, Y. S., Arnrich, B., and Ersoy, C. (2019). Stress de-
tection in daily life scenarios using smart phones and
wearable sensors: A survey. volume 92. Academic
Press Inc.
Choi, J., Ahmed, B., and Gutierrez-Osuna, R. (2011). De-
velopment and evaluation of an ambulatory stress
monitor based on wearable sensors. IEEE trans-
actions on information technology in biomedicine,
16(2):279–286.
Ciabattoni, L., Ferracuti, F., Longhi, S., Pepa, L., Romeo,
L., and Verdini, F. (2017). Real-time mental stress de-
tection based on smartwatch. pages 110–111. Institute
of Electrical and Electronics Engineers Inc.
Curtarelli, M. (2022). Osh pulse - occupational safety and
health in post-pandemic worplaces.
Fraunhofer AICOS (2021). tsfel.utils package.
https://tsfel.readthedocs.io/en/latest/descriptions/
modules/tsfel.utils.html. Accessed: 2022-12-19.
Goncalves, F., Carneiro, D., P
ˆ
ego, J., and Novais, P. (2018).
X3s: A multi-modal approach to monitor and assess
stress through human-computer interaction. Com-
puter Science and Information Systems, 15:683–703.
Hermens, H. J., Freriks, B., Disselhorst-Klug, C., and Rau,
G. (2000). Development of recommendations for
semg sensors and sensor placement procedures. Jour-
nal of electromyography and Kinesiology, 10(5):361–
374.
Kurt, S. and Osueke, K. K. (2014). The effects of
color on the moods of college students. sage Open,
4(1):2158244014525423.
Lawanont, W., Mongkolnam, P., Nukoolkit, C., and Inoue,
M. (2018). Daily stress recognition system using ac-
tivity tracker and smartphone based on physical ac-
tivity and heart rate data. In International confer-
ence on intelligent decision technologies, pages 11–
21. Springer.
Lin, Z., Kunze, K., Ueki, A., and Inakage, M. (2020).
Aromacue-a scent toolkit to cope with stress using the
4-7-8 breathing method. In Proceedings of the Four-
teenth International Conference on Tangible, Embed-
ded, and Embodied Interaction, pages 265–272.
Pedregosa, F., Varoquaux, G., Gramfort, A., Michel, V.,
Thirion, B., Grisel, O., Blondel, M., Prettenhofer, P.,
Weiss, R., Dubourg, V., et al. (2011). Scikit-learn:
Machine learning in python. the Journal of machine
Learning research, 12:2825–2830.
Pourmohammadi, S. and Maleki, A. (2020). Stress de-
tection using ecg and emg signals: A comprehen-
sive study. Computer methods and programs in
biomedicine, 193:105482.
Salai, M., Vass
´
anyi, I., and K
´
osa, I. (2016). Stress detection
using low cost heart rate sensors. Journal of health-
care engineering, 2016.
Shi, Y., Nguyen, M. H., Blitz, P., French, B., Fisk, S., De la
Torre, F., Smailagic, A., Siewiorek, D. P., al’Absi, M.,
Ertin, E., et al. (2010). Personalized stress detection
from physiological measurements. In International
symposium on quality of life technology, pages 28–29.
Siirtola, P. (2019). Continuous stress detection using the
sensors of commercial smartwatch. pages 1198–1201.
Association for Computing Machinery, Inc.
Silva, S., Cepeda, C., Rodrigues, J., Probst, P., and Gam-
boa, H. (2022). Assessing occupational health with
a cross-platform application based on self-reports and
biosignals. In HEALTHINF, pages 549–556.
Um, E., Plass, J. L., Hayward, E. O., Homer, B. D., et al.
(2012). Emotional design in multimedia learning.
Journal of educational psychology, 104(2):485.
Wijsman, J., Grundlehner, B., Liu, H., Hermens, H., and
Penders, J. (2011). Towards mental stress detection
using wearable physiological sensors. In 2011 An-
nual International Conference of the IEEE Engineer-
ing in Medicine and Biology Society, pages 1798–
1801. IEEE.
Wijsman, J., Grundlehner, B., Liu, H., Penders, J., and
Hermens, H. (2013a). Wearable physiological sen-
sors reflect mental stress state in office-like situations.
In 2013 humaine association conference on affective
computing and intelligent interaction, pages 600–605.
IEEE.
Wijsman, J., Grundlehner, B., Penders, J., and Hermens, H.
(2013b). Trapezius muscle emg as predictor of men-
tal stress. ACM transactions on embedded computing
systems (TECS), 12(4):1–20.
Zeiler, A., Faltermeier, R., Keck, I. R., Tom
´
e, A. M., Pun-
tonet, C. G., and Lang, E. W. (2010). Empirical mode
decomposition-an introduction. In The 2010 interna-
tional joint conference on neural networks (IJCNN),
pages 1–8. IEEE.
BIOSIGNALS 2023 - 16th International Conference on Bio-inspired Systems and Signal Processing
272