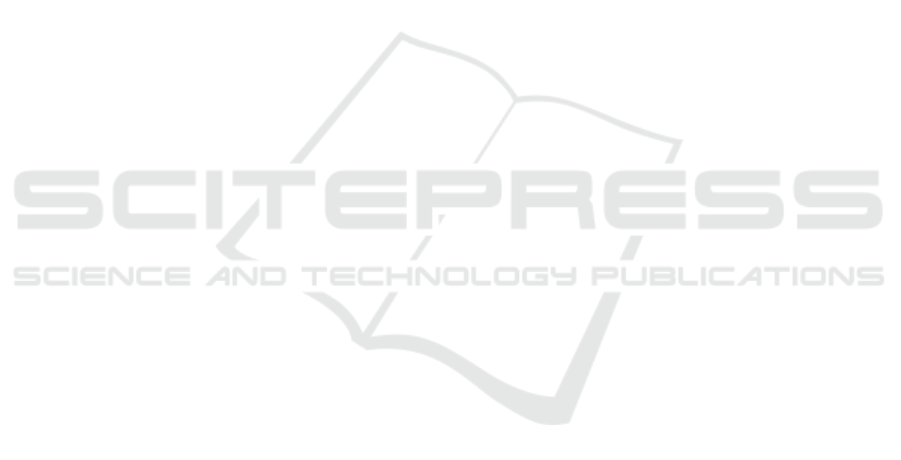
posed DNNAM system can be used in conjunction
with unmanned aerial vehicles (UAVs) to quickly
identify structural elements and can accurately detect
deterioration, anticipate how long a structure will last
and monitor large concrete structures.
REFERENCES
Bhattacharya, G., Puhan, N. B., and Mandal, B. (2021).
Stand-alone composite attention network for concrete
structural defect classification. IEEE Transactions on
Artificial Intelligence, 3(2):265–274.
Cordonnier, J.-B., Loukas, A., and Jaggi, M. (2019). On the
relationship between self-attention and convolutional
layers. arXiv preprint arXiv:1911.03584.
Gao, Y. and Mosalam, K. M. (2018). Deep transfer learn-
ing for image-based structural damage recognition.
Computer-Aided Civil and Infrastructure Engineer-
ing, 33(9):748–768.
Guo, H., Zheng, K., Fan, X., Yu, H., and Wang, S. (2019).
Visual attention consistency under image transforms
for multi-label image classification. In Proceedings
of the IEEE/CVF conference on computer vision and
pattern recognition, pages 729–739.
He, K., Zhang, X., Ren, S., and Sun, J. (2016). Deep resid-
ual learning for image recognition. In Proceedings of
the IEEE conference on computer vision and pattern
recognition, pages 770–778.
Hu, J., Shen, L., and Sun, G. (2018). Squeeze-and-
excitation networks. In Proceedings of the IEEE con-
ference on computer vision and pattern recognition,
pages 7132–7141.
Kaothalkar, A., Mandal, B., and Puhan, N. B. (2022). Struc-
turenet: Deep context attention learning for structural
component recognition. In Farinella, G. M., Radeva,
P., and Bouatouch, K., editors, Proceedings of the 17th
International Joint Conference on Computer Vision,
pages 567–573. SCITEPRESS.
Liang, X. (2019). Image-based post-disaster inspection of
reinforced concrete bridge systems using deep learn-
ing with bayesian optimization. Computer-Aided Civil
and Infrastructure Engineering, 34(5):415–430.
Liu, B., Gould, S., and Koller, D. (2010). Single im-
age depth estimation from predicted semantic labels.
In 2010 IEEE computer society conference on com-
puter vision and pattern recognition, pages 1253–
1260. IEEE.
Narazaki, Y., Hoskere, V., Hoang, T. A., Fujino, Y., Sakurai,
A., and Spencer Jr, B. F. (2020). Vision-based auto-
mated bridge component recognition with high-level
scene consistency. Computer-Aided Civil and Infras-
tructure Engineering, 35(5):465–482.
Narazaki, Y., Hoskere, V., Hoang, T. A., and Spencer, B. F.
(2017). Vision-based automated bridge component
recognition integrated with high-level scene under-
standing. arXiv preprint arXiv:1805.06041.
Narazaki, Y., Hoskere, V., Hoang, T. A., and Spencer Jr,
B. F. (2018). Automated vision-based bridge compo-
nent extraction using multiscale convolutional neural
networks. arXiv preprint arXiv:1805.06042.
Noh, H., Hong, S., and Han, B. (2015). Learning de-
convolution network for semantic segmentation. In
Proceedings of the IEEE international conference on
computer vision, pages 1520–1528.
Park, J., Woo, S., Lee, J.-Y., and Kweon, I. S. (2018).
Bam: Bottleneck attention module. arXiv preprint
arXiv:1807.06514.
Saxena, A., Chung, S., and Ng, A. (2005). Learning depth
from single monocular images. Advances in neural
information processing systems, 18.
Saxena, A., Sun, M., and Ng, A. Y. (2008). Make3d: Learn-
ing 3d scene structure from a single still image. IEEE
transactions on pattern analysis and machine intelli-
gence, 31(5):824–840.
Spencer, B. F., Hoskere, V., and Narazaki, Y. (2019). Ad-
vances in computer vision-based civil infrastructure
inspection and monitoring. Engineering, 5(2):199–
222.
Tan, Z., Yang, Y., Wan, J., Hang, H., Guo, G., and Li, S. Z.
(2019). Attention-based pedestrian attribute analysis.
IEEE transactions on image processing, 28(12):6126–
6140.
Vaswani, A., Shazeer, N., Parmar, N., Uszkoreit, J., Jones,
L., Gomez, A. N., Kaiser, Ł., and Polosukhin, I.
(2017). Attention is all you need. Advances in neural
information processing systems, 30.
Wang, F., Jiang, M., Qian, C., Yang, S., Li, C., Zhang, H.,
Wang, X., and Tang, X. (2017). Residual attention
network for image classification. In Proceedings of
the IEEE conference on computer vision and pattern
recognition, pages 3156–3164.
Wang, W. and Shen, J. (2017). Deep visual attention pre-
diction. IEEE Transactions on Image Processing,
27(5):2368–2378.
Woo, S., Park, J., Lee, J.-Y., and Kweon, I. S. (2018). Cbam:
Convolutional block attention module. In Proceed-
ings of the European conference on computer vision
(ECCV), pages 3–19.
Yeum, C. M., Choi, J., and Dyke, S. J. (2019). Automated
region-of-interest localization and classification for
vision-based visual assessment of civil infrastructure.
Structural Health Monitoring, 18(3):675–689.
Zhang, F., Chen, Y., Li, Z., Hong, Z., Liu, J., Ma, F., Han, J.,
and Ding, E. (2019). Acfnet: Attentional class feature
network for semantic segmentation. In Proceedings of
the IEEE/CVF International Conference on Computer
Vision, pages 6798–6807.
Zhou, S., Wang, J., Zhang, J., Wang, L., Huang, D., Du, S.,
and Zheng, N. (2020). Hierarchical u-shape attention
network for salient object detection. IEEE Transac-
tions on Image Processing, 29:8417–8428.
Zhu, Y., Zhao, C., Guo, H., Wang, J., Zhao, X., and Lu,
H. (2018). Attention couplenet: Fully convolutional
attention coupling network for object detection. IEEE
Transactions on Image Processing, 28(1):113–126.
VISAPP 2023 - 18th International Conference on Computer Vision Theory and Applications
326