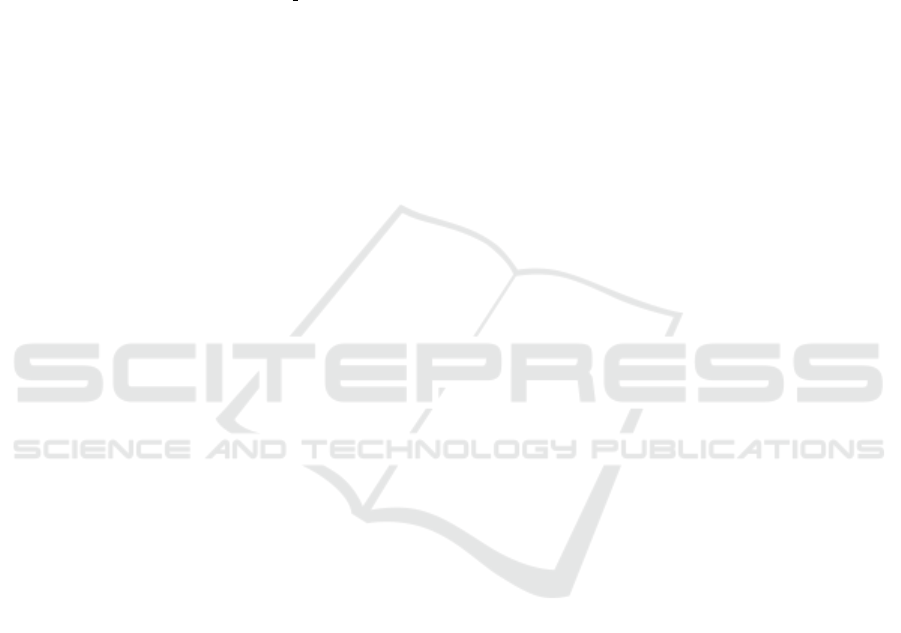
REFERENCES
Alsubaie, A. M., Mazaheri, M., Martinez-Valdes, E., and
Falla, D. (2021). Is movement variability altered in
people with chronic non-specific low back pain: a pro-
tocol for a systematic review. BMJ Open, 11(5).
Arippa, F., Nguyen, A., Pau, M., and Harris-Adamson, C.
(2022). Postural strategies among office workers dur-
ing a prolonged sitting bout. Applied Ergonomics,
102:103723.
Biosignals LIBPhys-UNL (2020). Biosignals - prevoccu-
pai. http://biosi.gnals.org/projects pages/prevocupai/
prevocupai.html. Accessed: 2022-10-20.
Bontrup, C., Taylor, W. R., Fliesser, M., Visscher, R.,
Green, T., Wippert, P.-M., and Zemp, R. (2019). Low
back pain and its relationship with sitting behaviour
among sedentary office workers. Applied Ergonomics,
81:102894.
Carnide, F., Veloso, A., Gamboa, H., Caldeira, S., and
Fragoso, I. (2006). Interaction of biomechanical and
morphological factors on shoulder workload in indus-
trial paint work. Clinical Biomechanics, 21(Suppl.
1):S33–S38.
Chen, Y.-L., Chan, Y.-C., and Zhang, L.-P. (2021). Pos-
tural variabilities associated with the most comfort-
able sitting postures: A preliminary study. Health-
care, 9(12):1685.
Forsman, P., Haeggstr
¨
om, E., Wallin, A., Toppila, E., and
Pyykk
¨
o, I. (2007). Daytime changes in postural stabil-
ity and repeatability of posturographic measurements.
Journal of occupational and environmental medicine,
49(6):591–596.
Goldman, R. (2011). Understanding quaternions. Graphi-
cal Models, 73(2):21–49.
Hulshof, C. T., Pega, F., Neupane, S., van der Molen, H. F.,
Colosio, C., Daams, J. G., Descatha, A., Kc, P., Kuijer,
P. P., Mandic-Rajcevic, S., Masci, F., Morgan, R. L.,
Nyg
˚
ard, C.-H., Oakman, J., Proper, K. I., Solovieva,
S., and Frings-Dresen, M. H. (2021). The prevalence
of occupational exposure to ergonomic risk factors: A
systematic review and meta-analysis from the who/ilo
joint estimates of the work-related burden of disease
and injury. Environment International, 146:106157.
Jorgensen, M., Rathleff, M., Laessoe, U., Caserotti, P.,
Nielsen, O., and Aagaard, P. (2012). Time-of-day in-
fluences postural balance in older adults. Gait & Pos-
ture, 35(4):653–657.
Jun, D., Johnston, V., McPhail, S. M., and O’Leary, S.
(2019). Are measures of postural behavior using mo-
tion sensors in seated office workers reliable? Human
Factors, 61(7):1141–1161.
Kelson, D. M., Mathiassen, S. E., and Srinivasan, D. (2019).
Trapezius muscle activity variation during computer
work performed by individuals with and without neck-
shoulder pain. Applied Ergonomics, 81:102908.
Lenzi, S. E., Standoli, C. E., Andreoni, G., Perego, P.,
and Lopomo, N. F. (2018). Comparison among stan-
dard method, dedicated toolbox and kinematic-based
approach in assessing risk of developing upper limb
musculoskeletal disorders. In Advances in Human
Factors in Wearable Technologies and Game Design,
pages 135–145. Springer International Publishing.
Madeleine, P., Marandi, R. Z., Norheim, K. L., Andersen,
J. B., and Samani, A. (2021). Sitting dynamics dur-
ing computer work are age-dependent. Applied Er-
gonomics, 93:103391.
Mingels, S., Dankaerts, W., van Etten, L., Bruckers, L., and
Granitzer, M. (2021). Lower spinal postural variabil-
ity during laptop-work in subjects with cervicogenic
headache compared to healthy controls. Scientific re-
ports, 11(1):1–11.
Paterno, M. V., Schmitt, L. C., Ford, K. R., Rauh, M. J., and
Hewett, T. E. (2013). Altered postural sway persists
after anterior cruciate ligament reconstruction and re-
turn to sport. Gait & Posture, 38(1):136–140.
Perista, H., Cardoso, A., Nunes, J., Carrilho, P., and Quin-
tal, E. (2016). Inqu
´
erito
`
as condic¸
˜
oes de trabalho em
portugal continental: Trabalhadores/as.
Ryan, C. G., Dall, P. M., Granat, M. H., and Grant, P. M.
(2011). Sitting patterns at work: objective measure-
ment of adherence to current recommendations. Er-
gonomics, 54(6):531–538.
Samani, A., Holtermann, A., Søgaard, K., and Madeleine, P.
(2010). Active biofeedback changes the spatial distri-
bution of upper trapezius muscle activity during com-
puter work. European journal of applied physiology,
110(2):415–423.
Silva, S., Cepeda, C., Rodrigues, J., Probst, P., and Gam-
boa, H. (2022). Assessing occupational health with
a cross-platform application based on self-reports and
biosignals. In Proceedings of the 15th International
Joint Conference on Biomedical Engineering Systems
and Technologies - HEALTHINF, pages 549–556.
SciTePress.
Soames, R. W. and Atha, J. (1982). The spectral character-
istics of postural sway behaviour. European Journal
of Applied Physiology and Occupational Physiology,
49:169–177.
Son, S. M. (2017). The circadian effects on postural stabil-
ity in young adults. The Journal of Korean Physical
Therapy, 29(3):142–144.
Srinivasan, D. and Mathiassen, S. E. (2012). Motor vari-
ability - an important issue in occupational life. Work,
41(Suppl. 1):2527–2534.
Søndergaard, K. H., Olesen, C. G., Søndergaard, E. K.,
de Zee, M., and Madeleine, P. (2010). The variabil-
ity and complexity of sitting postural control are as-
sociated with discomfort. Journal of Biomechanics,
43(10):1997–2001.
World Health Organization (2017). Protecting workers’
health. https://www.who.int/news-room/fact-sheets/
detail/protecting-workers’-health. Accessed: 2022-
10-20.
BIOSIGNALS 2023 - 16th International Conference on Bio-inspired Systems and Signal Processing
280