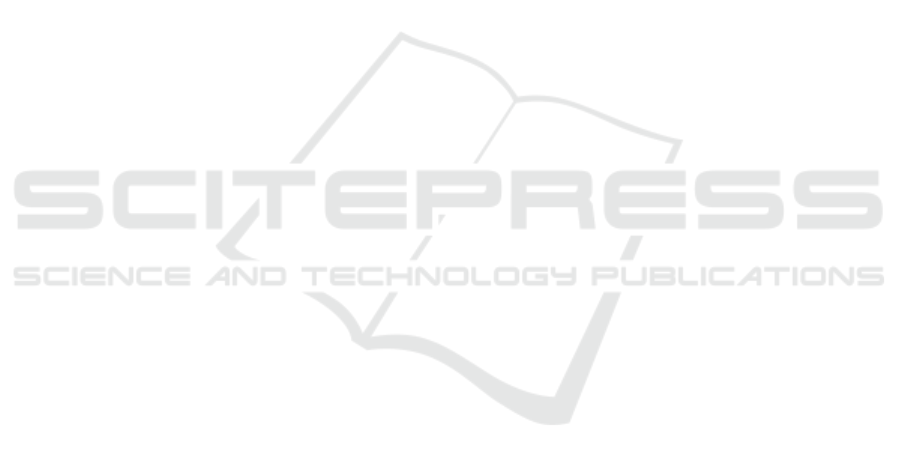
5.2 Results and Analysis
We generated alternatives to the 3 initial plans by
varying the duration with a step of 50 minutes. For
each of these durations we varied the maximum num-
ber of errors for each LU. What can be observed first,
for the 2 different cases, is that the number of gener-
ated plans remains quite low for Duration < 300 with
a number of generated plans lower than 500 what-
ever the initial plan and the number of allowed er-
rors. The number of generated alternatives increases
sharply between 300 and 400 minutes, but the con-
straints still allow the plans to be generated with-
out problems. We also note that with such duration
constraints, the number of generated plans varies lit-
tle for MaxErr > 3. Although the computations run
smoothly for the duration interval [100−400], there is
a large variation in the number of generated plans de-
pending on the length of the initial plan which varies
from a few hundred for an initial plan composed of
3 LU to more than 10000 for an initial plan com-
posed of 4 LU. These results therefore show that our
method works for generating alternatives to the ini-
tial plan. The number of generated plans is high and
offers many possibilities in order to select the most
suitable alternative plan for the encountered situation.
The maximum duration used for our tests is 400 min-
utes. However, this time is only reached when several
errors are made and the initial plan, if it is carried out
without errors, is much faster. It is therefore neces-
sary to think carefully about the skills that the trainer
wants to work on and the parameters of the limits ac-
cording to the type of session that the trainer wants to
set up. Thus, thanks to our system, it is quite possi-
ble to propose a rather short session but leaving the
possibility of many attempts. This allows the learner
to take more time, or, in case of quick success, to
start a new training session. Alternatively, our system
also allows for longer sessions, with more skills be-
ing worked on, but with less opportunity for mistakes
to be made. These possibilities of adaptation make it
possible to offer training sessions to different learner
profiles such as beginners or experienced operators.
6 CONCLUSIONS
In this paper we have presented an original solution
to design and plan training scenarios. Hybrid plan-
ning and the use of ANML allow us to take ad-
vantage of both the generative power of planning
and the control provided by HTNs. This structure
also greatly improves the expressiveness of our plans
thanks to the separation of scenario and pedagogical
content. Finally, this method improves the robust-
ness of our scenario and its resilience with respect to
its objectives thanks to an efficient generation of alter-
native plans allowing to anticipate the potential drifts
of the scenario and to repair them. Finally, these el-
ements were integrated into an interface allowing the
trainer to follow the scenario execution live and to ap-
ply the generated alternatives in case of drift. In or-
der to complete the system, we will study in our next
work the strategies of qualitative selection of the gen-
erated alternatives so that the repair of the scenario
corresponds as well as possible to the initial objec-
tives and the preferences of the trainers. This work
will be accompanied by the development of the moni-
toring system to support the execution of the scenario,
to detect drift situations and to implement the alterna-
tives.
ACKNOWLEDGEMENTS
This work is financed by the DGA RAPID ORCHES-
TRAA project.
REFERENCES
Aylett, R., Dias, J., and Paiva, A. (2006). An affectively
driven planner for synthetic characters. In Icaps,
pages 2–10.
Gupta, S. K., Anand, D. K., Brough, J. E., Schwartz, M.,
and Kavetsky, R. (2008). Training in Virtual Envi-
ronments: A Safe, Cost-Effective, and Engaging Ap-
proach to Training. University of Maryland.
Howe, A., Knoblock, C., McDermott, I. D., Ram, A.,
Veloso, M., Weld, D., SRI, D. W., Barrett, A., Chris-
tianson, D., et al. (1998). Pddl— the planning domain
definition language. Technical Report, Tech. Rep.
Porteous, J. (2016). Planning technologies for interactive
storytelling. In Handbook of Digital Games and En-
tertainment Technologies. Springer.
Porteous, J., Teutenberg, J., Charles, F., and Cavazza, M.
(2011). Controlling narrative time in interactive story-
telling. In The 10th International Conference on Au-
tonomous Agents and Multiagent Systems-Volume 2,
pages 449–456.
Ramirez, A. and Bulitko, V. (2014). Automated planning
and player modeling for interactive storytelling. IEEE
Transactions on Computational Intelligence and AI in
Games, 7(4):375–386.
Riedl, M., Saretto, C. J., and Young, R. M. (2003). Manag-
ing interaction between users and agents in a multi-
agent storytelling environment. In Proceedings of
the 2nd international joint conference on Autonomous
agents and multiagent systems, pages 741–748.
Automated Planning for Military Airline Controller Training Scenarios
473