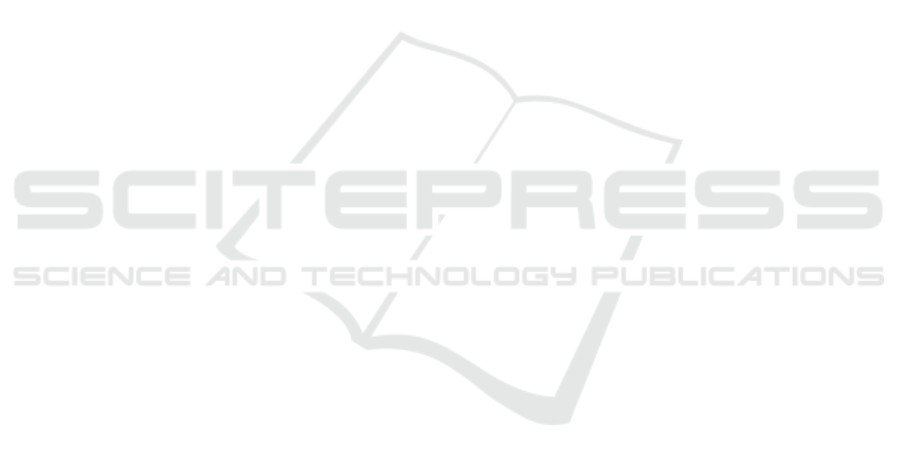
technological developments in online safety and se-
curity.
REFERENCES
Al-Rousan, S., Abuhussein, A., Alsubaei, F., Kahveci, O.,
Farra, H., and Shiva, S. (2020). Social-guard: detect-
ing scammers in online dating. In 2020 IEEE Interna-
tional Conference on Electro Information Technology
(EIT), pages 416–422. IEEE.
Barbero, F., Pendlebury, F., Pierazzi, F., and Cavallaro, L.
(2020). Transcending TRANSCEND: Revisiting mal-
ware classification with conformal evaluation. arXiv
preprint arXiv:2010.03856.
Bartlett, P. L. and Wegkamp, M. H. (2008). Classification
with a reject option using a hinge loss. Journal of
Machine Learning Research, 9(8).
Beldo, S. (2022). What percentage of dating profiles
are fake? https://blog.sift.com/what-percentage-of-
dating-profiles-are-fake/, accessed 2022-10-18.
Edwards, M., Suarez-Tangil, G., Peersman, C., Stringhini,
G., Rashid, A., and Whitty, M. (2018). The geography
of online dating fraud. In Workshop on Technology
and Consumer Protection. IEEE.
FTC (2022). FTC data show show romance scams
hit record high; $547 million reported lost in
2021. https://www.ftc.gov/news-events/news/press-
releases/2022/02/ftc-data-show-romance-scams-
hit-record-high-547-million-reported-lost-2021,
accessed 2022-10-18.
He, X., Gong, Q., Chen, Y., Zhang, Y., Wang, X., and Fu, X.
(2021). DatingSec: Detecting malicious accounts in
dating apps using a content-based attention network.
IEEE Transactions on Dependable and Secure Com-
puting, 18(5):2193–2208.
Huang, J., Stringhini, G., and Yong, P. (2015). Quit play-
ing games with my heart: Understanding online dat-
ing scams. In International Conference on Detection
of Intrusions and Malware, and Vulnerability Assess-
ment, pages 216–236. Springer.
Jordaney, R., Sharad, K., Dash, S. K., Wang, Z., Papini, D.,
Nouretdinov, I., and Cavallaro, L. (2017). Transcend:
Detecting concept drift in malware classification mod-
els. In 26th USENIX Security Symposium (USENIX
Security 17), pages 625–642.
Kubat, M. (2017). An Introduction to Machine Learning,
volume 2. Springer.
Pendlebury, F., Pierazzi, F., Jordaney, R., Kinder, J., and
Cavallaro, L. (2019). TESSERACT: Eliminating ex-
perimental bias in malware classification across space
and time. In 28th USENIX Security Symposium
(USENIX Security 19), pages 729–746.
Price, B. and Edwards, M. (2020). Resource networks of pet
scam websites. In Proceedings of the Symposium on
Electronic Crime Research (eCrime). Anti-Phishing
Working Group.
Rege, A. (2009). What’s love got to do with it? Exploring
online dating scams and identity fraud. International
Journal of Cyber Criminology, 3(2).
scikit-learn developers (2022). Histgradientboostingclassi-
fier. https://scikit-learn.org/stable/modules/generated/
sklearn.ensemble.HistGradientBoostingClassifier.
html, accessed 2022-10-18.
Shen, X., Lv, W., Qiu, J., Kaur, A., Xiao, F., and Xia, F.
(2022). Trust-aware detection of malicious users in
dating social networks. IEEE Transactions on Com-
putational Social Systems.
Singh, A., Walenstein, A., and Lakhotia, A. (2012). Track-
ing concept drift in malware families. In Proceedings
of the 5th ACM workshop on Security and Artificial
Intelligence, pages 81–92.
Suarez-Tangil, G., Edwards, M., Peersman, C., Stringhini,
G., Rashid, A., and Whitty, M. (2020). Automati-
cally dismantling online dating fraud. IEEE Transac-
tions on Information Forensics and Security, 15:1128–
1137.
Webb, G. I., Lee, L. K., Petitjean, F., and Goethals, B.
(2017). Understanding concept drift. arXiv preprint
arXiv:1704.00362.
Whitty, M. T. and Buchanan, T. (2012). The online romance
scam: A serious cybercrime. CyberPsychology, Be-
havior, and Social Networking, 15(3):181–183.
ICISSP 2023 - 9th International Conference on Information Systems Security and Privacy
542