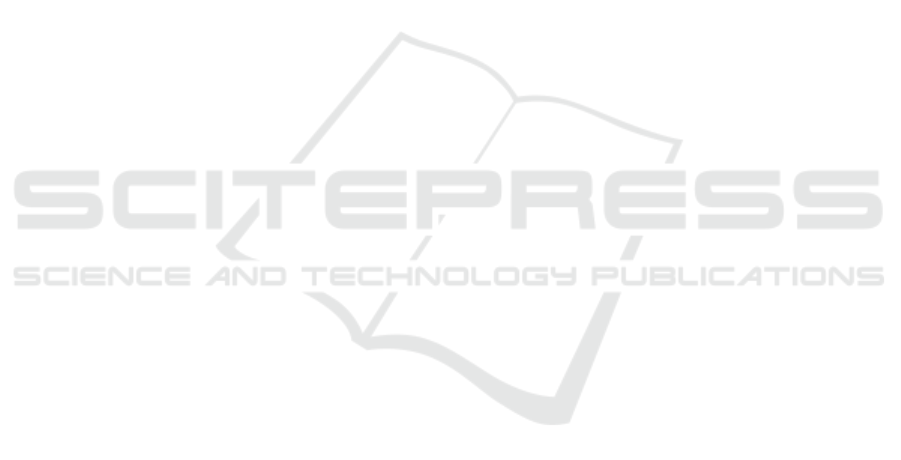
ICD9-CM and ATC codes. The classification results
between real and synthetic samples also prove that
these samples are hard to discriminate. Regarding
the results in the multi-class scenario, the identifi-
cation of patients with multiple chronic conditions
was improved (specifically for patients assigned to
CRG-6191, CRG-7080, and CRG-7081). Further re-
search may explore cost-sensitive learning methods
and GAN-based models that handle categorical and
numerical features aiming to improve the classifica-
tion results. Our study highlights the effectiveness of
GAN-based models to work with a high-dimensional
and sparse clinical dataset, allowing us to create real-
istic patient data and improve prediction performance.
ACKNOWLEDGMENT
This work was partly funded by the Spanish Re-
search Agency, grant numbers PID2019-106623RB-
C41/AEI/10.13039/501100011033 (BigTheory) and
PID2019-107768RA-I00 (AAVis-BMR) funded by
MCIN/AEI/10.13039/501100011033, by the Com-
munity of Madrid in the framework “Encourage-
ment of Young Phd students investigation” (Mapping-
UCI, F661), and by the European Union NextGenera-
tionEU funds (Youth Employment Plan of the Spanish
Government) in the INVESTIGO project with refer-
ence URJC-AI-11.
REFERENCES
American Medical Association (2004). International Clas-
sification of Diseases, 9th Revision, Clinical Modifi-
cation.
Baowaly, M. K., Lin, C.-C., Liu, C.-L., and Chen, K.-T.
(2019). Synthesizing electronic health records us-
ing improved generative adversarial networks. Jour-
nal of the American Medical Informatics Association,
26(3):228–241.
Bishop, C. M. (2006). Pattern Recognition and Ma-
chine Learning (Information Science and Statistics).
Springer-Verlag, Berlin, Heidelberg.
Bouza, C., Lopez-Cuadrado, T., and Amate-Blanco, J.
(2016). Use of explicit ICD9-CM codes to identify
adult severe sepsis: impacts on epidemiological esti-
mates. Critical Care, 20(1):313.
Breiman, L., Friedman, J. H., Olshen, R. A., and Stone,
C. J. (2017). Classification and regression trees. Rout-
ledge.
Budreviciute, A. et al. (2020). Management and preven-
tion strategies for non-communicable diseases (ncds)
and their risk factors. Frontiers in Public Health,
8:574111.
Cao, Y.-J., Jia, L.-L., Chen, Y.-X., Lin, N., Yang, C., Zhang,
B., Liu, Z., Li, X.-X., and Dai, H.-H. (2018). Recent
advances of generative adversarial networks in com-
puter vision. IEEE Access, 7:14985–15006.
Chawla, N. V., Bowyer, K. W., Hall, L. O., and Kegelmeyer,
W. P. (2002). Smote: synthetic minority over-
sampling technique. Journal of Artificial Intelligence
Research, 16:321–357.
Choi, E., Biswal, S., Malin, B., Duke, J., Stewart, W. F., and
Sun, J. (2017). Generating multi-label discrete patient
records using generative adversarial networks. In Proc
of the Machine learning for Healthcare Conference,
pages 286–305, Boston, Massachusetts.
Chong, J. L., Lim, K. K., and Matchar, D. B. (2019). Popu-
lation segmentation based on healthcare needs: a sys-
tematic review. Systematic Reviews, 8(1):1–11.
Chushig-Muzo, D., Soguero-Ruiz, C., de Miguel-Bohoyo,
P., and Mora-Jim
´
enez, I. (2021). Interpreting clinical
latent representations using autoencoders and proba-
bilistic models. Artificial Intelligence in Medicine,
122:102211.
Chushig-Muzo, D., Soguero-Ruiz, C., de Miguel-Bohoyo,
P., and Mora-Jim
´
enez, I. (2022). Interpreting clinical
latent representations using autoencoders and proba-
bilistic models. BioData Mining, 15(18):1–27.
Creswell, A., White, T., Dumoulin, V., Arulkumaran, K.,
Sengupta, B., and Bharath, A. A. (2018). Generative
adversarial networks: An overview. IEEE Signal Pro-
cessing Magazine, 35(1):53–65.
Engelmann, J. and Lessmann, S. (2021). Conditional
wasserstein gan-based oversampling of tabular data
for imbalanced learning. Expert Systems with Appli-
cations, 174:114582.
Falhammar, H., Lindh, J. D., Calissendorff, J., Skov, J.,
Nathanson, D., and Mannheimer, B. (2019). An-
tipsychotics and severe hyponatremia: A swedish
population–based case–control study. European Jour-
nal of Internal Medicine, 60:71–77.
Finison, K., Mohlman, M., Jones, C., Pinette, M., Jor-
genson, D., Kinner, A., Tremblay, T., and Gottlieb,
D. (2017). Risk-adjustment methods for all-payer
comparative performance reporting in vermont. BMC
Health Services Research, 17(1):1–13.
Hanley, J. A. and McNeil, B. J. (1982). The meaning and
use of the area under a receiver operating characteris-
tic (roc) curve. Radiology, 143(1):29–36.
He, H. and Garcia, E. (2009). Learning from imbalanced
data. IEEE Transactions on Knowledge and Data En-
gineering, 21(9):1263–1284.
Hughes, J. S., Averill, R. F., Eisenhandler, J., Goldfield,
N. I., Muldoon, J., Neff, J. M., and Gay, J. C. (2004).
Clinical Risk Groups (CRGs): a classification system
for risk-adjusted capitation-based payment and health
care management. Medical Care, 42(1):81–90.
Ma, Y. and He, H. (2013). Imbalanced learning: foun-
dations, algorithms, and applications. John Wiley &
Sons.
Palmer, K., Marengoni, A., Forjaz, M. J., Jureviciene, E.,
Laatikainen, et al. (2018). Multimorbidity care model:
Recommendations from the consensus meeting of the
On the Use of Generative Adversarial Networks to Predict Health Status Among Chronic Patients
177