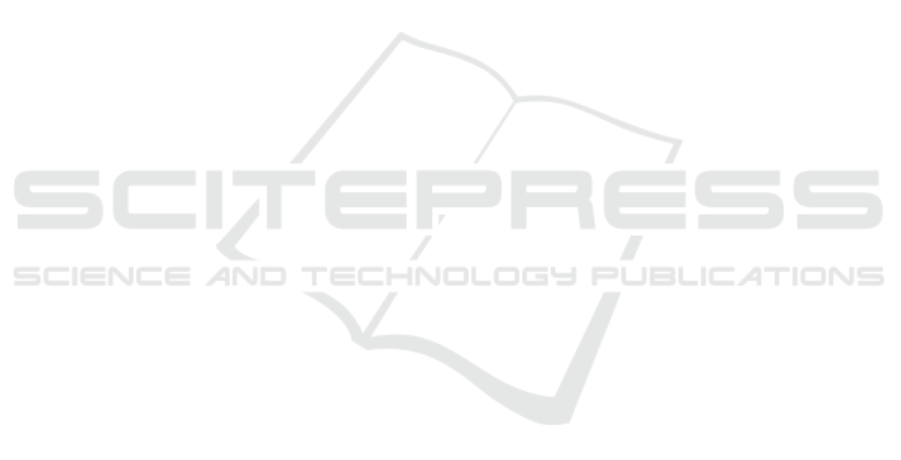
art. IEEE Transactions on Pattern Analysis and Ma-
chine Intelligence, 34(4):743–761.
Feifel, P., Bonarens, F., and K
¨
oster, F. (2021a). Leverag-
ing interpretability: Concept-based pedestrian detec-
tion with deep neural networks. In Computer Science
in Cars Symposium, CSCS ’21. Association for Com-
puting Machinery.
Feifel, P., Bonarens, F., and Koster, F. (2021b). Reevalu-
ating the safety impact of inherent interpretability on
deep neural networks for pedestrian detection. In Pro-
ceedings of the IEEE/CVF Conference on Computer
Vision and Pattern Recognition, pages 29–37.
Feifel, P., Franke, B., Raulf, A., Schwenker, F., Bonarens,
F., and K
¨
oster, F. (2022). Revisiting the evaluation
of deep neural networks for pedestrian detection. In
Proceedings of the Workshop on Artificial Intelligence
Safety 2022.
Geirhos, R., Rubisch, P., Michaelis, C., Bethge, M., Wich-
mann, F. A., and Brendel, W. (2018). Imagenet-
trained cnns are biased towards texture; increasing
shape bias improves accuracy and robustness. arXiv
preprint arXiv:1811.12231.
Ghiasi-Shirazi, K. (2019). Generalizing the convolution op-
erator in convolutional neural networks. Neural Pro-
cessing Letters, 50(3):2627–2646.
Haselhoff, A., Kronenberger, J., Kuppers, F., and Schnei-
der, J. (2021). Towards black-box explainability with
gaussian discriminant knowledge distillation. In Pro-
ceedings of the IEEE/CVF Conference on Computer
Vision and Pattern Recognition, pages 21–28.
Hoyer, L., Dai, D., and Van Gool, L. (2022). Daformer: Im-
proving network architectures and training strategies
for domain-adaptive semantic segmentation. In Pro-
ceedings of the IEEE/CVF Conference on Computer
Vision and Pattern Recognition, pages 9924–9935.
Khan, A. H., Munir, M., van Elst, L., and Dengel, A. (2022).
F2dnet: Fast focal detection network for pedestrian
detection. arXiv preprint arXiv:2203.02331.
Khosla, P., Teterwak, P., Wang, C., Sarna, A., Tian, Y.,
Isola, P., Maschinot, A., Liu, C., and Krishnan, D.
(2020). Supervised contrastive learning. Advances
in Neural Information Processing Systems, 33:18661–
18673.
Kim, B., Wattenberg, M., Gilmer, J., Cai, C., Wexler, J.,
Viegas, F., et al. (2018). Interpretability beyond fea-
ture attribution: Quantitative testing with concept ac-
tivation vectors (tcav). In International conference on
machine learning, pages 2668–2677. PMLR.
Koh, P. W., Nguyen, T., Tang, Y. S., Mussmann, S., Pier-
son, E., Kim, B., and Liang, P. (2020). Concept bottle-
neck models. In International Conference on Machine
Learning, pages 5338–5348. PMLR.
Li, J., Liao, S., Jiang, H., and Shao, L. (2020). Box Guided
Convolution for Pedestrian Detection. In 28th ACM
International Conference on Multimedia, pages 1615–
1624.
Li, J., Zhou, P., Xiong, C., and Hoi, S. C. H. (2021). Pro-
totypical contrastive learning of unsupervised repre-
sentations. In International Conference on Learning
Representations ICLR.
Lin, T.-Y., Goyal, P., Girshick, R., He, K., and Doll
´
ar, P.
(2017). Focal Loss for Dense Object Detection. In
Proceedings of the IEEE/CVF Conference on Com-
puter Vision and Pattern Recognition (CVPR), pages
2980–2988.
Liu, W., Liao, S., Ren, W., Hu, W., and Yu, Y. (2019). High-
level semantic feature detection: A new perspective
for pedestrian detection. In IEEE Conference on Com-
puter Vision and Pattern Recognition (CVPR), pages
5187–5196.
Meletis, P., Wen, X., Lu, C., de Geus, D., and Dubbelman,
G. (2020). Cityscapes-panoptic-parts and pascal-
panoptic-parts datasets for scene understanding. arXiv
preprint arXiv:2004.07944.
Newell, A., Yang, K., and Deng, J. (2016). Stacked hour-
glass networks for human pose estimation. In Euro-
pean conference on computer vision, pages 483–499.
Springer.
Peng, H. and Yu, S. (2021). Beyond softmax loss: Intra-
concentration and inter-separability loss for classifi-
cation. Neurocomputing, 438:155–164.
Rudin, C. (2019). Stop explaining black box machine learn-
ing models for high stakes decisions and use inter-
pretable models instead. Nature Machine Intelligence,
1(5):206–215.
Soviany, P., Ionescu, R. T., Rota, P., and Sebe, N. (2021).
Curriculum self-paced learning for cross-domain ob-
ject detection. Computer Vision and Image Under-
standing, 204:103166.
Tarvainen, A. and Valpola, H. (2017). Mean teachers are
better role models: Weight-averaged consistency tar-
gets improve semi-supervised deep learning results.
Advances in neural information processing systems,
30.
Xie, B., Li, S., Li, M., Liu, C. H., Huang, G., and Wang,
G. (2022). Sepico: Semantic-guided pixel contrast
for domain adaptive semantic segmentation. arXiv
preprint arXiv:2204.08808.
Yu, F., Wang, D., Shelhamer, E., and Darrell, T. (2018).
Deep layer aggregation. In IEEE Conference on Com-
puter Vision and Pattern Recognition (CVPR), pages
2403–2412.
Zhang, J., Lin, L., Zhu, J., Li, Y., Chen, Y.-c., Hu, Y., and
Hoi, C. S. (2020). Attribute-aware pedestrian detec-
tion in a crowd. IEEE Transactions on Multimedia.
Zhang, P., Zhang, B., Zhang, T., Chen, D., Wang, Y., and
Wen, F. (2021). Prototypical pseudo label denois-
ing and target structure learning for domain adap-
tive semantic segmentation. In Proceedings of the
IEEE/CVF conference on computer vision and pattern
recognition, pages 12414–12424.
Zhang, S., Benenson, R., and Schiele, B. (2017). Cityper-
sons: A diverse dataset for pedestrian detection. In
IEEE Conference on Computer Vision and Pattern
Recognition (CVPR), pages 3213–3221.
Domain Adaptive Pedestrian Detection Based on Semantic Concepts
659