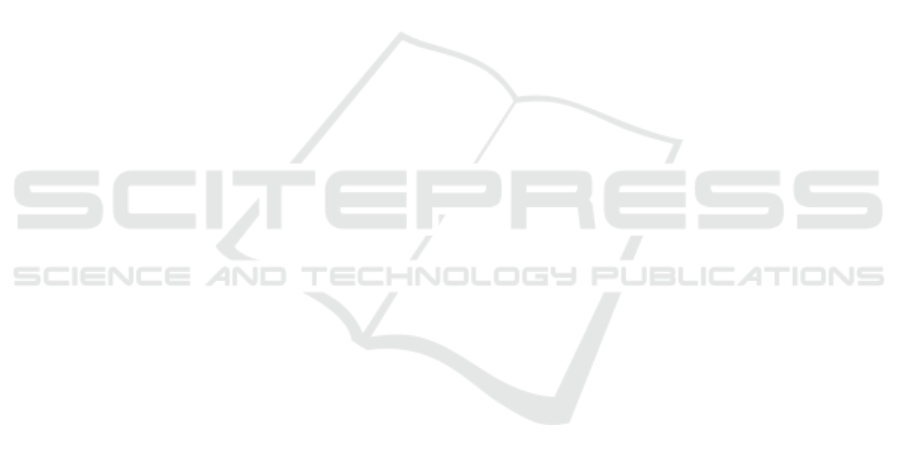
learning model for small molecules. arXiv preprint
arXiv:2203.04676.
Bagherian, M., Sabeti, E., Wang, K., Sartor, M. A.,
Nikolovska-Coleska, Z., and Najarian, K. (2021). Ma-
chine learning approaches and databases for predic-
tion of drug–target interaction: a survey paper. Brief-
ings in bioinformatics, 22(1):247–269.
Bardes, A., Ponce, J., and LeCun, Y. (2021). Vi-
creg: Variance-invariance-covariance regulariza-
tion for self-supervised learning. arXiv preprint
arXiv:2105.04906.
Bolg
´
ar, B. and Antal, P. (2017). Vb-mk-lmf: fusion
of drugs, targets and interactions using variational
bayesian multiple kernel logistic matrix factorization.
BMC bioinformatics, 18(1):1–18.
Chatterjee, A., Ahmed, O. S., Walters, R., Shafi, Z., Gysi,
D., Yu, R., Eliassi-Rad, T., Barab
´
asi, A.-L., and
Menichetti, G. (2021). Ai-bind: Improving binding
predictions for novel protein targets and ligands. arXiv
preprint arXiv:2112.13168.
Chen, J., Zhang, L., Cheng, K., Jin, B., Lu, X., and
Che, C. (2022). Predicting drug-target interaction via
self-supervised learning. IEEE/ACM Transactions on
Computational Biology and Bioinformatics.
Chen, T., Kornblith, S., Norouzi, M., and Hinton, G. (2020).
A simple framework for contrastive learning of visual
representations. In International conference on ma-
chine learning, pages 1597–1607. PMLR.
Collobert, R. and Weston, J. (2008). A unified architec-
ture for natural language processing: Deep neural net-
works with multitask learning. In Proceedings of the
25th international conference on Machine learning,
pages 160–167.
Gaulton, A., Hersey, A., Nowotka, M., Bento, A. P., Cham-
bers, J., Mendez, D., Mutowo, P., Atkinson, F., Bel-
lis, L. J., Cibri
´
an-Uhalte, E., et al. (2017). The
chembl database in 2017. Nucleic acids research,
45(D1):D945–D954.
Hadsell, R., Chopra, S., and LeCun, Y. (2006). Dimen-
sionality reduction by learning an invariant mapping.
In 2006 IEEE Computer Society Conference on Com-
puter Vision and Pattern Recognition (CVPR’06), vol-
ume 2, pages 1735–1742. IEEE.
Harrer, S., Shah, P., Antony, B., and Hu, J. (2019). Arti-
ficial intelligence for clinical trial design. Trends in
pharmacological sciences, 40(8):577–591.
Hsieh, C.-K., Yang, L., Cui, Y., Lin, T.-Y., Belongie, S.,
and Estrin, D. (2017). Collaborative metric learning.
In Proceedings of the 26th international conference on
world wide web, pages 193–201.
Jaeger, S., Fulle, S., and Turk, S. (2018). Mol2vec: un-
supervised machine learning approach with chemical
intuition. Journal of chemical information and mod-
eling, 58(1):27–35.
Khosla, P., Teterwak, P., Wang, C., Sarna, A., Tian, Y.,
Isola, P., Maschinot, A., Liu, C., and Krishnan, D.
(2020). Supervised contrastive learning. Advances
in Neural Information Processing Systems, 33:18661–
18673.
Le-Khac, P. H., Healy, G., and Smeaton, A. F. (2020). Con-
trastive representation learning: A framework and re-
view. IEEE Access, 8:193907–193934.
Lee, A. A., Brenner, M. P., and Colwell, L. J. (2016). Pre-
dicting protein–ligand affinity with a random matrix
framework. Proceedings of the National Academy of
Sciences, 113(48):13564–13569.
Li, Y., Qiao, G., Gao, X., and Wang, G. (2022). Supervised
graph co-contrastive learning for drug–target interac-
tion prediction. Bioinformatics, 38(10):2847–2854.
Lipscomb, C. E. (2000). Medical subject headings
(mesh). Bulletin of the Medical Library Association,
88(3):265.
Lu, A. X., Zhang, H., Ghassemi, M., and Moses, A.
(2020). Self-supervised contrastive learning of protein
representations by mutual information maximization.
BioRxiv.
Luo, H., Li, M., Yang, M., Wu, F.-X., Li, Y., and Wang,
J. (2021). Biomedical data and computational mod-
els for drug repositioning: a comprehensive review.
Briefings in bioinformatics, 22(2):1604–1619.
Musgrave, K., Belongie, S., and Lim, S.-N. (2020). Pytorch
metric learning. arXiv preprint arXiv:2008.09164.
¨
Ozt
¨
urk, H.,
¨
Ozg
¨
ur, A., and Ozkirimli, E. (2018). Deepdta:
deep drug–target binding affinity prediction. Bioinfor-
matics, 34(17):i821–i829.
Peska, L., Buza, K., and Koller, J. (2017). Drug-target inter-
action prediction: a bayesian ranking approach. Com-
puter methods and programs in biomedicine, 152:15–
21.
Pi
˜
nero, J., Bravo,
`
A., Queralt-Rosinach, N., Guti
´
errez-
Sacrist
´
an, A., Deu-Pons, J., Centeno, E., Garc
´
ıa-
Garc
´
ıa, J., Sanz, F., and Furlong, L. I. (2016). Dis-
genet: a comprehensive platform integrating informa-
tion on human disease-associated genes and variants.
Nucleic acids research, page gkw943.
Sun, Y., Cheng, C., Zhang, Y., Zhang, C., Zheng, L., Wang,
Z., and Wei, Y. (2020). Circle loss: A unified perspec-
tive of pair similarity optimization. In Proceedings
of the IEEE/CVF Conference on Computer Vision and
Pattern Recognition, pages 6398–6407.
Tang, J., Szwajda, A., Shakyawar, S., Xu, T., Hintsanen, P.,
Wennerberg, K., and Aittokallio, T. (2014). Making
sense of large-scale kinase inhibitor bioactivity data
sets: a comparative and integrative analysis. Journal
of Chemical Information and Modeling, 54(3):735–
743.
Weston, J., Bengio, S., and Usunier, N. (2010). Large scale
image annotation: learning to rank with joint word-
image embeddings. Machine learning, 81(1):21–35.
Wouters, O. J., McKee, M., and Luyten, J. (2020). Esti-
mated research and development investment needed
to bring a new medicine to market, 2009-2018. Jama,
323(9):844–853.
Zhang, S., Yao, L., Tay, Y., Xu, X., Zhang, X., and
Zhu, L. (2018). Metric factorization: Recommen-
dation beyond matrix factorization. arXiv preprint
arXiv:1802.04606.
DT-ML: Drug-Target Metric Learning
211