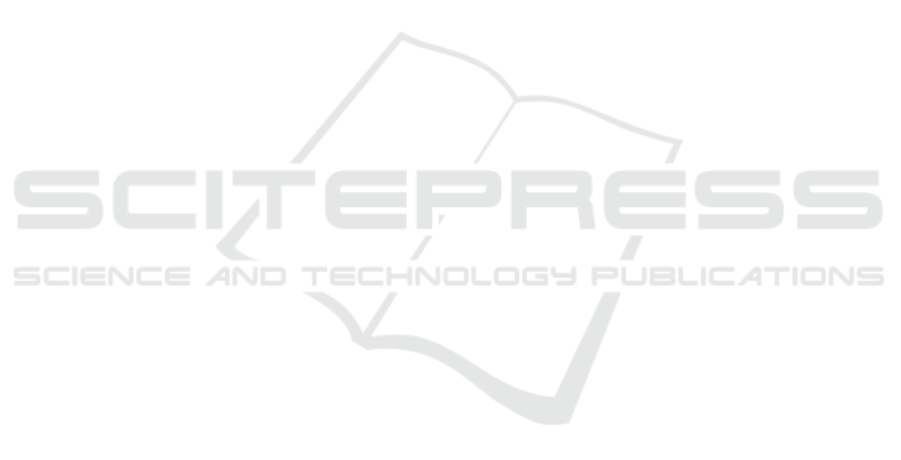
L., Santiago, C., Amato, G., Pizzorusso, T., and Gen-
naro, C. (2022a). Learning to count biological struc-
tures with raters’ uncertainty. Medical Image Analy-
sis, 80:102500.
Ciampi, L., Foszner, P., Messina, N., Staniszewski, M.,
Gennaro, C., Falchi, F., Serao, G., Cogiel, M., Golba,
D., Szcz˛esna, A., and Amato, G. (2022b). Bus vio-
lence: An open benchmark for video violence detec-
tion on public transport. Sensors, 22(21).
Ciampi, L., Gennaro, C., Carrara, F., Falchi, F., Vairo, C.,
and Amato, G. (2022c). Multi-camera vehicle count-
ing using edge-AI. Expert Systems with Applications,
207:117929.
Ciampi, L., Messina, N., Falchi, F., Gennaro, C., and Am-
ato, G. (2020). Virtual to real adaptation of pedestrian
detectors. Sensors, 20(18):5250.
Clavet, S. (2016). Motion matching and the road to next-
gen animation. In Proc. of GDC, volume 2016.
Contributors, M. (2020). MMTracking: OpenMMLab
video perception toolbox and benchmark. https://
github.com/open-mmlab/mmtracking.
Courty, N., Allain, P., Creusot, C., and Corpetti, T. (2014).
Using the agoraset dataset: Assessing for the quality
of crowd video analysis methods. Pattern Recognition
Letters, 44:161–170.
Dendorfer, P., Rezatofighi, H., Milan, A., Shi, J., Cremers,
D., Reid, I., Roth, S., Schindler, K., and Leal-Taixé, L.
(2020). Mot20: A benchmark for multi object track-
ing in crowded scenes. arXiv:2003.09003[cs]. arXiv:
2003.09003.
Foszner, P., Staniszewski, M., Szcz˛esna, A., Cogiel, M.,
Golba, D., Ciampi, L., Messina, N., Gennaro, C.,
Falchi, F., Amato, G., and Serao, G. (2022). Bus Vio-
lence: a large-scale benchmark for video violence de-
tection in public transport.
Holden, D., Habibie, I., Kusajima, I., and Komura, T.
(2017). Fast neural style transfer for motion data.
IEEE computer graphics and applications, 37(4):42–
49.
Holden, D., Kanoun, O., Perepichka, M., and Popa, T.
(2020). Learned motion matching. ACM Transactions
on Graphics (TOG), 39(4):53–1.
Khadka, A. R., Oghaz, M., Matta, W., Cosentino, M., Re-
magnino, P., and Argyriou, V. (2019). Learning how
to analyse crowd behaviour using synthetic data. In
Proceedings of the 32nd International Conference on
Computer Animation and Social Agents, pages 11–14.
Lemonari, M., Blanco, R., Charalambous, P., Pelechano,
N., Avraamides, M., Pettré, J., and Chrysanthou, Y.
(2022). Authoring virtual crowds: A survey. In Com-
puter Graphics Forum, volume 41, pages 677–701.
Wiley Online Library.
Lin, T.-Y., Goyal, P., Girshick, R., He, K., and Dollar, P.
(2017). Focal loss for dense object detection. In 2017
IEEE International Conference on Computer Vision
(ICCV). IEEE.
P˛eszor, D., Staniszewski, M., and Wojciechowska, M.
(2016). Facial reconstruction on the basis of video
surveillance system for the purpose of suspect iden-
tification. In Nguyen, N. T., Trawi
´
nski, B., Fujita,
H., and Hong, T.-P., editors, Intelligent Information
and Database Systems, pages 467–476, Berlin, Hei-
delberg. Springer Berlin Heidelberg.
Saeed, R. A., Recupero, D. R., and Remagnino, P. (2022).
Simulating crowd behaviour combining both micro-
scopic and macroscopic rules. Information Sciences,
583:137–158.
Sindagi, V., Yasarla, R., and Patel, V. M. (2020). Jhu-
crowd++: Large-scale crowd counting dataset and a
benchmark method. IEEE Transactions on Pattern
Analysis and Machine Intelligence.
Staniszewski, M., Foszner, P., Kostorz, K., Michalczuk,
A., Wereszczy
´
nski, K., Cogiel, M., Golba, D., Woj-
ciechowski, K., and Pola
´
nski, A. (2020). Application
of crowd simulations in the evaluation of tracking al-
gorithms. Sensors, 20(17):4960.
Staniszewski, M., Kloszczyk, M., Segen, J., Wereszczy
´
nski,
K., Drabik, A., and Kulbacki, M. (2016). Recent de-
velopments in tracking objects in a video sequence. In
Intelligent Information and Database Systems, pages
427–436. Springer Berlin Heidelberg.
Van Toll, W. and Pettré, J. (2021). Algorithms for micro-
scopic crowd simulation: Advancements in the 2010s.
In Computer Graphics Forum, volume 40, pages 731–
754. Wiley Online Library.
Wang, Q., Gao, J., Lin, W., and Li, X. (2020). Nwpu-crowd:
A large-scale benchmark for crowd counting and lo-
calization. IEEE transactions on pattern analysis and
machine intelligence, 43(6):2141–2149.
Wang, Q., Gao, J., Lin, W., and Yuan, Y. (2019). Learn-
ing from synthetic data for crowd counting in the
wild. In Proceedings of the IEEE/CVF conference on
computer vision and pattern recognition, pages 8198–
8207.
Wereszczy
´
nski, K., Michalczuk, A., Foszner, P., Golba, D.,
Cogiel, M., and Staniszewski, M. (2021). Elsa: Euler-
lagrange skeletal animations - novel and fast motion
model applicable to vr/ar devices. In Computational
Science – ICCS 2021, pages 120–133, Cham. Springer
International Publishing.
Wojke, N. and Bewley, A. (2018). Deep cosine metric learn-
ing for person re-identification. In 2018 IEEE Win-
ter Conference on Applications of Computer Vision
(WACV), pages 748–756. IEEE.
Wojke, N., Bewley, A., and Paulus, D. (2017). Simple on-
line and realtime tracking with a deep association met-
ric. In 2017 IEEE International Conference on Image
Processing (ICIP), pages 3645–3649. IEEE.
Yang, S., Li, T., Gong, X., Peng, B., and Hu, J. (2020). A
review on crowd simulation and modeling. Graphical
Models, 111:101081.
Yoon, Y., Kim, D. Y., Yoon, K., Song, Y., and Jeon,
M. (2019). Online multiple pedestrian tracking us-
ing deep temporal appearance matching association.
CoRR, abs/1907.00831.
Development of a Realistic Crowd Simulation Environment for Fine-Grained Validation of People Tracking Methods
229