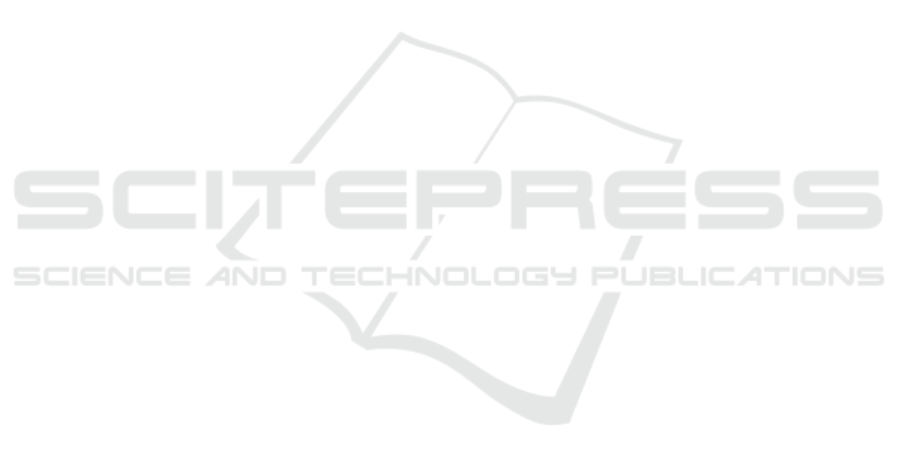
ing with deep learning.
REFERENCES
Ahmadi, M., Norouzi, A., Karimi, N., Samavi, S., and
Emami, A. (2020). Redmark: Framework for resid-
ual diffusion watermarking based on deep networks.
Expert Systems with Applications, 146:113157.
Arnab, A., Dehghani, M., Heigold, G., Sun, C., Lu
ˇ
ci
´
c, M.,
and Schmid, C. (2021). Vivit: A video vision trans-
former. In Proceedings of the IEEE/CVF Interna-
tional Conference on Computer Vision, pages 6836–
6846.
Bahrami, Z. and Akhlaghian Tab, F. (2018). A new robust
video watermarking algorithm based on surf features
and block classification. Multimedia Tools and Appli-
cations, 77(1):327–345.
Bayoudh, I., Ben Jabra, S., and Zagrouba, E. (2018).
Online multi-sprites based video watermarking ro-
bust to collusion and transcoding attacks for emerg-
ing applications. Multimedia Tools and Applications,
77(11):14361–14379.
Bayoudh, I., Jabra, S. B., and Zagrouba, E. (2015). On line
video watermarking-a new robust approach of video
watermarking based on dynamic multi-sprites genera-
tion. In VISAPP (3), pages 158–165.
Ben Jabra, S. and Zagrouba, E. (2021). Robust anaglyph 3d
video watermarking based on cyan mosaic generation
and dct insertion in krawtchouk moments. The Visual
Computer, pages 1–15.
Byrnes, O., La, W., Wang, H., Ma, C., Xue, M., and Wu, Q.
(2021). Data hiding with deep learning: A survey uni-
fying digital watermarking and steganography. arXiv
preprint arXiv:2107.09287.
Hou, J.-U. (2021). Mpeg and da-ad resilient dct-based video
watermarking using adaptive frame selection. Elec-
tronics, 10(20):2467.
Jamal, S. S., Khan, M. U., and Shah, T. (2016a). A wa-
termarking technique with chaotic fractional s-box
transformation. Wireless Personal Communications,
90(4):2033–2049.
Jamal, S. S., Khan, M. U., and Shah, T. (2016b). A
watermarking technique with chaotic fractional s-box
transformation. Wireless Personal Communications,
90(4):2033–2049.
Kandi, H., Mishra, D., and Gorthi, S. R. S. (2017). Explor-
ing the learning capabilities of convolutional neural
networks for robust image watermarking. Computers
& Security, 65:247–268.
Kerbiche, A., Jabra, S. B., Zagrouba, E., and Charvil-
lat, V. (2017). Robust video watermarking approach
based on crowdsourcing and hybrid insertion. In 2017
International Conference on Digital Image Comput-
ing: Techniques and Applications (DICTA), pages 1–
8. IEEE.
Kerbiche, A., Jabra, S. B., Zagrouba, E., and Charvillat, V.
(2018). A robust video watermarking based on feature
regions and crowdsourcing. Multimedia Tools and Ap-
plications, 77(20):26769–26791.
Koubaa, M., Elarbi, M., Ben Amar, C., and Nicolas, H.
(2012). Collusion, mpeg4 compression and frame
dropping resistant video watermarking. Multimedia
tools and applications, 56(2):281–301.
LeCun, Y., Bengio, Y., and Hinton, G. (2015). Deep learn-
ing. nature, 521(7553):436–444.
Lee, J.-E., Seo, Y.-H., and Kim, D.-W. (2020). Convolu-
tional neural network-based digital image watermark-
ing adaptive to the resolution of image and watermark.
Applied Sciences, 10(19):6854.
Li, Y., Wang, H., and Barni, M. (2021). A survey of deep
neural network watermarking techniques. Neurocom-
puting, 461:171–193.
Liu, Y., Guo, M., Zhang, J., Zhu, Y., and Xie, X. (2019).
A novel two-stage separable deep learning framework
for practical blind watermarking. In Proceedings of
the 27th ACM International Conference on Multime-
dia, pages 1509–1517.
Luo, X., Li, Y., Chang, H., Liu, C., Milanfar, P., and
Yang, F. (2021). Dvmark: A deep multiscale
framework for video watermarking. arXiv preprint
arXiv:2104.12734.
Mun, S.-M., Nam, S.-H., Jang, H., Kim, D., and Lee, H.-
K. (2019). Finding robust domain from attacks: A
learning framework for blind watermarking. Neuro-
computing, 337:191–202.
Raut, S. S. and Mune, A. (2017a). A review paper on digi-
tal watermarking techniques. International Journal of
Engineering Science, 10460.
Raut, S. S. and Mune, A. (2017b). A review paper on digi-
tal watermarking techniques. International Journal of
Engineering Science, 10460.
Sang, J., Liu, Q., and Song, C.-L. (2020). Robust video wa-
termarking using a hybrid dct-dwt approach. Journal
of Electronic Science and Technology, 18(2):100052.
Yang, L., Wang, H., Zhang, Y., Li, J., He, P., and Meng,
S. (2021). A robust dct-based video watermarking
scheme against recompression and synchronization
attacks. In International Workshop on Digital Water-
marking, pages 149–162. Springer.
Zhang, K. A., Xu, L., Cuesta-Infante, A., and Veeramacha-
neni, K. (2019). Robust invisible video watermarking
with attention. arXiv preprint arXiv:1909.01285.
Zhong, X., Huang, P.-C., Mastorakis, S., and Shih, F. Y.
(2020). An automated and robust image watermarking
scheme based on deep neural networks. IEEE Trans-
actions on Multimedia, 23:1951–1961.
Zhu, J., Kaplan, R., Johnson, J., and Fei-Fei, L. (2018).
Hidden: Hiding data with deep networks. In Proceed-
ings of the European conference on computer vision
(ECCV), pages 657–672.
A Robust Deep Learning-Based Video Watermarking Using Mosaic Generation
675