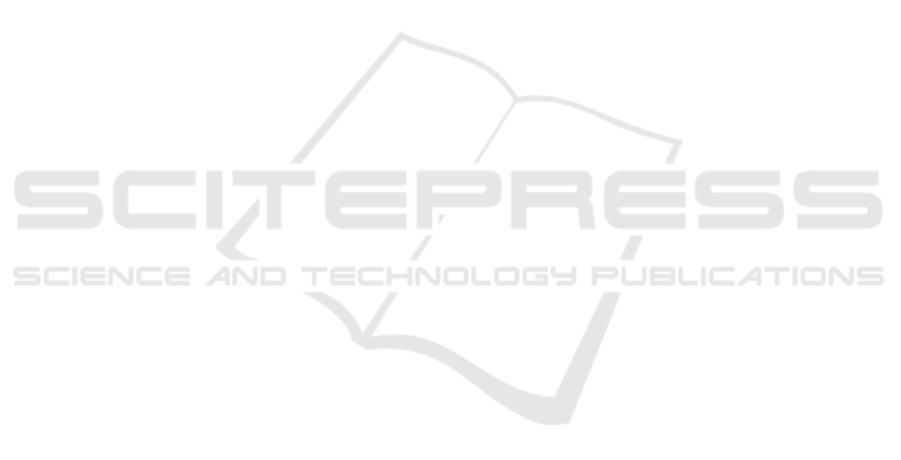
tient measurements in the sleep laboratory are already
planed. After obtaining these, we will add the final
step in the data processing chain, which is the auto-
matic diagnosis of the OSA stage. Due to the limited
number of patients in this trial study we have decided
not to include this stage, but rather focus on develop-
ing an efficient detection of respiratory events.
ACKNOWLEDGEMENTS
This work is funded through a research grant (No.: SE
3160/4-1 675251) from the German Research Foun-
dation (DFG).
REFERENCES
Abad, J., Mu
˜
noz-Ferrer, A., Prada, M., L
´
opez, C., Marin,
A., Mart
´
ınez-Rivera, C., Morera-Prat, J., and Ruiz-
Manzano, J. (2016). Automatic video analysis for ob-
structive sleep apnea syndrome diagnosis. Sleep, 39.
Akbarian, S., Montazeri Ghahjaverestan, N., Yadollahi, A.,
and Taati, B. (2020). Distinguishing obstructive ver-
sus central apneas in infrared video of sleep using
deep learning: Validation study. 22(5).
Berry, R., Quan, S., Abreu, A., and et al. (2020). . The
AASM Manual for the Scoring of Sleep and Associ-
ated Events: Rules, Terminology and Technical Spec-
ifications, Version 2.6. American Academy of Sleep
Medicine.
Cachada, N., Thomas, M., and Wharton, S. (2017). Com-
parison of manual and automatic scoring of limited
channel sleep studies:noxturnal software correlates
well with manual scoring in severe osa. page PA2301.
Cattani, L., Alinovi, D., Ferrari, G., Raheli, R., Pavlidis, E.,
Spagnoli, C., and Pisani, F. (2014). A wire-free, non-
invasive, low-cost video processing-based approach to
neonatal apnoea detection. pages 67–73.
Charlton, P. H., Bonnici, T., Tarassenko, L., Clifton, D. A.,
Beale, R., and Watkinson, P. J. (2016). An assessment
of algorithms to estimate respiratory rate from the
electrocardiogram and photoplethysmogram. Physi-
ological measurement, 37:610–626.
Dietrich, P., Heist, S., Landmann, M., K
¨
uhmstedt, P.,
and Notni, G. (2019). Bicos—an algorithm for fast
real-time correspondence search for statistical pattern
projection-based active stereo sensors. Applied Sci-
ences 2019, Vol. 9, Page 3330, 9:3330.
Gastel, M. V., Stuijk, S., Overeem, S., Dijk, J. P. V., Gilst,
M. M. V., and Haan, G. D. (2021). Camera-based vi-
tal signs monitoring during sleep - a proof of concept
study. IEEE journal of biomedical and health infor-
matics, 25:1409–1418.
Gederi, E. and Clifford, G. D. (2012). Fusion of image and
signal processing for the detection of obstructive sleep
apnea. pages 890–893.
Geertsema, E., Visser, G., Sander, L., and Kalitzin, S.
(2020). Automated non-contact detection of central
apneas using video. Biomedical Signal Processing
and Control, 55:101658.
Heinzer, R., Vat, S., Marques-Vidal, P., Marti-Soler, H., An-
dries, D., Tobback, N., Mooser, V., Preisig, M., Mal-
hotra, A., Waeber, G., Vollenweider, P., Tafti, M., and
Haba-Rubio, J. (2015). Prevalence of sleep-disordered
breathing in the general population: the hypnolaus
study. The Lancet. Respiratory medicine, 3:310–318.
Heist, S., Dietrich, P., Landmann, M., K
¨
uhmstedt, P., Notni,
G., and T
¨
unnermann, A. (2018). Gobo projection
for 3d measurements at highest frame rates: a perfor-
mance analysis. Light: Science and Applications 2018
7:1, 7:1–13.
Hu, M., Zhai, G., Li, D., Fan, Y., Duan, H., Zhu, W., and
Yang, X. (2018). Combination of near-infrared and
thermal imaging techniques for the remote and simul-
taneous measurements of breathing and heart rates un-
der sleep situation. Plos One.
Jain, A. (1997). Feature selection: evaluation, application,
and small sample performance. IEEE Transactions on
Pattern Analysis and Machine Intelligence, 19:153–
158.
Kapur, V. K., Auckley, D. H., Chowdhuri, S., Kuhlmann,
D. C., Mehra, R., Ramar, K., and Harrod, C. G.
(2017). Clinical practice guideline for diagnostic test-
ing for adult obstructive sleep apnea: An american
academy of sleep medicine clinical practice guideline.
Journal of Clinical Sleep Medicine, 13:479–504.
Karimi, M., Hedner, J., H
¨
abel, H., Nerman, O., and Grote,
L. (2015). Sleep apnea related risk of motor vehi-
cle accidents is reduced by continuous positive airway
pressure: Swedish traffic accident registry data. Sleep,
38:341–349.
Lorato, I., Stuijk, S., Meftah, M., Kommers, D., An-
driessen, P., van Pul, C., and de Haan, G. (2021). Au-
tomatic separation of respiratory flow from motion in
thermal videos for infant apnea detection. Sensors,
21(18).
Lugaresi, C., Tang, J., Nash, H., Mcclanahan, C., Uboweja,
E., Hays, M., Zhang, F., Chang, C.-L., Yong, M. G.,
Lee, J., Chang, W.-T., Hua, W., Georg, M., Grund-
mann, M., and Research, G. (2019). Mediapipe: A
framework for building perception pipelines.
Rundo, J. V. (2019). Obstructive sleep apnea basics. Cleve-
land Clinic journal of medicine, 86:2–9.
Scebba, G., Da Poian, G., and Karlen, W. (2021). Mul-
tispectral video fusion for non-contact monitoring of
respiratory rate and apnea. IEEE Transactions on
Biomedical Engineering, 68(1):350–359.
Veauthier, C., Ryczewski, J., Mansow-Model, S., Otte, K.,
Kayser, B., Glos, M., Sch
¨
obel, C., Paul, F., Brandt,
A., and Penzel, T. (2019). Contactless recording of
sleep apnea and periodic leg movements by nocturnal
3-d-video and subsequent visual perceptive comput-
ing. Scientific Reports, 9:16812.
Vogels, T., Gastel, M. V., Wang, W., and Haan, G. D.
(2018). Fully-automatic camera-based pulse-oximetry
during sleep. IEEE Computer Society Conference on
Contactless Optical Detection of Nocturnal Respiratory Events
343