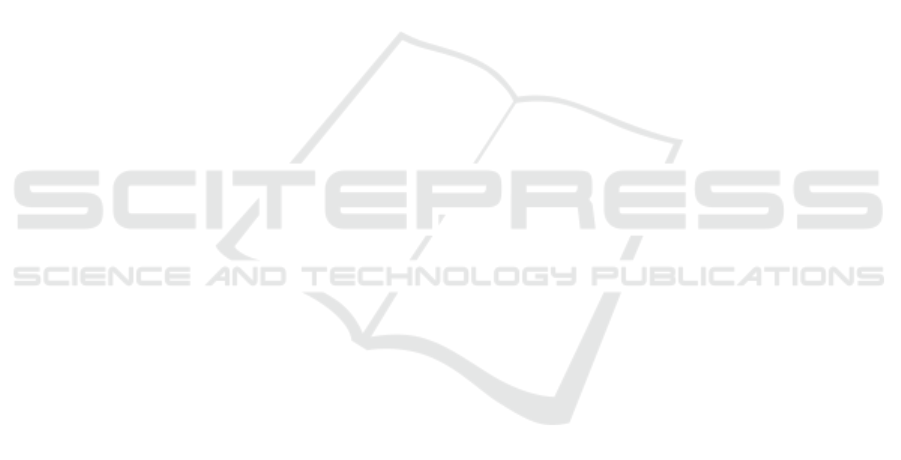
Fernando, K. R. M. and Tsokos, C. P. (2021). Dynamically
weighted balanced loss: class imbalanced learning
and confidence calibration of deep neural networks.
IEEE Transactions on Neural Networks and Learning
Systems.
Gonnet, P. and Deselaers, T. (2020). Indylstms: Indepen-
dently recurrent lstms. In IEEE International Con-
ference on Acoustics, Speech and Signal Processing
(ICASSP). IEEE.
Guellil, I., Sa
ˆ
adane, H., Azouaou, F., Gueni, B., and Nou-
vel, D. (2021). Arabic natural language process-
ing: An overview. Journal of King Saud University-
Computer and Information Sciences.
Hamdi, S., Hamdi, A., and Ben Yahia, S. (2022). Bert
and word embedding for interest mining of instagram
users. In Advances in Computational Collective Intel-
ligence, pages 123–136, Cham. Springer International
Publishing.
Huang, C., Li, Y., Loy, C. C., and Tang, X. (2016). Learn-
ing deep representation for imbalanced classification.
In Proceedings of the IEEE conference on computer
vision and pattern recognition.
Jafari, M., Li, R., Xing, Y., Auer, D., Francis, S., Garibaldi,
J., and Chen, X. (2019). Fu-net: multi-class image
segmentation using feedback weighted u-net. In In-
ternational Conference on Image and Graphics, pages
529–537. Springer.
Kaur, H., Pannu, H. S., and Malhi, A. K. (2019). A system-
atic review on imbalanced data challenges in machine
learning: Applications and solutions. ACM Comput-
ing Surveys (CSUR), 52(4):1–36.
Lin, T.-Y., Goyal, P., Girshick, R., He, K., and Doll
´
ar, P.
(2017). Focal loss for dense object detection. In
Proceedings of the IEEE international conference on
computer vision, pages 2980–2988.
Matthew, E. P., Neumann, M., Iyyer, M., Gardner, M.,
Clark, C., Kenton, L., and Zettlemoyer, L. (2018).
Deep contextualized word representations.
Oudah, M., Almahairi, A., and Habash, N. (2019). The
impact of preprocessing on arabic-english statistical
and neural machine translation.
Pontiki, M., Galanis, D., Papageorgiou, H., Androutsopou-
los, I., Manandhar, S., Al-Smadi, M., Al-Ayyoub,
M., Zhao, Y., Qin, B., De Clercq, O., et al. (2016a).
Semeval-2016 task 5: Aspect based sentiment anal-
ysis. In International workshop on semantic evalua-
tion, pages 19–30.
Pontiki, M., Galanis, D., Papageorgiou, H., Manandhar, S.,
and Androutsopoulos, I. (2016b). Semeval 2016 task
5: aspect based sentiment analysis (absa-16) annota-
tion guidelines.
Radford, A., Narasimhan, K., Salimans, T., and Sutskever,
I. (2018). Improving language understanding by gen-
erative pre-training.
Rengasamy, D., Jafari, M., Rothwell, B., Chen, X., and
Figueredo, G. P. (2020). Deep learning with dynamic
weighted loss function for sensor-based prognostics
and health management. Sensors.
Ruder, S., Ghaffari, P., and Breslin, J. G. (2016). Insight-1 at
semeval-2016 task 5: Deep learning for multilingual
aspect-based sentiment analysis.
Tamchyna, A. and Veselovsk
´
a, K. (2016). Ufal at semeval-
2016 task 5: recurrent neural networks for sentence
classification. In Proc. of the 10th international work-
shop on semantic evaluation.
Vaswani, A., Shazeer, N., Parmar, N., Uszkoreit, J., Jones,
L., Gomez, A. N., Kaiser, ., and Polosukhin, I. (2017).
Attention is all you need. Advances in neural infor-
mation processing systems.
ICAART 2023 - 15th International Conference on Agents and Artificial Intelligence
250