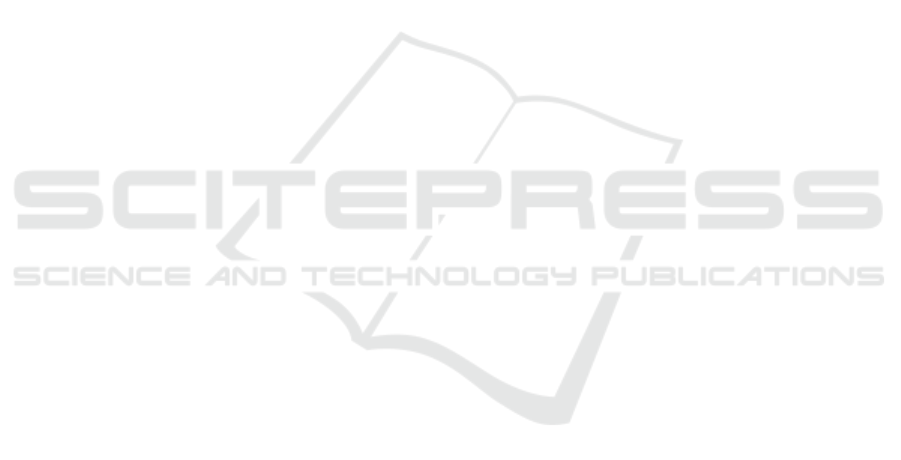
Fan, L., Zhang, T., and Du, W. (2021). Optical-flow-
based framework to boost video object detection per-
formance with object enhancement. Expert Systems
with Applications, 170:114544.
Geiger, A., Lenz, P., and Urtasun, R. (2012). Are we ready
for autonomous driving? the kitti vision benchmark
suite. In 2012 IEEE conference on computer vision
and pattern recognition, pages 3354–3361. IEEE.
Girshick, R., Donahue, J., Darrell, T., and Malik, J. (2014).
Rich feature hierarchies for accurate object detec-
tion and semantic segmentation. In Proceedings of
the IEEE conference on computer vision and pattern
recognition, pages 580–587.
Glegoła, W., Karpus, A., and Przybyłek, A. (2021). Mo-
bileNet family tailored for Raspberry Pi. Procedia
Computer Science, 192:2249–2258.
Gruosso, M., Capece, N., and Erra, U. (2021). Human
segmentation in surveillance video with deep learn-
ing. Multimedia Tools and Applications, 80(1):1175–
1199.
Haouari, F., Faraj, R., and AlJa’am, J. M. (2018). Fog
computing potentials, applications, and challenges. In
2018 International Conference on Computer and Ap-
plications (ICCA), pages 399–406. IEEE.
He, K., Zhang, X., Ren, S., and Sun, J. (2016). Deep resid-
ual learning for image recognition. In Proceedings of
the IEEE conference on computer vision and pattern
recognition, pages 770–778.
Hou, B., Liu, Y., Ling, N., Liu, L., and Ren, Y. (2021). A
Fast Lightweight 3D Separable Convolutional Neural
Network With Multi-Input Multi-Output for Moving
Object Detection. IEEE Access, 9:148433–148448.
Howard, A., Sandler, M., Chu, G., Chen, L.-C., Chen, B.,
Tan, M., Wang, W., Zhu, Y., Pang, R., Vasudevan, V.,
et al. (2019). Searching for mobilenetv3. In Pro-
ceedings of the IEEE/CVF International Conference
on Computer Vision, pages 1314–1324.
Howard, A. G., Zhu, M., Chen, B., Kalenichenko, D.,
Wang, W., Weyand, T., Andreetto, M., and Adam, H.
(2017). MobileNets: Efficient Convolutional Neural
Networks for Mobile Vision Applications.
Hung, M.-H., Pan, J.-S., and Hsieh, C.-H. (2014). A fast
algorithm of temporal median filter for background
subtraction. J. Inf. Hiding Multim. Signal Process.,
5(1):33–40.
Jagannathan, P., Rajkumar, S., Frnda, J., Divakarachari,
P. B., and Subramani, P. (2021). Moving Vehicle
Detection and Classification Using Gaussian Mix-
ture Model and Ensemble Deep Learning Technique.
Wireless Communications and Mobile Computing,
2021:1–15.
Krizhevsky, A., Sutskever, I., and Hinton, G. E. (2012). Im-
agenet classification with deep convolutional neural
networks. Advances in neural information processing
systems, 25.
Kulchandani, J. S. and Dangarwala, K. J. (2015). Moving
object detection: Review of recent research trends. In
2015 International Conference on Pervasive Comput-
ing (ICPC), pages 1–5. IEEE.
Lin, T.-Y., Goyal, P., Girshick, R., He, K., and Doll
´
ar, P.
(2017). Focal loss for dense object detection. In
Proceedings of the IEEE international conference on
computer vision, pages 2980–2988.
Lin, T.-Y., Maire, M., Belongie, S., Hays, J., Perona, P.,
Ramanan, D., Doll
´
ar, P., and Zitnick, C. L. (2014).
Microsoft coco: Common objects in context. In Euro-
pean conference on computer vision, pages 740–755.
Springer.
Liu, L., Ouyang, W., Wang, X., Fieguth, P., Chen, J., Liu,
X., and Pietik
¨
ainen, M. (2020). Deep Learning for
Generic Object Detection: A Survey. International
Journal of Computer Vision, 128(2):261–318.
Liu, W., Anguelov, D., Erhan, D., Szegedy, C., Reed, S.,
Fu, C.-Y., and Berg, A. C. (2016). Ssd: Single shot
multibox detector. In European conference on com-
puter vision, pages 21–37. Springer.
Machado, P., Oikonomou, A., Ferreira, J. F., and Mcginnity,
T. M. (2021). HSMD: An Object Motion Detection
Algorithm Using a Hybrid Spiking Neural Network
Architecture. IEEE Access, 9:125258–125268.
Patel, H. A. and Thakore, D. G. (2013). Moving object
tracking using kalman filter. International Journal of
Computer Science and Mobile Computing, 2(4):326–
332.
Redmon, J., Divvala, S., Girshick, R., and Farhadi, A.
(2016). You only look once: Unified, real-time object
detection. In Proceedings of the IEEE conference on
computer vision and pattern recognition, pages 779–
788.
Ren, S., He, K., Girshick, R., and Sun, J. (2015). Faster
r-cnn: Towards real-time object detection with region
proposal networks. Advances in neural information
processing systems, 28.
Russakovsky, O., Deng, J., Su, H., Krause, J., Satheesh, S.,
Ma, S., Huang, Z., Karpathy, A., Khosla, A., Bern-
stein, M., et al. (2015). Imagenet large scale visual
recognition challenge. International journal of com-
puter vision, 115(3):211–252.
Simonyan, K. and Zisserman, A. (2014). Very deep con-
volutional networks for large-scale image recognition.
arXiv preprint arXiv:1409.1556.
Song, Z., Ali, S., and Bouguila, N. (2020). Background
subtraction using infinite asymmetric gaussian mix-
ture models with simultaneous feature selection. IET
Image Processing, 14(11):2321–2332.
Suzuki, S. et al. (1985). Topological structural analy-
sis of digitized binary images by border following.
Computer vision, graphics, and image processing,
30(1):32–46.
Tan, M. and Le, Q. (2019). Efficientnet: Rethinking model
scaling for convolutional neural networks. In Interna-
tional conference on machine learning, pages 6105–
6114. PMLR.
Tayeb, A. A., Aldhaheri, R. W., and Hanif, M. S.
(2021). Vehicle speed estimation using gaussian mix-
ture model and kalman filter. International Journal of
Computers, Communications and Control, 16(4).
Xie, S., Girshick, R., Doll
´
ar, P., Tu, Z., and He, K. (2017).
Aggregated residual transformations for deep neural
A Lightweight Gaussian-Based Model for Fast Detection and Classification of Moving Objects
183