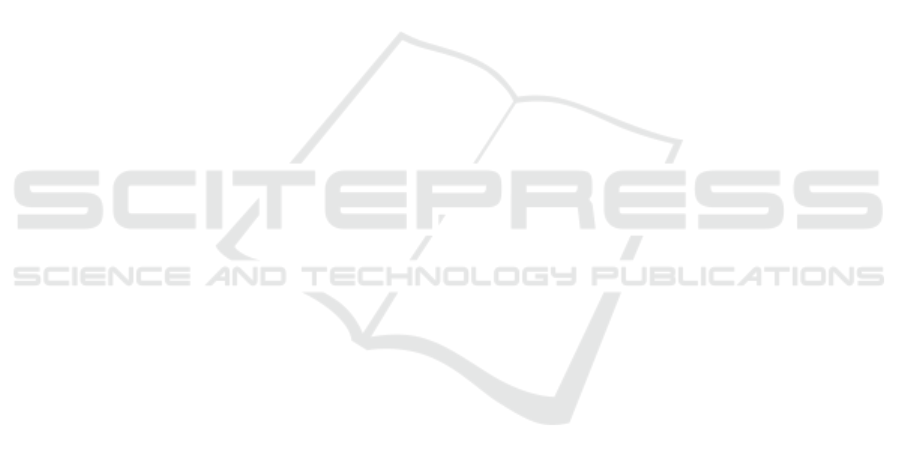
6 CONCLUSION & FUTURE
WORK
We propose a novel approach to forecast time-to-
accident (TTA) by leveraging Spatio-temporal fea-
tures extracted from traffic accident videos. Our ap-
proach uses inexpensive and easy-to-install dashboard
cameras as opposed to expensive depth imaging de-
vices or sensors that require experts for installation.
This can allow for easy integration with any vehicle
and can be used as a collision avoidance tool. Our
approach only uses the first N-frames where N is at
most 10 (1 second), this allows the driver enough time
to take action to mitigate the risks given the predic-
tion horizon is between 3-6 seconds. Additionally, we
present an efficient 3D CNN architecture with signif-
icantly fewer parameters compared to state-of-the-art
3D CNN architectures (e.g., C3D) without compro-
mising performance. This can enable our approach
to be implemented in real-time scenarios where min-
imum inference latency, low computational cost, and
high accuracy are necessary. Comparing the results
of our multi-frame experiments against the single-
frame experiments there is clear evidence that Spatio-
temporal features perform better as opposed to using
only spatial features. Apart from estimating TTA, our
model can also recognize accident and non-accident
scenes with 100% accuracy. This can be beneficial for
avoiding false alarms in real-time applications. We
also notice that there is no clear monotonic relation-
ship between temporal depth and prediction error, our
findings align with other studies in the literature as
mentioned in the previous section. Apart from the
temporal depth our experiments suggest that spatial
resolution impacts the predicted outcome. As a part
of future work, we will work on the interpretability
of our model to analyze the features that impact the
prediction error. We also plan to integrate our model
with an accident localization framework to detect var-
ious road users that pose a collision threat. Further-
more, we will implement our approach in real-world
scenarios and assess the feasibility of our solution in
real-time.
REFERENCES
Bahmei, B., Birmingham, E., and Arzanpour, S. (2022).
Cnn-rnn and data augmentation using deep convolu-
tional generative adversarial network for environmen-
tal sound classification. IEEE Signal Processing Let-
ters, 29:682–686.
Bao, W., Yu, Q., and Kong, Y. (2020). Uncertainty-based
traffic accident anticipation with spatio-temporal rela-
tional learning. In Proceedings of the 28th ACM In-
ternational Conference on Multimedia, pages 2682–
2690.
Bewley, A., Ge, Z., Ott, L., Ramos, F., and Upcroft, B.
(2016). Simple online and realtime tracking. CoRR,
abs/1602.00763.
Chan, F.-H., Chen, Y.-T., Xiang, Y., and Sun, M. (2016).
Anticipating accidents in dashcam videos. In Asian
Conference on Computer Vision, pages 136–153.
Springer.
Gaurav, R., Tripp, B., and Narayan, A. (2021). Driving
scene understanding: How much temporal context and
spatial resolution is necessary? In Canadian Confer-
ence on AI.
Hayward, J. C. (1972). Near miss determination through
use of a scale of danger.
Jim
´
enez, F., Naranjo, J. E., and Garc
´
ıa, F. (2013). An
improved method to calculate the time-to-collision
of two vehicles. International Journal of Intelligent
Transportation Systems Research, 11(1):34–42.
Kayukawa, S., Higuchi, K., Guerreiro, J., Morishima, S.,
Sato, Y., Kitani, K., and Asakawa, C. (2019). Bbeep:
A sonic collision avoidance system for blind travellers
and nearby pedestrians. In Proceedings of the 2019
CHI Conference on Human Factors in Computing Sys-
tems, pages 1–12.
Loquercio, A., Maqueda, A. I., Del-Blanco, C. R., and
Scaramuzza, D. (2018). Dronet: Learning to fly
by driving. IEEE Robotics and Automation Letters,
3(2):1088–1095.
Manglik, A., Weng, X., Ohn-Bar, E., and Kitanil, K. M.
(2019). Forecasting time-to-collision from monoc-
ular video: Feasibility, dataset, and challenges. In
2019 IEEE/RSJ International Conference on Intelli-
gent Robots and Systems (IROS), pages 8081–8088.
IEEE.
Saffarzadeh, M., Nadimi, N., Naseralavi, S., and Mam-
doohi, A. R. (2013). A general formulation for time-
to-collision safety indicator. In Proceedings of the
Institution of Civil Engineers-Transport, volume 166,
pages 294–304. Thomas Telford Ltd.
Sharma, S., Ansari, J. A., Murthy, J. K., and Krishna, K. M.
(2018). Beyond pixels: Leveraging geometry and
shape cues for online multi-object tracking. CoRR,
abs/1802.09298.
Suzuki, T., Kataoka, H., Aoki, Y., and Satoh, Y. (2018).
Anticipating traffic accidents with adaptive loss and
large-scale incident db. In Proceedings of the IEEE
conference on computer vision and pattern recogni-
tion, pages 3521–3529.
The Insurance Institute, H. S. (2022). Real-world benefits
of crash avoidance technologies.
Tøttrup, D., Skovgaard, S. L., Sejersen, J. l. F., and Pi-
mentel de Figueiredo, R. (2022). A real-time method
for time-to-collision estimation from aerial images.
Journal of Imaging, 8(3):62.
Tran, D., Bourdev, L., Fergus, R., Torresani, L., and Paluri,
M. (2015). Learning spatiotemporal features with 3d
convolutional networks. In Proceedings of the IEEE
international conference on computer vision, pages
4489–4497.
Learning Spatio-Temporal Features via 3D CNNs to Forecast Time-to-Accident
539