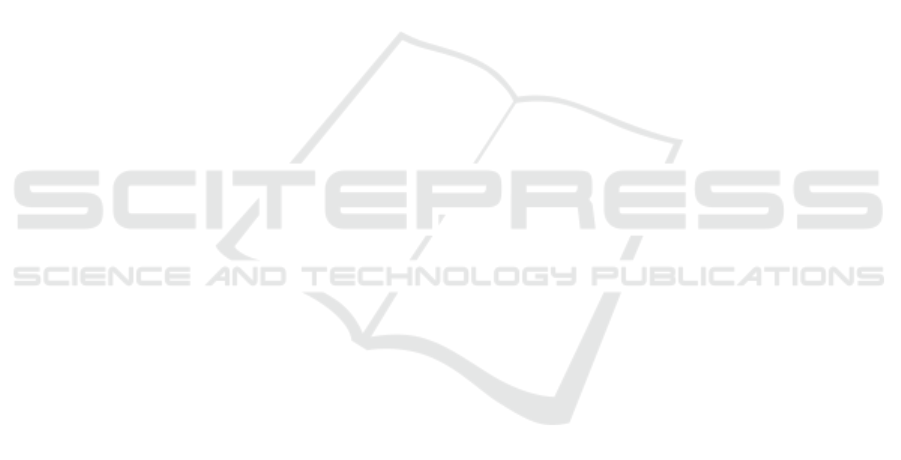
ETHICS DECLARATIONS
All volunteers gave their informed written consent
following approval and in accordance with the CER-
SU Review Board.
REFERENCES
Abe, T., Nonomura, T., Komada, Y., Asaoka, S., Sasai, T.,
Ueno, A., and Inoue, Y. (2011). Detecting deteriorated
vigilance using percentage of eyelid closure time dur-
ing behavioral maintenance of wakefulness tests. In-
ternational Journal of Psychophysiology, 82(3):269–
274.
Allen, J. (2007). Photoplethysmography and its application
in clinical physiological measurement. Physiological
measurement, 28(3):R1.
Arnal, P. J., Thorey, V., Ballard, M. E., Hernandez, A. B.,
Guillot, A., Jourde, H., Harris, M., Guillard, M.,
Van Beers, P., Chennaoui, M., et al. (2019). The
dreem headband as an alternative to polysomnography
for eeg signal acquisition and sleep staging. BioRxiv,
page 662734.
Board, S. (1999). Evaluation of us department of transporta-
tion efforts in the 1990s to address operator fatigue.
Safety Report NTSB/SR-99/01, Washington, DC.
Cardiosport (2022). Cardiosport TP5 device descrip-
tion. https://www.cardiosport.com/cardiosport-heart-
rate-monitors. Accessed: 2022-09-23.
Chai, M. et al. (2019). Drowsiness monitoring based on
steering wheel status. Transportation research part
D: transport and environment, 66:95–103.
Cruz-Neira, C., Sandin, D. J., and DeFanti, T. A. (1993).
Surround-screen projection-based virtual reality: the
design and implementation of the cave. In Pro-
ceedings of the 20th annual conference on Computer
graphics and interactive techniques, pages 135–142.
de Naurois, C. J., Bourdin, C., Stratulat, A., Diaz, E., and
Vercher, J.-L. (2019). Detection and prediction of
driver drowsiness using artificial neural network mod-
els. Accident Analysis & Prevention, 126:95–104.
EuropeanCommission (2021). European Com-
mission driver drowsiness and attention
warning. https://eur-lex.europa.eu/legal-
content/EN/ALL/?uri=CELEX:32021R1341. Ac-
cessed: 2022-09-23.
Friedrichs, F. and Yang, B. (2010). Drowsiness monitor-
ing by steering and lane data based features under
real driving conditions. In 2010 18th European Sig-
nal Processing Conference, pages 209–213. IEEE.
Garmin (2022). Garmin Venu SQ device description.
https://www.garmin.com/en-US/p/707174. Accessed:
2022-09-23.
Hori, C., Watanabe, S., Hori, T., Harsham, B. A., Hershey,
J., Koji, Y., Fujii, Y., and Furumoto, Y. (2016). Driver
confusion status detection using recurrent neural net-
works. In 2016 IEEE International Conference on
Multimedia and Expo (ICME), pages 1–6. IEEE.
Kaida, K., Takahashi, M.,
˚
Akerstedt, T., Nakata, A., Otsuka,
Y., Haratani, T., and Fukasawa, K. (2006). Valida-
tion of the karolinska sleepiness scale against perfor-
mance and eeg variables. Clinical neurophysiology,
117(7):1574–1581.
Kim, I.-H., Bong, J.-H., Park, J., and Park, S. (2017). Pre-
diction of driver’s intention of lane change by aug-
menting sensor information using machine learning
techniques. Sensors, 17(6):1350.
McKinley, R. A., McIntire, L. K., Schmidt, R., Repperger,
D. W., and Caldwell, J. A. (2011). Evaluation of
eye metrics as a detector of fatigue. Human factors,
53(4):403–414.
Sahayadhas, A., Sundaraj, K., and Murugappan, M. (2012).
Detecting driver drowsiness based on sensors: a re-
view. Sensors, 12(12):16937–16953.
Zontone, P., Antonio, A., Bernardini, R., Linz, L. D., Pi-
ras, A., and Rinaldo, R. (2020). Supervised Learning
Techniques for Stress Detection in Car Drivers. Ad-
vances in Science, Technology and Engineering Sys-
tems Journal, 5(6):22–29.
Physiological Data Recording in VR Simulator for Sleepiness Detection During Driving
415