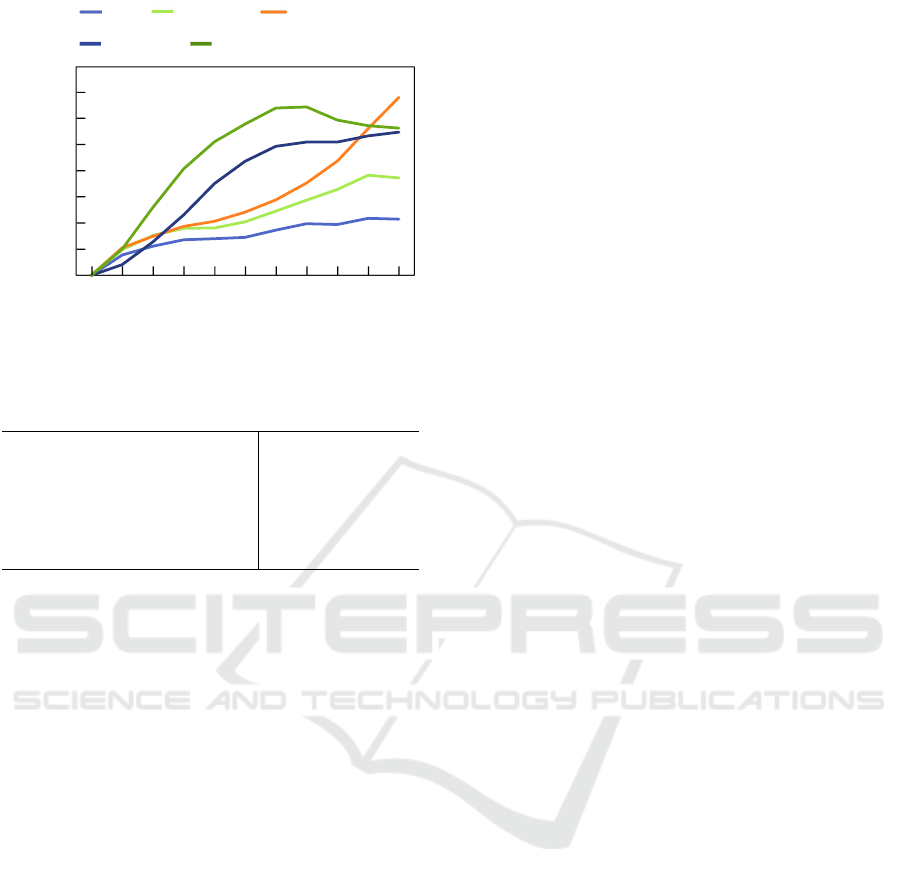
0
0.02
0.04
0.06
0.08
0.1
0.12
0.14
0.16
0 0.2 0.4 0.6 0.8 1
confidence
Percentage of inser�on
Human knowledge
ABN
ABN + AMB
ABN + COT ABN + COMB
Figure 9: Insertion metrics on CUB-200-2010 dataset.
Table 4: Area Under Curve for Insertion metrics on CUB-
200-2010 dataset. Bold letters indicate the highest score.
model AUC
ABN 0.0302
ABN + AMB 0.0451
Human knowledge 0.0576
ABN + COT 0.0704
ABN + COMB 0.0921
tention map. In this experiment, we used only sam-
ples that ABN misclassified to evaluate misclassifica-
tion improvements. Figure 9 and Table 4 show the
results of insertion for each dataset. Table 4 shows
that the AUC of the proposed method was higher than
that of ABN and ABN + AMB, human knowledge-
based fine-tuning. These results demonstrate that the
proposed method can optimize the attention map.
5 CONCLUSION
In this paper, we investigated the relationship between
the attention area outside the recognition target and
the incorrect answer class probability and proposed a
method to optimize an attention map by introducing
Complement Objective Training (COT) into the at-
tention branch network (ABN) and attention mining
branch (AMB). Our experiments showed that the pro-
posed method improved both the attention area and
the recognition accuracy. Further, evaluation with in-
sertion metrics demonstrated that the attention map
obtained by the proposed method could capture the
effective region for recognition. Our future work will
apply this technology to segmentation and multitask-
ing.
ACKNOWLEDGEMENTS
This paper is based on results obtained from a
project, JPNP20006, commissioned by the New En-
ergy and Industrial Technology Development Organi-
zation (NEDO).
REFERENCES
Alex, K., S. I. and Hinton, G. E. (2012). Paper templates.
In ImageNet Classification with Deep Convolutional
Neural Networks. SCITEPRESS.
Bojarski, M., Choromanska, A., Choromanski, K., Firner,
B., Jackel, L., Muller, U., and Zieba, K. (2016). Vi-
sualbackprop: efficient visualization of cnns. arXiv
preprint arXiv:1611.05418.
Chattopadhay, A., Sarkar, A., Howlader, P., and Balasub-
ramanian, V. N. (2018). Grad-cam++: Generalized
gradient-based visual explanations for deep convolu-
tional networks. In 2018 IEEE winter conference on
applications of computer vision (WACV), pages 839–
847. IEEE.
Chen, H.-Y., Wang, P.-H., Liu, C.-H., Chang, S.-C.,
Pan, J.-Y., Chen, Y.-T., Wei, W., and Juan, D.-
C. (2019). Complement objective training. arXiv
preprint arXiv:1903.01182.
Fong, R., Patrick, M., and Vedaldi, A. (2019). Under-
standing deep networks via extremal perturbations
and smooth masks. In Proceedings of the IEEE/CVF
International Conference on Computer Vision (ICCV).
Fong, R. C. and Vedaldi, A. (2017). Interpretable explana-
tions of black boxes by meaningful perturbation. In
Proceedings of the IEEE international conference on
computer vision, pages 3429–3437.
Fukui, H., Hirakawa, T., Yamashita, T., and Fujiyoshi, H.
(2019). Attention branch network: Learning of atten-
tion mechanism for visual explanation. In Proceed-
ings of the IEEE/CVF conference on computer vision
and pattern recognition, pages 10705–10714.
He, K., Zhang, X., Ren, S., and Sun, J. (2016). Deep resid-
ual learning for image recognition. In Proceedings of
the IEEE conference on computer vision and pattern
recognition, pages 770–778.
Iwayoshi, T., Mitsuhara, M., Takada, M., Hirakawa, T., Ya-
mashita, T., and Fujiyoshi, H. (2021). Attention min-
ing branch for optimizing attention map. In 2021 17th
International Conference on Machine Vision and Ap-
plications (MVA), pages 1–5. IEEE.
Khosla, A., Jayadevaprakash, N., Yao, B., and Li, F.-F.
(2011). Novel dataset for fine-grained image catego-
rization: Stanford dogs. In Proc. CVPR workshop on
fine-grained visual categorization (FGVC), volume 2.
Citeseer.
Lin, M., Chen, Q., and Yan, S. (2013). Network in network.
arXiv preprint arXiv:1312.4400.
Mitsuhara, M., Fukui, H., Sakashita, Y., Ogata, T., Hi-
rakawa, T., Yamashita, T., and Fujiyoshi, H. (2021).
Embedding human knowledge in deep neural network
VISAPP 2023 - 18th International Conference on Computer Vision Theory and Applications
112