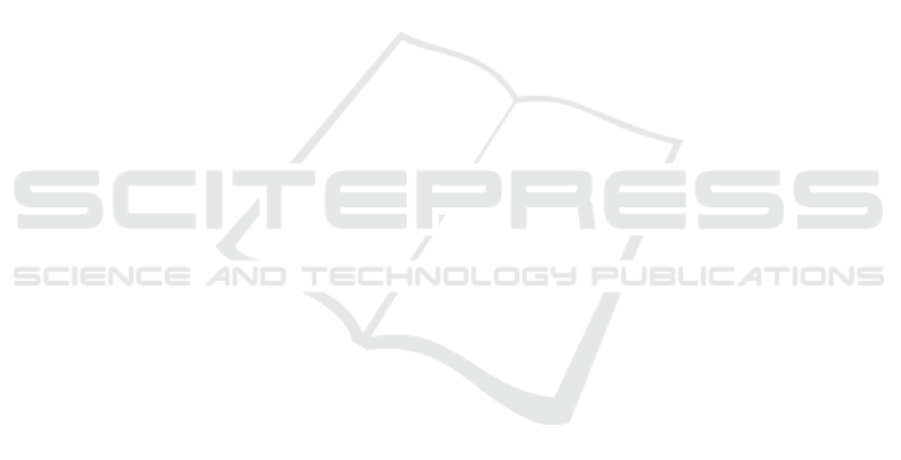
5.2 Future Research
Scripts have different characteristics, possibly re-
sulting in differing distributions of extracted fea-
tures. Likewise, individual features capture varying
attributes of handwriting. Therefore, temporal infor-
mation on handwriting styles might follow different
trends across various features. While linear SVMs
performed well on the MPS data set for the features
used in the current research, these potential differ-
ences in distributions were not considered. This could
lead to a decrease in performance for models trained
on augmented data. Hence, other kernels should be
studied to obtain optimal models for individual fea-
tures and scripts.
One of the risks with historical manuscript dating
is that the majority of the samples from a period, or a
year, originate from one writer. Rather than learning
to distinguish between characteristics in handwriting
styles specific to a particular period or year, models
would learn traits specific to writers for these years.
Data augmented to simulate variability between writ-
ers within time periods might lead to more robust
models than when data is augmented to simulate a re-
alistic within-writer variability.
As mentioned in Section 2, deep learning ap-
proaches outperformed statistical approaches on the
MPS data set. Considering this, it would be in-
teresting to investigate whether data augmentation
might positively affect historical manuscript dating
on smaller and heavier degraded manuscripts, such as
the EAA collections. Moreover, using the shape evo-
lution of individual characters with grapheme-based
statistical features might bypass the issue of limited
data and loss of information due to the resizing of im-
ages.
ACKNOWLEDGEMENT
The study for this article collaborated with several re-
search outcomes from the European Research Coun-
cil (EU Horizon 2020) project: The Hands that Wrote
the Bible: Digital Palaeography and Scribal Culture
of the Dead Sea Scrolls (HandsandBible 640497),
principal investigator: Mladen Popovi
´
c. Furthermore,
for the high-resolution, multi-spectral images of the
Dead Sea Scrolls, we are grateful to the Israel An-
tiquities Authority (IAA), courtesy of the Leon Levy
Dead Sea Scrolls Digital Library; photographer: Shai
Halevi. Additionally, we express our gratitude to the
Bodleian Libraries, University of Oxford, the Khalili
collections, and the Staatliche Museen zu Berlin (pho-
tographer: Sandra Steib) for the early Aramaic im-
ages. We also thank Petros Samara for collecting the
Medieval Paleographical Scale (MPS) dataset for the
Dutch NWO project. Finally, we thank the Center for
Information Technology of the University of Gronin-
gen for their support and for providing access to the
Peregrine high-performance computing cluster.
REFERENCES
Bulacu, M., Brink, A., Van Der Zant, T., and Schomaker, L.
(2009a). Recognition of handwritten numerical fields
in a large single-writer historical collection. In 2009
10th international conference on document analysis
and recognition, pages 808–812. IEEE.
Bulacu, M., Brink, A., Zant, T., and Schomaker, L. (2009b).
Recognition of handwritten numerical fields in a large
single-writer historical collection. pages 808–812.
Note: this is a peer-reviewed conference paper on
an important international conference series, ICDAR;
2009 10th International Conference on Document
Analysis and Recognition ; Conference date: 26-07-
2009 Through 29-07-2009.
Bulacu, M. and Schomaker, L. (2007). Text-independent
writer identification and verification using textural and
allographic features. IEEE Transactions on Pattern
Analysis and Machine Intelligence, 29(4):701–717.
Cuevas, A., Febrero, M., and Fraiman, R. (2004). An anova
test for functional data. Computational statistics &
data analysis, 47(1):111–122.
Dhali, M., Wit, J., and Schomaker, L. (2019). Binet:
Degraded-manuscript binarization in diverse docu-
ment textures and layouts using deep encoder-decoder
networks. ArXiv. 26 pages, 15 figures, 11 tables.
Dhali, M. A., Jansen, C. N., de Wit, J. W., and Schomaker,
L. (2020). Feature-extraction methods for histori-
cal manuscript dating based on writing style develop-
ment. Pattern Recognition Letters, 131:413–420.
Geng, X., Zhou, Z.-H., and Smith-Miles, K. (2007). Au-
tomatic age estimation based on facial aging patterns.
IEEE Transactions on Pattern Analysis and Machine
Intelligence, 29(12):2234–2240.
Hamid, A., Bibi, M., Moetesum, M., and Siddiqi, I.
(2019). Deep learning based approach for histori-
cal manuscript dating. In 2019 International Con-
ference on Document Analysis and Recognition (IC-
DAR), pages 967–972.
He, S., Samara, P., Burgers, J., and Schomaker, L. (2014).
Towards style-based dating of historical documents.
In 14th International Conference on Frontiers in
Handwritten Recognition. IEEE. 14th International
Conference on Frontiers in Handwriting Recognition
; Conference date: 01-09-2014 Through 04-09-2014.
He, S., Samara, P., Burgers, J., and Schomaker, L. (2016a).
Historical manuscript dating based on temporal pat-
tern codebook. Computer Vision and Image Under-
standing, 152:167–175.
He, S., Samara, P., Burgers, J., and Schomaker, L. (2016b).
Image-based historical manuscript dating using con-
ICPRAM 2023 - 12th International Conference on Pattern Recognition Applications and Methods
134