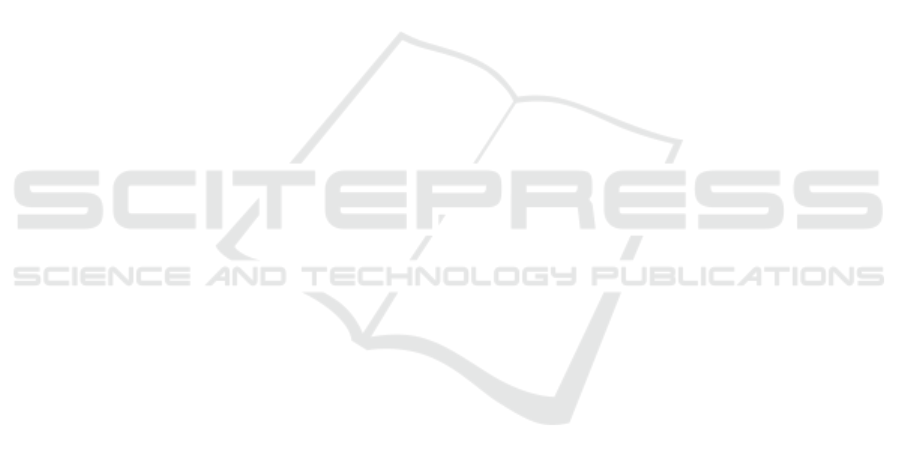
for medieval manuscripts. In 2019 International Con-
ference on Document Analysis and Recognition (IC-
DAR), pages 1200–1206. IEEE.
Archibald, T., Poggemann, M., Chan, A., and Martinez, T.
(2021). Trace: A differentiable approach to line-level
stroke recovery for offline handwritten text. arXiv
preprint arXiv:2105.11559.
Baek, Y., Lee, B., Han, D., Yun, S., and Lee, H. (2019).
Character region awareness for text detection. In Pro-
ceedings of the IEEE/CVF Conference on Computer
Vision and Pattern Recognition, pages 9365–9374.
Barakat, B. K., El-Sana, J., and Rabaev, I. (2019). The
pinkas dataset. In 2019 International Conference on
Document Analysis and Recognition (ICDAR), pages
732–737. IEEE.
Berndt, D. J. and Clifford, J. (1994). Using dynamic time
warping to find patterns in time series. In KDD work-
shop, volume 10, pages 359–370. Seattle, WA, USA:.
Bhunia, A. K., Bhowmick, A., Bhunia, A. K., Konwer, A.,
Banerjee, P., Roy, P. P., and Pal, U. (2018). Hand-
writing trajectory recovery using end-to-end deep
encoder-decoder network. In 2018 24th International
Conference on Pattern Recognition (ICPR), pages
3639–3644. IEEE.
Bochkovskiy, A., Wang, C.-Y., and Liao, H.-Y. M. (2020).
Yolov4: Optimal speed and accuracy of object detec-
tion. arXiv preprint arXiv:2004.10934.
Boillet, M., Kermorvant, C., and Paquet, T. (2021). Mul-
tiple document datasets pre-training improves text
line detection with deep neural networks. In 2020
25th International Conference on Pattern Recognition
(ICPR), pages 2134–2141. IEEE.
Demır, A. A.,
¨
OzS¸eker,
˙
I., and
¨
Ozkaya, U. (2021). Text line
segmentation in handwritten documents with genera-
tive adversarial networks. In 2021 International Con-
ference on INnovations in Intelligent SysTems and Ap-
plications (INISTA), pages 1–5. IEEE.
Diaz, M., Crispo, G., Parziale, A., Marcelli, A., and Ferrer,
M. A. (2022). Writing order recovery in complex and
long static handwriting.
Faundez-Zanuy, M., Fierrez, J., Ferrer, M. A., Diaz, M.,
Tolosana, R., and Plamondon, R. (2020). Hand-
writing biometrics: Applications and future trends
in e-security and e-health. Cognitive Computation,
12(5):940–953.
Gader, T. B. A. and Echi, A. K. (2020). Unconstrained
handwritten arabic text-lines segmentation based on
ar2u-net. In 2020 17th International Conference on
Frontiers in Handwriting Recognition (ICFHR), pages
349–354. IEEE.
Gader, T. B. A. and Echi, A. K. (2022). Deep learning-
based segmentation of connected components in ara-
bic handwritten documents. In International Confer-
ence on Intelligent Systems and Pattern Recognition,
pages 93–106. Springer.
Guyon, I., Schomaker, L., Plamondon, R., Liberman, M.,
and Janet, S. (1994). Unipen project of on-line data
exchange and recognizer benchmarks. In Proceed-
ings of the 12th IAPR International Conference on
Pattern Recognition, Vol. 3-Conference C: Signal Pro-
cessing (Cat. No. 94CH3440-5), volume 2, pages 29–
33. IEEE.
Hanif, S. and Latecki, L. J. Autonomous character region
score fusion for word detection in camera-captured
handwriting documents.
Hanif, S., Li, C., Alazzawe, A., and Latecki, L. J. (2019).
Image retrieval with similar object detection and local
similarity to detected objects. In Pacific Rim Inter-
national Conference on Artificial Intelligence, pages
42–55. Springer.
Kurar Barakat, B., Cohen, R., Droby, A., Rabaev, I., and
El-Sana, J. (2020). Learning-free text line segmen-
tation for historical handwritten documents. Applied
Sciences, 10(22):8276.
Lee, A. W., Chung, J., and Lee, M. (2021). Gnhk: A dataset
for english handwriting in the wild. In International
Conference on Document Analysis and Recognition,
pages 399–412. Springer.
Li, M., Lv, T., Cui, L., Lu, Y., Florencio, D., Zhang, C.,
Li, Z., and Wei, F. (2021). Trocr: Transformer-based
optical character recognition with pre-trained models.
arXiv preprint arXiv:2109.10282.
Liu, C.-L., Yin, F., Wang, D.-H., and Wang, Q.-F.
(2011). Casia online and offline chinese handwriting
databases. In 2011 International Conference on Doc-
ument Analysis and Recognition, pages 37–41. IEEE.
Marti, U.-V. and Bunke, H. (2002). The iam-database:
an english sentence database for offline handwrit-
ing recognition. International Journal on Document
Analysis and Recognition, 5(1):39–46.
Nguyen, H. T., Nakamura, T., Nguyen, C. T., and
Nakawaga, M. (2021). Online trajectory recovery
from offline handwritten japanese kanji characters of
multiple strokes. In 2020 25th International Con-
ference on Pattern Recognition (ICPR), pages 8320–
8327. IEEE.
Plamondon, R. and Srihari, S. N. (2000). Online and off-
line handwriting recognition: a comprehensive survey.
IEEE Transactions on pattern analysis and machine
intelligence, 22(1):63–84.
Privitera, C. M. and Plamondon, R. (1995). A system for
scanning and segmenting cursively handwritten words
into basic strokes. In Proceedings of 3rd International
Conference on Document Analysis and Recognition,
volume 2, pages 1047–1050. IEEE.
Rabhi, B., Elbaati, A., Boubaker, H., Hamdi, Y., Hussain,
A., and Alimi, A. M. (2021). Multi-lingual character
handwriting framework based on an integrated deep
learning based sequence-to-sequence attention model.
Memetic Computing, 13(4):459–475.
Rabhi, B., Elbaati, A., Boubaker, H., Pal, U., and Alimi,
A. (2022). Multi-lingual handwriting recovery frame-
work based on convolutional denoising autoencoder
with attention model.
Ren, S., He, K., Girshick, R., and Sun, J. (2015). Faster
r-cnn: Towards real-time object detection with region
proposal networks. Advances in neural information
processing systems, 28.
ICPRAM 2023 - 12th International Conference on Pattern Recognition Applications and Methods
670