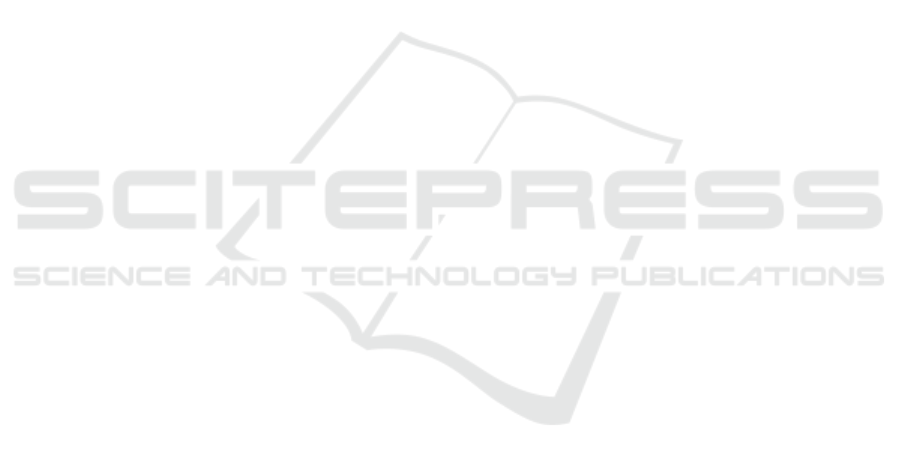
REFERENCES
Amiri, M., Brooks, R., Behboodi, B., and Rivaz, H. (2020).
Two-stage ultrasound image segmentation using u-net
and test time augmentation. International journal of
computer assisted radiology and surgery, 15(6):981–
988.
Chollet, F. (2021). Deep Learning with Python, Second Edi-
tion.
E-Helse (2019). Utredning om bruk av kunstig intelligens i
helsesektoren.
Fang, Y., Huang, H., Yang, W., Xu, X., Jiang, W., and
Lai, X. (2022). Nonlocal convolutional block atten-
tion module vnet for gliomas automatic segmentation.
International Journal of Imaging Systems and Tech-
nology, 32(2):528–543.
Huang, Q., Huang, Y., Luo, Y., Yuan, F., and Li, X. (2020).
Segmentation of breast ultrasound image with seman-
tic classification of superpixels. Medical image anal-
ysis, 61:101657.
Iqbal, A., Sharif, M., Yasmin, M., Raza, M., and Aftab, S.
(2022). Generative adversarial networks and its appli-
cations in the biomedical image segmentation: a com-
prehensive survey. International Journal of Multime-
dia Information Retrieval, pages 1–36.
Kheradmandi, N. and Mehranfar, V. (2022). A critical re-
view and comparative study on image segmentation-
based techniques for pavement crack detection. Con-
struction and Building Materials, 321:126162.
Lee, H., Park, J., and Hwang, J. Y. (2020). Channel at-
tention module with multiscale grid average pooling
for breast cancer segmentation in an ultrasound im-
age. IEEE transactions on ultrasonics, ferroelectrics,
and frequency control, 67(7):1344–1353.
Lin, M., Cai, Q., and Zhou, J. (2022a). 3d md-unet: A novel
model of multi-dataset collaboration for medical im-
age segmentation. Neurocomputing, 492:530–544.
Lin, Z., Zhang, Z., Han, L.-H., and Lu, S.-P. (2022b). Multi-
mode interactive image segmentation. In Proceedings
of the 30th ACM International Conference on Multi-
media, pages 905–914.
Liu, Z., Yang, C., Huang, J., Liu, S., Zhuo, Y., and Lu, X.
(2021). Deep learning framework based on integra-
tion of s-mask r-cnn and inception-v3 for ultrasound
image-aided diagnosis of prostate cancer. Future Gen-
eration Computer Systems, 114:358–367.
Ouahabi, A. and Taleb-Ahmed, A. (2021). Deep learn-
ing for real-time semantic segmentation: Application
in ultrasound imaging. Pattern Recognition Letters,
144:27–34.
Ronneberger, O., Fischer, P., and Brox, T. (2015). U-net:
Convolutional networks for biomedical image seg-
mentation. volume 9351.
Wang, H., Chen, Y., Cai, Y., Chen, L., Li, Y., Sotelo, M. A.,
and Li, Z. (2022). Sfnet-n: An improved sfnet al-
gorithm for semantic segmentation of low-light au-
tonomous driving road scenes. IEEE Transactions on
Intelligent Transportation Systems.
Wu, D., Zhuang, Z., Xiang, C., Zou, W., and Li, X. (2019).
6d-vnet: End-to-end 6-dof vehicle pose estimation
from monocular rgb images. In Proceedings of the
IEEE/CVF Conference on Computer Vision and Pat-
tern Recognition Workshops, pages 0–0.
Wu, L., Lu, M., and Fang, L. (2022). Deep covariance
alignment for domain adaptive remote sensing image
segmentation. IEEE Transactions on Geoscience and
Remote Sensing, 60:1–11.
Wu, Y., Shen, X., Bu, F., and Tian, J. (2020). Ultrasound
image segmentation method for thyroid nodules using
aspp fusion features. IEEE Access, 8:172457–172466.
Xue, C., Zhu, L., Fu, H., Hu, X., Li, X., Zhang, H., and
Heng, P.-A. (2021). Global guidance network for
breast lesion segmentation in ultrasound images. Med-
ical Image Analysis, page 101989.
You, C., Zhou, Y., Zhao, R., Staib, L., and Duncan, J. S.
(2022). Simcvd: Simple contrastive voxel-wise repre-
sentation distillation for semi-supervised medical im-
age segmentation. IEEE Transactions on Medical
Imaging.
Zeng, Y., Tsui, P.-H., Wu, W., Zhou, Z., and Wu, S. (2021).
Fetal ultrasound image segmentation for automatic
head circumference biometry using deeply supervised
attention-gated v-net. Journal of Digital Imaging,
34(1):134–148.
ICAART 2023 - 15th International Conference on Agents and Artificial Intelligence
564