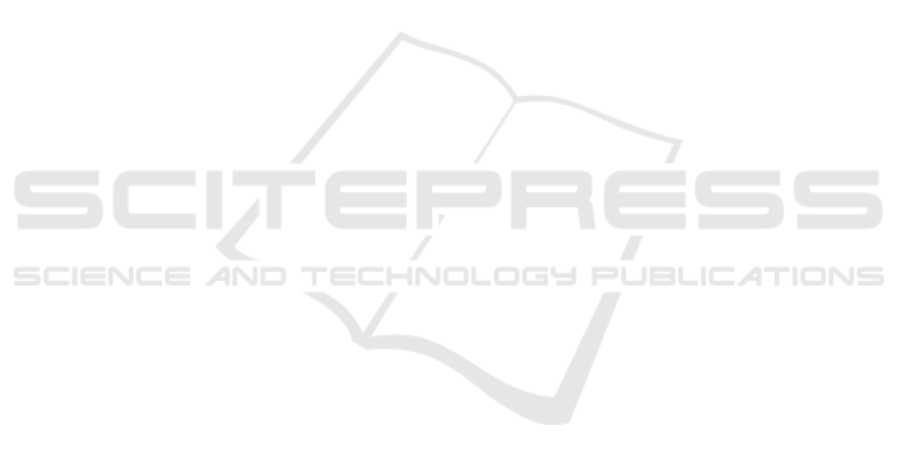
Relatively to the Experiment 3, the increasing of
training complexity caused by the increasing number
of training samples did improve the evaluation met-
rics results. So, it is recommended to train a novelty
detection technique for each real world scenario. In
this way, the technique can be optimized for samples
with the same features as the training samples.
The work presented in this paper solves the leather
detection problem. However, every day, new re-
searches present novelty detection methodologies that
overcome the previous state-of-the-art techniques.
So, for future work, the continuous upgrading of
leather detection solutions using recent novelty detec-
tion methodologies is mandatory. New ways to es-
timate the binary threshold, to convert the anomaly
score maps into a binary mask, should also be ex-
plored. Also, it is crucial to continue looking for solu-
tions with low computation requirements. Most of the
time, these solutions are applied in small computers
that do not have the required hardware to implement
the methodologies. On other hand, the presented so-
lutions can perform better if the training dataset was
bigger. In this way, it is necessary to invest in leather
image capture.
ACKNOWLEDGEMENT
This work is supported by: European Structural and
Investment Funds in the FEDER component, through
the Operational Competitiveness and International-
ization Programme (COMPETE 2020) [Project nº
42778; Funding Reference: POCI-01-0247-FEDER-
042778].
REFERENCES
ALLPI (2016). Africa leather and leather products institute
- hides and skins improvement handbook: Trainer’s
manual - hides and skins improvement handbook:
Trainer’s manual.
Aslam, M., Khan, T. M., Naqvi, S. S., Holmes, G., and
Naffa, R. (2020). Ensemble convolutional neural
networks with knowledge transfer for leather defect
classification in industrial settings. IEEE Access,
8:198600–198614.
Bergmann, P., Batzner, K., Fauser, M., Sattlegger, D., and
Steger, C. (2021). The mvtec anomaly detection
dataset: A comprehensive real-world dataset for un-
supervised anomaly detection. International Journal
of Computer Vision, 129:1038–1059.
Bergmann, P., Fauser, M., Sattlegger, D., and Steger,
C. (2019a). Mvtec ad-a comprehensive real-world
dataset for unsupervised anomaly detection. Pro-
ceedings of the IEEE Computer Society Conference
on Computer Vision and Pattern Recognition, 2019-
June:9584–9592.
Bergmann, P., L
¨
owe, S., Fauser, M., Sattlegger, D., and
Steger, C. (2019b). Improving unsupervised defect
segmentation by applying structural similarity to au-
toencoders. In Proceedings of the 14th Interna-
tional Joint Conference on Computer Vision, Imag-
ing and Computer Graphics Theory and Applications.
SCITEPRESS - Science and Technology Publications.
Bong, H. Q., Truong, Q. B., Nguyen, H. C., and Nguyen,
M. T. (2019). Vision-based inspection system for
leather surface defect detection and classification.
NICS 2018 - Proceedings of 2018 5th NAFOSTED
Conference on Information and Computer Science,
pages 300–304.
Defard, T., Setkov, A., Loesch, A., and Audigier, R. (2021).
Padim: a patch distribution modeling framework for
anomaly detection and localization. In International
Conference on Pattern Recognition, pages 475–489.
Springer.
Deng, H. and Li, X. (2022). Anomaly detection via reverse
distillation from one-class embedding. In Proceedings
of the IEEE/CVF Conference on Computer Vision and
Pattern Recognition, pages 9737–9746.
Gan, Y. S., Chee, S.-S., Huang, Y.-C., Liong, S.-T., and Yau,
W.-C. (2020). Automated leather defect inspection
using statistical approach on image intensity. Jour-
nal of Ambient Intelligence and Humanized Comput-
ing 2020 12:10, 12:9269–9285.
Georgieva, L., Krastev, K., and Angelov, N. (2003). Iden-
tification of surface leather defects. In CompSysTech,
volume 3, pages 303–307. Citeseer.
Gudovskiy, D., Ishizaka, S., and Kozuka, K. (2022). Cflow-
ad: Real-time unsupervised anomaly detection with
localization via conditional normalizing flows. In
2022 IEEE/CVF Winter Conference on Applications
of Computer Vision (WACV), pages 1819–1828.
Liong, S., Zheng, D., Huang, Y.-C., and Gan, Y. (2020).
Leather defect classification and segmentation using
deep learning architecture. International Journal of
Computer Integrated Manufacturing, 33:1–13.
Liong, S.-T., Gan, Y. S., Huang, Y.-C., Liu, K.-H., and Yau,
W.-C. (2019). Integrated neural network and machine
vision approach for leather defect classification. arXiv
preprint arXiv:1905.11731.
Wang, G., Han, S., Ding, E., and Huang, D. (2021).
Student-teacher feature pyramid matching for un-
supervised anomaly detection. arXiv preprint
arXiv:2103.04257.
Zavrtanik, V., Kristan, M., and Skocaj, D. (2021). Draem
- a discriminatively trained reconstruction embedding
for surface anomaly detection. In Proceedings of the
IEEE/CVF International Conference on Computer Vi-
sion (ICCV), pages 8330–8339.
VISAPP 2023 - 18th International Conference on Computer Vision Theory and Applications
204