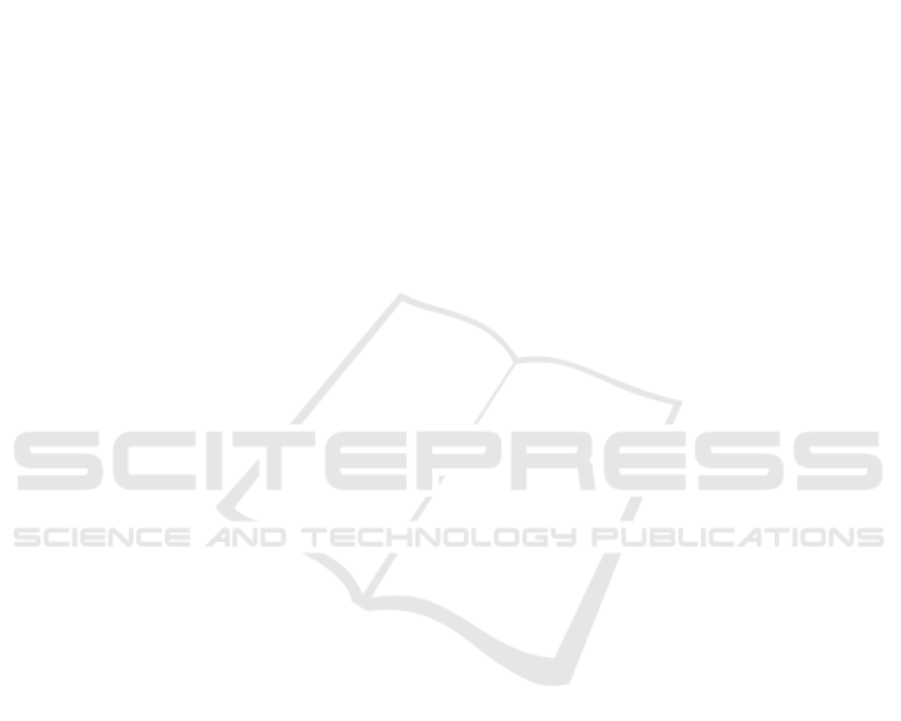
Ultra-Low Power Electronic Circuits Inspired by Biological Genetic
Processes
Ilan Oren, Raghd Abu-Sinni and Ramez Daniel
Faculty of Bio-Medical Engineering, Technion - Israel Institute of Technology, Haifa 3200003, Israel
Keywords:
Subthreshold Electronic Circuits, Analog Design, Neural Network, Molecular Network, Bio-Inspired.
Abstract:
Neuromorphic engineering, inspired by principles and architecture of neuronal circuitries, enabled the design
of Artificial Neural networks (ANNs) for Intelligent systems. These systems perform very complex computa-
tion tasks, yet they consume significant power. Thus, using artificial intelligence (AI) for applications where
only a small power source is available is very limited. While the neuronal networks in the brain can recognize
complex patterns and memorize enormous elements, molecular and protein networks can perform other com-
plex tasks such as adaptive immunity and cell differentiation at high energy efficiency. Here, we claim that a
bio-inspired computing platform mimicking molecular protein networks can lead to ultra-low power emergent
computation. Previously, we proposed a molecular-inspired computing model named Perceptgene that has the
attributes of learning and adaptivity as the neural network (Rizik et al., 2022). Similarities were found be-
tween equations describing biochemical reactions and transistor operation at subthreshold (Sarpeshkar, 2011)
enabling the design of Perceptgene with subthreshold electrical circuits. Thus, the subthreshold Perceptgene
circuits are expected to allow computing and learning capabilities at ultra-low power consumption.
1 INTRODUCTION
Biological neural systems are comprised of remark-
able parallel and distributed computing networks with
adaptive, self-repairing, and replicative capacities in
the performance of real-world tasks. Scientists and
engineers have been inspired to mimic these features
when designing artificial intelligence systems. Neu-
romorphic computing (Mead, 1990) applies abstract
models of neural systems, such as the perceptron
(Haykin, 2004), and uses microelectronics to build
artificial intelligent machines. Today, the world is in-
creasingly dependent upon this artificial intelligence
(AI) and machine learning (ML) systems in several
fields. Among these fields are health and finance, face
and object recognition, command of autonomous ve-
hicles, speech recognition, and natural language pro-
cessing. However, the power consumption of cur-
rent deep-learning machines and ‘layered neural net-
works’ is one of the most challenging limitations
of these systems. The steep increase in their en-
ergy consumption and the computing power required
for training them, which has grown 300,000-fold be-
tween 2012-18, are both unsustainable, putting sub-
stantial applications beyond the reach of all but well-
resourced organizations. While the brain can perform
sophisticated information processing by employing
complex neuronal circuit topologies with highly inter-
connected nodes, molecular biological systems con-
tain extensively noisy parts that collectively interact
to solve parallel tasks online with high energy effi-
ciency. A single cell in the body, for example, per-
forms 10 million energy-consuming biochemical op-
erations per second on its noisy molecular inputs at
1pW of average power (Sarpeshkar, 2010). In this
study, we propose ultra-low power electronic cir-
cuits inspired by gene networks to demonstrate
the computational abilities of neuronal networks.
This approach relies on insights we have gained
that map neuronal networks to molecular biologi-
cal systems (biomorphic (Rizik et al., 2022) (Daniel
et al., 2013)) and then to electronic circuits (cyto-
morphic (Sarpeshkar, 2011) (Hanna et al., 2020) ),
as shown in Fig. 1. The proposed computational ap-
proach is realized by building subthreshold electronic
circuits mimicking molecular networks based on the
Perceptgene model [1]. We anticipate that subthresh-
old Perceptgene circuits will enable the implementa-
tion of an adaptive system with ultra-low power com-
putation abilities.
150
Oren, I., Abu-Sinni, R. and Daniel, R.
Ultra-Low Power Electronic Circuits Inspired by Biological Genetic Processes.
DOI: 10.5220/0011707800003414
In Proceedings of the 16th International Joint Conference on Biomedical Engineering Systems and Technologies (BIOSTEC 2023) - Volume 1: BIODEVICES, pages 150-156
ISBN: 978-989-758-631-6; ISSN: 2184-4305
Copyright
c
2023 by SCITEPRESS – Science and Technology Publications, Lda. Under CC license (CC BY-NC-ND 4.0)