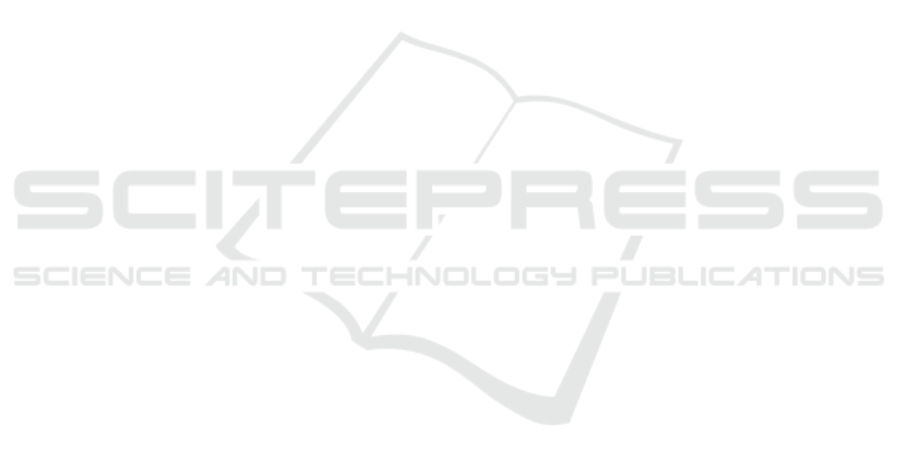
He, K., Zhang, X., Ren, S., and Sun, J. (2016). Deep resid-
ual learning for image recognition. 2016 IEEE Con-
ference on Computer Vision and Pattern Recognition
(CVPR), pages 770–778.
Henriksen, K. S., Lynge, M. S., Jeppesen, M. D. B., Al-
lahham, M. M. J., Nikolov, I. A., Haurum, J. B.,
and Moeslund, T. B. (2020). Generating synthetic
point clouds of sewer networks: An initial investi-
gation. In Augmented Reality, Virtual Reality, and
Computer Graphics: 7th International Conference,
AVR 2020, Lecce, Italy, September 7–10, 2020, Pro-
ceedings, Part II, page 364–373, Berlin, Heidelberg.
Springer-Verlag.
Huang, G., Liu, Z., and Weinberger, K. Q. (2017). Densely
connected convolutional networks. 2017 IEEE Con-
ference on Computer Vision and Pattern Recognition
(CVPR), pages 2261–2269.
Iyer, S. and Sinha, S. (2006). Segmentation of pipe images
for crack detection in buried sewers. Comp.-Aided
Civil and Infrastruct. Engineering, 21:395–410.
Iyer, S., Sinha, S. K., Pedrick, M., and Tittmann, B. R.
(2012). Evaluation of ultrasonic inspection and imag-
ing systems for concrete pipes. Automation in Con-
struction, 22:149–164.
Ji, H. W., Yoo, S. S., Lee, B.-J., Koo, D. D., and Kang, J.-
H. (2020). Measurement of wastewater discharge in
sewer pipes using image analysis. Water, 12(6).
Kumar, S., Wang, M., Abraham, D., Jahanshahi, M., Iseley,
T., and Cheng, J. (2020). Deep learning–based auto-
mated detection of sewer defects in cctv videos. Jour-
nal of Computing in Civil Engineering, 34:04019047.
Kumar, S. S., Abraham, D. M., Jahanshahi, M. R., Iseley,
T., and Starr, J. (2018). Automated defect classifica-
tion in sewer closed circuit television inspections us-
ing deep convolutional neural networks. Automation
in Construction, 91:273–283.
Lepot, M., Stani
´
c, N., and Clemens, F. H. (2017). A tech-
nology for sewer pipe inspection (part 2): Experimen-
tal assessment of a new laser profiler for sewer defect
detection and quantification. Automation in Construc-
tion, 73:1–11.
Li, D., Cong, A., and Guo, S. (2019). Sewer damage detec-
tion from imbalanced cctv inspection data using deep
convolutional neural networks with hierarchical clas-
sification. Automation in Construction, 101:199–208.
Liu, Z. and Kleiner, Y. (2013). State of the art review of in-
spection technologies for condition assessment of wa-
ter pipes. Measurement, 46:1–15.
Meijer, D., Scholten, L., Clemens, F., and Knobbe, A.
(2019). A defect classification methodology for sewer
image sets with convolutional neural networks. Au-
tomation in Construction, 104:281–298.
Montero, R., Victores, J., Mart
´
ınez, S., Jard
´
on, A., and
Balaguer, C. (2015). Past, present and future of
robotic tunnel inspection. Automation in Construc-
tion, 59:99–112.
Moradi, S., Zayed, T., Nasiri, F., and Golkhoo, F. (2020).
Automated anomaly detection and localization in
sewer inspection videos using proportional data mod-
eling and deep learning–based text recognition. Jour-
nal of Infrastructure Systems, 26:04020018.
Myrans, J., Everson, R. M., and Kapelan, Z. (2018). Auto-
mated detection of faults in sewers using cctv image
sequences. Automation in Construction.
Pan, G., Zheng, Y., Guo, S., and Lv, Y. (2020). Auto-
matic sewer pipe defect semantic segmentation based
on improved u-net. Automation in Construction,
119:103383.
Piciarelli, C., Avola, D., Pannone, D., and Foresti, G. L.
(2019). A vision-based system for internal pipeline
inspection. IEEE Transactions on Industrial Informat-
ics, 15(6):3289–3299.
Sandler, M., Howard, A., Zhu, M., Zhmoginov, A., and
Chen, L.-C. (2018). Mobilenetv2: Inverted residu-
als and linear bottlenecks. In 2018 IEEE/CVF Con-
ference on Computer Vision and Pattern Recognition,
pages 4510–4520.
Sinha, K. and Fieguth, W. (2006). Morphological segmen-
tation and classification of underground pipe images.
Mach. Vision Appl., 17(1):21–31.
Szegedy, C., Vanhoucke, V., Ioffe, S., Shlens, J., and Wojna,
Z. (2016). Rethinking the inception architecture for
computer vision. In 2016 IEEE Conference on Com-
puter Vision and Pattern Recognition (CVPR), pages
2818–2826.
Tan, M. and Le, Q. (2019). EfficientNet: Rethinking model
scaling for convolutional neural networks. In Chaud-
huri, K. and Salakhutdinov, R., editors, Proceedings of
the 36th International Conference on Machine Learn-
ing, volume 97 of Proceedings of Machine Learning
Research, pages 6105–6114. PMLR.
Wang, J., Sun, K., Cheng, T., Jiang, B., Deng, C., Zhao,
Y., Liu, D., Mu, Y., Tan, M., Wang, X., Liu, W., and
Xiao, B. (2019). Deep high-resolution representation
learning for visual recognition. TPAMI.
Wang, M. and Cheng, J. C. P. (2020). A unified con-
volutional neural network integrated with conditional
random field for pipe defect segmentation. Comput.-
Aided Civ. Infrastruct. Eng., 35(2):162–177.
Wang, M., Kumar, S. S., and Cheng, J. C. (2021). Auto-
mated sewer pipe defect tracking in cctv videos based
on defect detection and metric learning. Automation
in Construction, 121:103438.
Xie, Q., Li, D., Xu, J., Yu, Z., and Wang, J. (2019). Auto-
matic detection and classification of sewer defects via
hierarchical deep learning. IEEE Transactions on Au-
tomation Science and Engineering, 16(4):1836–1847.
Yin, X., Chen, Y., Bouferguene, A., Zaman, H., Al-Hussein,
M., and Kurach, L. (2020). A deep learning-based
framework for an automated defect detection sys-
tem for sewer pipes. Automation in Construction,
109:102967.
ICPRAM 2023 - 12th International Conference on Pattern Recognition Applications and Methods
904