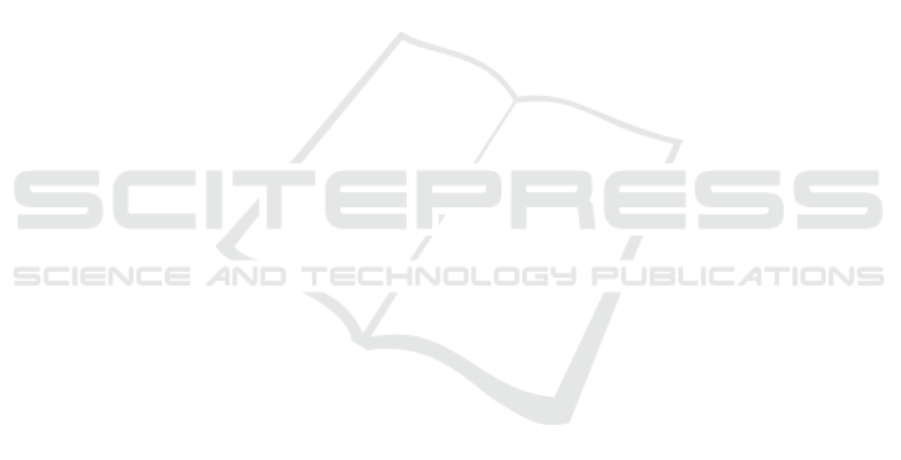
Liang, J., Hu, D., and Feng, J. (2021). Domain adapta-
tion with auxiliary target domain-oriented classifier.
In Proceedings of the IEEE/CVF Conference on Com-
puter Vision and Pattern Recognition (CVPR).
Liu, H., Long, M., Wang, J., and Jordan, M. (2019).
Transferable adversarial training: A general approach
to adapting deep classifiers. In Proceedings of the
36th International Conference on Machine Learning
(PMLR).
Liu, H., Wang, J., and Long, M. (2021). Cycle self-training
for domain adaptation. In Advances in Neural Infor-
mation Processing Systems.
Ma, W., Zhang, J., Li, S., Liu, C. H., Wang, Y., and Li, W.
(2021). Exploiting both domain-specific and invari-
ant knowledge via a win-win transformer for unsuper-
vised domain adaptation.
Na, J., Han, D., Chang, H. J., and Hwang, W. (2021a). Con-
trastive vicinal space for unsupervised domain adapta-
tion.
Na, J., Jung, H., Chang, H. J., and Hwang, W. (2021b).
Fixbi: Bridging domain spaces for unsupervised do-
main adaptation. In Proceedings of the IEEE/CVF
Conference on Computer Vision and Pattern Recog-
nition (CVPR).
Peng, X., Bai, Q., Xia, X., Huang, Z., Saenko, K., and
Wang, B. (2019). Moment matching for multi-source
domain adaptation. In Proceedings of the IEEE/CVF
International Conference on Computer Vision (ICCV).
Peng, X., Usman, B., Kaushik, N., Hoffman, J., Wang, D.,
and Saenko, K. (2017). Visda: The visual domain
adaptation challenge.
Russakovsky, O., Deng, J., Su, H., Krause, J., Satheesh, S.,
Ma, S., Huang, Z., Karpathy, A., Khosla, A., Bern-
stein, M., and et al. (2015). Imagenet large scale vi-
sual recognition challenge. International Journal of
Computer Vision, 115(3).
Saenko, K., Kulis, B., Fritz, M., and Darrell, T. (2010).
Adapting visual category models to new domains. In
European Conference on Computer Vision (ECCV).
Sagawa, S. and Hino, H. (2022). Gradual domain adaptation
via normalizing flows.
Tang, H., Chen, K., and Jia, K. (2020). Unsupervised do-
main adaptation via structurally regularized deep clus-
tering. In Proceedings of the IEEE/CVF Conference
on Computer Vision and Pattern Recognition (CVPR).
Venkateswara, H., Eusebio, J., Chakraborty, S., and Pan-
chanathan, S. (2017). Deep hashing network for un-
supervised domain adaptation. In IEEE Conference
on Computer Vision and Pattern Recognition (CVPR).
Wang, X., Li, L., Ye, W., Long, M., and Wang, J. (2019).
Transferable attention for domain adaptation. Pro-
ceedings of the AAAI Conference on Artificial Intel-
ligence, 33(1).
Wei, G., Lan, C., Zeng, W., and Chen, Z. (2021). Metaalign:
Coordinating domain alignment and classification for
unsupervised domain adaptation. In Proceedings of
the IEEE/CVF Conference on Computer Vision and
Pattern Recognition (CVPR).
Wilson, G. and Cook, D. J. (2020). A survey of unsuper-
vised deep domain adaptation. ACM Transactions on
Intelligent Systems and Technology, 11(5).
Wu, Y., Inkpen, D., and El-Roby, A. (2020). Dual mixup
regularized learning for adversarial domain adapta-
tion. In European Conference on Computer Vision
(ECCV).
Xu, M., Zhang, J., Ni, B., Li, T., Wang, C., Tian, Q., and
Zhang, W. (2020). Adversarial domain adaptation
with domain mixup. Proceedings of the AAAI Con-
ference on Artificial Intelligence, 34(4).
Xu, T., Chen, W., Wang, P., Wang, F., Li, H., and Jin, R.
(2021). Cdtrans: Cross-domain transformer for unsu-
pervised domain adaptation.
Yan, S., Song, H., Li, N., Zou, L., and Ren, L. (2020).
Improve unsupervised domain adaptation with mixup
training.
Yang, G., Tang, H., Zhong, Z., Ding, M., Shao, L., Sebe, N.,
and Ricci, E. (2021a). Transformer-based source-free
domain adaptation.
Yang, L., Wang, Y., Gao, M., Shrivastava, A., Weinberger,
K. Q., Chao, W.-L., and Lim, S.-N. (2021b). Deep co-
training with task decomposition for semi-supervised
domain adaptation. In Proceedings of the IEEE/CVF
International Conference on Computer Vision (ICCV).
Yun, S., Han, D., Oh, S. J., Chun, S., Choe, J., and Yoo,
Y. (2019). Cutmix: Regularization strategy to train
strong classifiers with localizable features. In Pro-
ceedings of the IEEE/CVF International Conference
on Computer Vision (ICCV).
Zhang, H., Cisse, M., Dauphin, Y. N., and Lopez-Paz, D.
(2017). Mixup: Beyond empirical risk minimization.
Zhang, Y., Deng, B., Jia, K., and Zhang, L. (2021a). Grad-
ual domain adaptation via self-training of auxiliary
models.
Zhang, Y., Li, J., and Wang, Z. (2022). Low-confidence
samples matter for domain adaptation.
Zhang, Y., Wang, Z., and Mao, Y. (2021b). Rpn prototype
alignment for domain adaptive object detector. In Pro-
ceedings of the IEEE/CVF Conference on Computer
Vision and Pattern Recognition (CVPR).
Zhu, R., Zhao, B., Liu, J., Sun, Z., and Chen, C. W. (2021).
Improving contrastive learning by visualizing feature
transformation. In Proceedings of the IEEE/CVF In-
ternational Conference on Computer Vision (ICCV).
Zhuang, F., Qi, Z., Duan, K., Xi, D., Zhu, Y., Zhu, H.,
Xiong, H., and He, Q. (2021). A comprehensive sur-
vey on transfer learning. Proceedings of the IEEE,
109(1).
IFMix: Utilizing Intermediate Filtered Images for Domain Adaptation in Classification
211