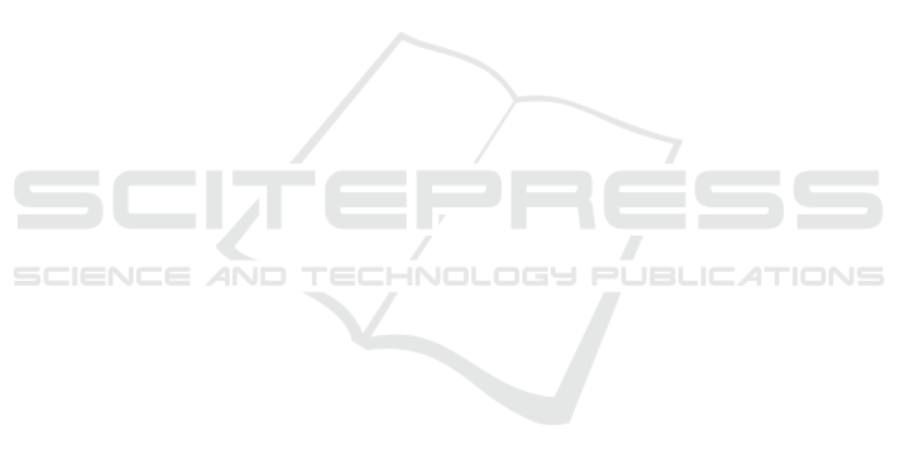
ture aspirations could be devoted to improve the ini-
tialization methods. Additionally, due to the impor-
tance of prepossessing data in machine learning mod-
els, to further enhance the model accuracy, feature se-
lection and data quality assessment methods could be
investigated.
REFERENCES
Alawadi, S., Mera, D., Fernandez-Delgado, M., Alkhab-
bas, F., Olsson, C. M., and Davidsson, P. (2020). A
comparison of machine learning algorithms for fore-
casting indoor temperature in smart buildings. Energy
Systems, pages 1–17.
Ali, S. and Bouguila, N. (2022). Towards scalable de-
ployment of hidden markov models in occupancy es-
timation: A novel methodology applied to the study
case of occupancy detection. Energy and Buildings,
254:111594.
Amayri, M., Arora, A., Ploix, S., Bandhyopadyay, S., Ngo,
Q.-D., and Badarla, V. R. (2016). Estimating occu-
pancy in heterogeneous sensor environment. Energy
and Buildings, 129:46–58.
Amayri, M., Ploix, S., Bouguila, N., and Wurtz, F. (2020).
Database quality assessment for interactive learning:
Application to occupancy estimation. Energy and
Buildings, 209:109578.
Bdiri, T. and Bouguila, N. (2012). Positive vectors clus-
tering using inverted dirichlet finite mixture models.
Expert Systems with Applications, 39(2):1869–1882.
Bdiri, T. and Bouguila, N. (2013). Bayesian learning of in-
verted dirichlet mixtures for svm kernels generation.
Neural Computing and Applications, 23(5):1443–
1458.
Bouguila, N. (2012). Infinite liouville mixture models with
application to text and texture categorization. Pattern
Recognition Letters, 33(2):103–110.
Bouguila, N., Fan, W., and Amayri, M. (2022). Hidden
Markov Models and Applications. Springer.
Bourouis, S., Alroobaea, R., Rubaiee, S., Andejany, M.,
M.Almansour, F., and Bouguila, N. (2021). Markov
chain monte carlo-based bayesian inference for learn-
ing finite and infinite inverted beta-liouville mixture
models. IEEE Access, 9:71170–71183.
Bourouis, S., Mashrgy, M. A., and Bouguila, N. (2014a).
Bayesian learning of finite generalized inverted
dirichlet mixtures: Application to object classifi-
cation and forgery detection. Expert Syst. Appl.,
41(5):2329–2336.
Bourouis, S., Mashrgy, M. A., and Bouguila, N. (2014b).
Bayesian learning of finite generalized inverted dirich-
let mixtures: Application to object classification and
forgery detection. Expert Systems with Applications,
41(5):2329–2336.
Ebadat, A., Bottegal, G., Varagnolo, D., Wahlberg, B., and
Johansson, K. H. (2013). Estimation of building oc-
cupancy levels through environmental signals decon-
volution. In Proceedings of the 5th ACM Workshop
on Embedded Systems For Energy-Efficient Buildings,
pages 1–8.
Epaillard, E. and Bouguila, N. (2016). Proportional data
modeling with hidden markov models based on gen-
eralized dirichlet and beta-liouville mixtures applied
to anomaly detection in public areas. Pattern Recog-
nit., 55:125–136.
Erickson, V. L., Carreira-Perpi, M. A., and Cerpa, A. E.
(2014). Occupancy modeling and prediction for build-
ing energy management. ACM Transactions on Sensor
Networks (TOSN), 10(3):1–28.
Fang, K. T., Kotz, S., and Wangng, K. (2018). Symmetric
Multivariate and Related Distributions. Chapman and
Hall/CRC.
Gaetani, I., Hoes, P.-J., and Hensen, J. L. (2016). Occupant
behavior in building energy simulation: Towards a fit-
for-purpose modeling strategy. Energy and Buildings,
121:188–204.
Hu, C., Fan, W., Du, J.-X., and Bouguila, N. (2019). A
novel statistical approach for clustering positive data
based on finite inverted beta-liouville mixture models.
Neurocomputing, 333:110–123.
Kim, D., Yoon, Y., Lee, J., Mago, P., Lee, K., and Cho, H.
(2022). Design and implementation of smart build-
ings: A review of current research trend. Energies,
15:4278.
Koochemeshkian, P., Zamzami, N., and Bouguila, N.
(2020). Distribution-based regression models for
semi-bounded data analysis. In 2020 IEEE Interna-
tional Conference on Systems, Man, and Cybernetics
(SMC), pages 4073–4080. IEEE.
lingmnwah, G. (1976). On the generalised inverted dirichlet
distribution. Demonstratio Mathematica, 9(3):119–
130.
Nasfi, R., Amayri, M., and Bouguila, N. (2020). A novel
approach for modeling positive vectors with inverted
dirichlet-based hidden markov models. Knowledge-
Based Systems, 192:105335.
Nguyen, H., Rahmanpour, M., Manouchehri, N., Maanic-
shah, K., Amayri, M., and Bouguila, N. (2019). A
statistical approach for unsupervised occupancy de-
tection and estimation in smart buildings. In 2019
IEEE International Smart Cities Conference (ISC2),
pages 414–419. IEEE.
Soltanaghaei, E. and Whitehouse, K. (2016). Walksense:
Classifying home occupancy states using walkway
sensing. BuildSys ’16, page 167–176, New York, NY,
USA. Association for Computing Machinery.
Tiao, G. G. and Cuttman, I. (1965). The inverted dirichlet
distribution with applications. Journal of the Ameri-
can Statistical Association, 60(311):793–805.
Vai
ˇ
ciulyt
˙
e, J. and Sakalauskas, L. (2020). Recursive pa-
rameter estimation algorithm of the dirichlet hidden
markov model. Journal of Statistical Computation
and Simulation, 90(2):306–323.
SMARTGREENS 2023 - 12th International Conference on Smart Cities and Green ICT Systems
88