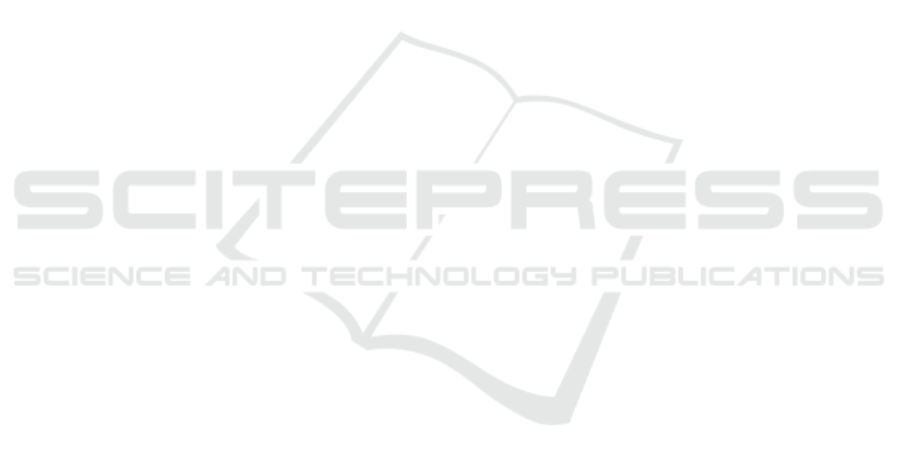
23/2339734/0/en/Abercrombie-Fitch-Co-Reports-
Third-Quarter-Results.html.
Hansen, J. V., McDonald, J. B., and Nelson, R. D. (1999).
Time series prediction with genetic-algorithm de-
signed neural networks: An empirical comparison
with modern statistical models. Computational Intel-
ligence, 15(3):171–184.
Hinton, G. E., Srivastava, N., Krizhevsky, A., Sutskever,
I., and Salakhutdinov, R. (2012). Improving neural
networks by preventing co-adaptation of feature de-
tectors. CoRR, abs/1207.0580.
Hryshko, A. and Downs, T. (2004). System for foreign
exchange trading using genetic algorithms and rein-
forcement learning. International Journal of Systems
Science, 35(13-14):763–774.
Investopedia (2019). 3 oil and gas stocks to watch this
week. https://www.investopedia.com/3-oil-and-gas-
stocks-to-watch-this-week-4628357.
Investopedia (2020). Buy and hold definition. https://www.
investopedia.com/terms/b/buyandhold.asp.
Investopedia (2022a). Break-even analysis. https://www.
investopedia.com/terms/b/breakevenanalysis.asp.
Investopedia (2022b). Calmar ratio. https://www.
investopedia.com/terms/c/calmarratio.asp.
Investopedia (2022c). Sharpe ratio. https://www.
investopedia.com/terms/s/sharperatio.asp.
Investopedia (2022d). Sortino ratio. https://www.
investopedia.com/terms/s/sortinoratio.asp.
Krizhevsky, A., Sutskever, I., and Hinton, G. E. (2012).
Imagenet classification with deep convolutional neu-
ral networks. In Proceedings of the 25th Interna-
tional Conference on Neural Information Processing
Systems - Volume 1, NIPS’12, page 1097–1105, Red
Hook, NY, USA. Curran Associates Inc.
Kumbure, M. M., Lohrmann, C., Luukka, P., and Porras,
J. (2022). Machine learning techniques and data for
stock market forecasting: A literature review. Expert
Systems with Applications, 197:116659.
Lee, T.-S. and Chiu, C.-C. (2002). Neural network fore-
casting of an opening cash price index. International
Journal of Systems Science, 33(3):229–237.
Letteri, I., Della Penna, G., and Caianiello, P. (2019a). Fea-
ture selection strategies for HTTP botnet traffic de-
tection. In 2019 IEEE European Symposium on Se-
curity and Privacy Workshops, EuroS&P Workshops
2019, Stockholm, Sweden, June 17-19, 2019, pages
202–210. IEEE.
Letteri, I., Della Penna, G., and De Gasperis, G. (2018).
Botnet detection in software defined networks by deep
learning techniques. In Castiglione, A., Pop, F., Ficco,
M., and Palmieri, F., editors, Cyberspace Safety and
Security - 10th International Symposium, CSS 2018,
Amalfi, Italy, October 29-31, 2018, Proceedings, vol-
ume 11161 of Lecture Notes in Computer Science,
pages 49–62. Springer.
Letteri, I., Della Penna, G., and De Gasperis, G. (2019b).
Security in the internet of things: botnet detec-
tion in software-defined networks by deep learning
techniques. Int. J. High Perform. Comput. Netw.,
15(3/4):170–182.
Letteri, I., Della Penna, G., De Gasperis, G., and Dyoub, A.
(2022a). A stock trading system for a medium volatile
asset using multi layer perceptron.
Letteri, I., Della Penna, G., Di Vita, L., and Grifa, M. T.
(2020a). Mta-kdd’19: A dataset for malware traf-
fic detection. In Loreti, M. and Spalazzi, L., editors,
Proceedings of the Fourth Italian Conference on Cy-
ber Security, Ancona, Italy, February 4th to 7th, 2020,
volume 2597 of CEUR Workshop Proceedings, pages
153–165. CEUR-WS.org.
Letteri, I., Di Cecco, A., and Della Penna, G. (2020b).
Dataset optimization strategies for malware traffic de-
tection. CoRR, abs/2009.11347.
Letteri, I., Di Cecco, A., Dyoub, A., and Della Penna, G.
(2021). Imbalanced dataset optimization with new re-
sampling techniques. In Arai, K., editor, Intelligent
Systems and Applications - Proceedings of the 2021
Intelligent Systems Conference, IntelliSys 2021, Am-
sterdam, The Netherlands, 2-3 September, 2021, Vol-
ume 2, volume 295 of Lecture Notes in Networks and
Systems, pages 199–215. Springer.
Letteri, I., Penna, G. D., Gasperis, G. D., and Dyoub, A.
(2022b). Dnn-forwardtesting: A new trading strat-
egy validation using statistical timeseries analysis and
deep neural networks. CoRR, abs/2210.11532.
Linke, A., Mash, L., Fong, C., Kinnear, M., Kohli, J.,
Wilkinson, M., Tung, R., Jao Keehn, R., Carper, R.,
Fishman, I., and Müller, R.-A. (2020). Dynamic time
warping outperforms pearson correlation in detecting
atypical functional connectivity in autism spectrum
disorders. NeuroImage, 223:117383.
Lu, J. and Ohta, H. (2002). Using the optimization layer-
by-layer learning algorithm on local-recurrent-global-
feedforward networks in financial time series pre-
dictions. International Journal of Systems Science,
33(12):959–967.
Marín, G., Caasas, P., and Capdehourat, G. (2021). Deep-
mal - deep learning models for malware traffic detec-
tion and classification. In Haber, P., Lampoltshammer,
T., Mayr, M., and Plankensteiner, K., editors, Data
Science – Analytics and Applications, pages 105–112,
Wiesbaden. Springer Fachmedien Wiesbaden.
Marquez, J. (1995). Time series analysis : James d. hamil-
ton, 1994, (princeton university press, princeton, nj).
International Journal of Forecasting, 11(3):494–495.
Mehra, R. (1998). On the volatility of stock prices: an ex-
ercise in quantitative theory. International Journal of
Systems Science, 29(11):1203–1211.
Mondal, S. S., Mohanty, S. P., Harlander, B., Koseoglu,
M., Rane, L., Romanov, K., Liu, W.-K., Hatwar,
P., Salathe, M., and Byrum, J. (2019). Investment
Ranking Challenge: Identifying the best performing
stocks based on their semi-annual returns. Papers
1906.08636, arXiv.org.
Prasad, P. S. K., Madhav, V., Lal, R., and Ravi, V. (2022).
Optimal technical indicator-based trading strategies
using nsga-ii.
scikit-learn (2022). Gridsearchcv. https://scikit-
learn.org/stable/modules/generated/sklearn.model\
_selection.GridSearchCV.html.
FEMIB 2023 - 5th International Conference on Finance, Economics, Management and IT Business
24