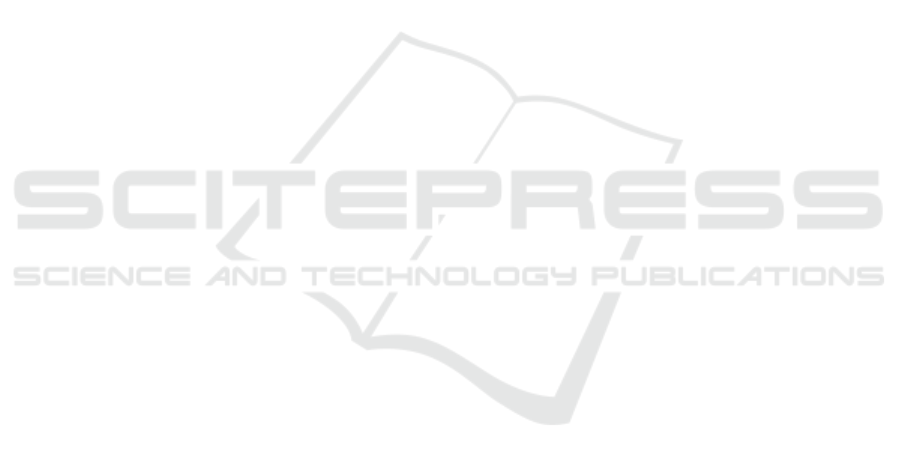
Elkan, C. (2001). The foundations of cost-sensitive learn-
ing. In International joint conference on artificial in-
telligence, volume 17, pages 973–978. Lawrence Erl-
baum Associates Ltd.
Fang, Z., Zhu, S., Zhang, J., Liu, Y., Chen, Z., and He, Y.
(2020). Low rank directed acyclic graphs and causal
structure learning. arXiv preprint arXiv:2006.05691.
Gencoglu, O. and Gruber, M. (2020). Causal modeling
of twitter activity during Covid-19. Computation,
8(4):85.
Heindorf, S., Scholten, Y., Wachsmuth, H.,
Ngonga Ngomo, A.-C., and Potthast, M. (2020).
Causenet: Towards a causality graph extracted from
the web. In Proceedings of the 29th ACM Inter-
national Conference on Information & Knowledge
Management, pages 3023–3030.
Holzinger, A. (2016). Interactive machine learning for
health informatics: when do we need the human-in-
the-loop? Brain Informatics, 3(2):119–131.
Jaber, A., Zhang, J., and Bareinboim, E. (2018). Causal
identification under Markov equivalence. arXiv
preprint arXiv:1812.06209.
Lachapelle, S., Brouillard, P., Deleu, T., and Lacoste-Julien,
S. (2019). Gradient-based neural DAG learning. arXiv
preprint arXiv:1906.02226.
Lake, B. M., Ullman, T. D., Tenenbaum, J. B., and Gersh-
man, S. J. (2017). Building machines that learn and
think like people. Behavioral and brain sciences, 40.
Li, Z., Ding, X., Liu, T., Hu, J. E., and Van Durme, B.
(2021). Guided generation of cause and effect. arXiv
preprint arXiv:2107.09846.
Liu, J., Chen, Y., and Zhao, J. (2021). Knowledge enhanced
event causality identification with mention masking
generalizations. In Proceedings of the Twenty-Ninth
International Conference on International Joint Con-
ferences on Artificial Intelligence, pages 3608–3614.
Magliacane, S., van Ommen, T., Claassen, T., Bongers,
S., Versteeg, P., and Mooij, J. M. (2017). Do-
main adaptation by using causal inference to pre-
dict invariant conditional distributions. arXiv preprint
arXiv:1707.06422.
Ng, I., Fang, Z., Zhu, S., Chen, Z., and Wang, J.
(2019). Masked gradient-based causal structure learn-
ing. arXiv preprint arXiv:1910.08527.
Ng, I., Ghassami, A., and Zhang, K. (2020). On the role
of sparsity and DAG constraints for learning linear
DAGs. arXiv preprint arXiv:2006.10201.
Ng, I., Lachapelle, S., Ke, N. R., Lacoste-Julien, S., and
Zhang, K. (2022). On the convergence of continuous
constrained optimization for structure learning. In In-
ternational Conference on Artificial Intelligence and
Statistics, pages 8176–8198. PMLR.
O’Donnell, R. T., Nicholson, A. E., Han, B., Korb, K. B.,
Alam, M. J., and Hope, L. R. (2006). Causal discov-
ery with prior information. In Australasian Joint Con-
ference on Artificial Intelligence, pages 1162–1167.
Springer.
Pearl, J. (2009). Causality. Cambridge university press.
Pearl, J. and Verma, T. S. (1995). A theory of inferred cau-
sation. In Studies in Logic and the Foundations of
Mathematics, volume 134, pages 789–811. Elsevier.
Peters, J., Janzing, D., and Sch
¨
olkopf, B. (2017). Elements
of causal inference: foundations and learning algo-
rithms. The MIT Press.
Ramsey, J., Glymour, M., Sanchez-Romero, R., and Gly-
mour, C. (2017). A million variables and more: the
Fast Greedy Equivalence Search algorithm for learn-
ing high-dimensional graphical causal models, with
an application to functional magnetic resonance im-
ages. International journal of data science and ana-
lytics, 3(2):121–129.
Reisach, A., Seiler, C., and Weichwald, S. (2021). Beware
of the simulated dag! causal discovery benchmarks
may be easy to game. Advances in Neural Information
Processing Systems, 34:27772–27784.
Sachs, K., Perez, O., Pe’er, D., Lauffenburger, D. A., and
Nolan, G. P. (2005). Causal protein-signaling net-
works derived from multiparameter single-cell data.
Science, 308(5721):523–529.
Scutari, M. (2009). Learning bayesian networks with the
bnlearn r package. arXiv preprint arXiv:0908.3817.
Sharma, A. and Kiciman, E. (2020). Dowhy: An end-
to-end library for causal inference. arXiv preprint
arXiv:2011.04216.
Spirtes, P., Glymour, C. N., Scheines, R., and Heckerman,
D. (2000). Causation, prediction, and search. MIT
press.
Wei, D., Gao, T., and Yu, Y. (2020). Dags with no fears:
A closer look at continuous optimization for learning
bayesian networks. arXiv preprint arXiv:2010.09133.
Xin, D., Ma, L., Liu, J., Macke, S., Song, S., and
Parameswaran, A. (2018). Accelerating human-in-
the-loop machine learning: Challenges and opportu-
nities. In Proceedings of the second workshop on data
management for end-to-end machine learning, pages
1–4.
Yang, Y., Kandogan, E., Li, Y., Sen, P., and Lasecki, W. S.
(2019). A study on interaction in human-in-the-loop
machine learning for text analytics. In IUI Workshops.
Yu, Y., Chen, J., Gao, T., and Yu, M. (2019). DAG-GNN:
DAG structure learning with graph neural networks.
In International Conference on Machine Learning,
pages 7154–7163. PMLR.
Zadrozny, B. (2004). Learning and evaluating classi-
fiers under sample selection bias. In Proceedings of
the twenty-first international conference on Machine
learning, page 114.
Zhang, K., Zhu, S., Kalander, M., Ng, I., Ye, J., Chen, Z.,
and Pan, L. (2021). gcastle: A python toolbox for
causal discovery. arXiv preprint arXiv:2111.15155.
Zheng, X., Aragam, B., Ravikumar, P., and Xing, E. P.
(2018). DAGs with no tears: Continuous op-
timization for structure learning. arXiv preprint
arXiv:1803.01422.
Zheng, X., Dan, C., Aragam, B., Ravikumar, P., and Xing,
E. (2020). Learning sparse nonparametric DAGs.
In International Conference on Artificial Intelligence
and Statistics, pages 3414–3425. PMLR.
ICPRAM 2023 - 12th International Conference on Pattern Recognition Applications and Methods
144