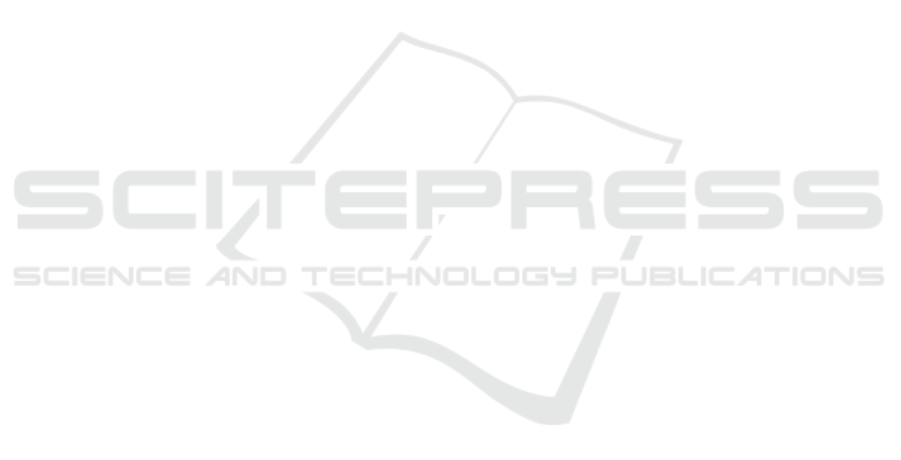
process (Taj-Eddin et al., 2017), aiming to monitor
plants.
Motivated by the above, we propose a robust mo-
tion amplification method to reveal leaf movements
that are not perceptible by the naked eye. The video
magnification approach assumes that the object of in-
terest has small motions and background regions re-
main still (Le Ngo and Phan, 2020; Elgharib et al.,
2015). Thus, video stabilization is a crucial step in
this magnification process to reach accurate results.
We apply video stabilization as a preprocess to re-
move the undesirable large handshake based on a ro-
bust feature trajectories algorithm (Ken-Yi Lee et al.,
2009). Moreover, we utilize leaf extraction and mask
to segment the region of interest (ROI) and apply
magnification to only this region, achieving higher
motion magnification quality. We added a plant seg-
mentation algorithm (Riehle et al., 2020) to obtain an
appropriate segmentation for our approach.
The system proposed by this paper can visualize,
with a smartphone camera, without any additional in-
strument, the small leaf movements of the plant in
a non-destructive manner. Such movements may be
associated with the growth rate of the plant, help-
ing researchers and farmers to investigate and design
control systems based on faster metric acquisitions.
We also explore the magnification bounds and robust-
ness of our approach to provide a measurement tool
for controlled plant environments, where the different
aforementioned plant assessment systems can benefit
from our method.
In summary, the main contributions of this paper
include the proposed architecture system with special
target to magnify small motions of plants using a low-
cost setup, and its robustness and performance eval-
uation. We show that the proposed architecture at-
tenuates considerable distortions related to the back-
ground noise or shaking artifacts, and increases the
magnification quality when compared with a standard
Eulerian phase-based technique (Wu et al., 2012).
The dataset and its acquisition parameters are pro-
vided in a public repository (Pena et al., 2022).
The paper is organized as follows. In Section 2 the
traditional phase-based motion magnification is dis-
cussed. Section 3 describes the proposed system and
its architecture, presenting all steps to visualize the
amplified plant motions. Experimental results are pre-
sented in Section 4, with bound evaluation, and com-
parisons between real and artificial plants. Finally, in
Section 5, we present our final remarks.
2 EULERIAN PHASE-BASED
METHOD
The Eulerian phase-based method (Wadhwa et al.,
2013) is a technique to magnify the small changes in
short videos. The algorithm amplifies the phase dif-
ference value computed between the current and first
frames. Initially, the video is decomposed in spatial
frequencies by a complex-valued steerable pyramid.
This pyramid allows us to measure and modify local
motions since its filters have an impulsive response
with finite spatial support. Then, the local phase rep-
resented by the spatial scale and orientation of the
steerable pyramid is isolated to a specific temporal
frequency by using a temporal bandpass filter. The
frame I
ω
(x,t) of the complex steerable pyramid, fil-
tered for frequency ω, has a local displacement δ(t)
modeled as an intensity of complex sinusoid, given
by
I
ω
(x,t) = A
ω
e
jω(x+δ(t))
. (1)
The temporal filter removes the DC component
and allows the amplification of the displacement δ(t)
by a factor α for each sub-band, producing αδ(t) pix-
els of shift. The sub-band, with the increase in phase
is given by
ˆ
I
ω
(x,t) = A
ω
e
jω(x+(1+α)δ(t))
. (2)
3 PROPOSED METHOD
The proposed method aims to amplify plant move-
ments in a shorter duration than many days of time-
lapse acquisitions. We claim that in short video ac-
quisition, we can highlight small plant movements
for analysis. The architecture is separated into three
steps, described in Figure 1, as: 1) The frames are
stabilized using point feature matching. 2) We mag-
nify the video motion using a modified phase-based
Eulerian technique. 3) We use a mask based on the
leaf segmentation technique, applying the magnifica-
tion only to the ROI during the magnification.
3.1 Stabilization Process
The stabilization process is essential to avoid artifacts
or amplification of the background shaking in the mo-
tion magnification stage. First, interest points are
identified in the frame by corner detection using the
features-from-accelerated-segment test (FAST) algo-
rithm (Rosten and Drummond, 2006). Then, we esti-
mate a transformation that corrects the distortion be-
A Low-Cost Process for Plant Motion Magnification for Smart Indoor Farming
379