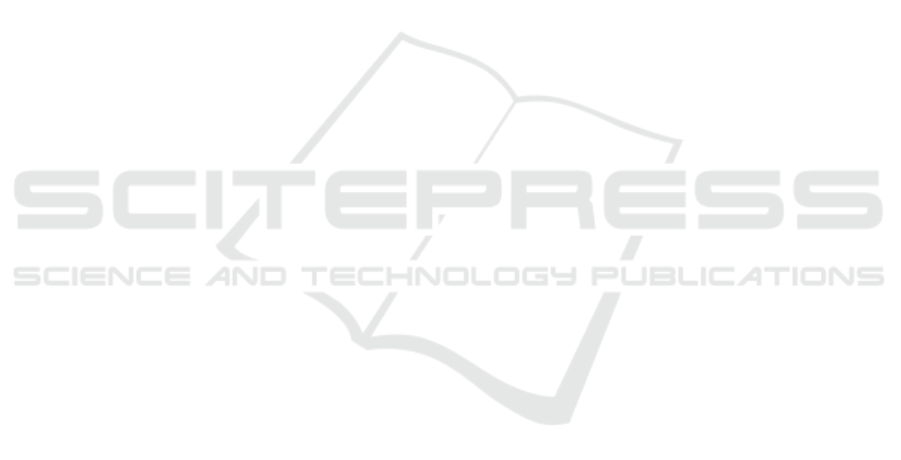
Learner’s Evaluation. Most of students declared
that the recommendations were helpful and suited
(section 4.2.3), however we did not observed higher
following rates in learning analysis. The survey was
proposed to all students having faced the recommen-
dation system however only 49 answered. It can be
explained because most students do not use the ap-
plication regularly and may not accessed the survey.
That highlights the difficulty of developing a recom-
mendation system and finding an evaluation criteria
for this context.
6 CONCLUSION
We propose a recommendation system to improve
navigation in a mobile application through different
chapters and different grade levels. This system relies
on a notions graph to link chapters, uses IRT method
to assign each student a working strategy (revision,
continuation or deepening) to filter content and build
a pedagogical score to rank chapters by relevancy. It
is designed for a micro-learning use and to encourage
autonomous learning through the different grade lev-
els. The system is currently implemented and used
by students. Pedagogical experts approved the rec-
ommendation made for the revision and continuation
strategies, and the users evaluated the system as help-
ful and suitable. Interviewing more experts will help
to consolidate our findings and to perform some sta-
tistical analysis on the given marks. The deepen-
ing strategy seems to be more debatable mainly ex-
plained by the reluctance (of experts and students) on
the use and role of an extracurricular application to
discover coming years program. Concerning the ex-
perts recommendations not prefiltered by the system
or those not in top three (Figure 4), we could look
for a method to enhance or correct our notions graph.
We assume that some students reject the recommen-
dation because their were assigned to the wrong strat-
egy. Analysis of students behaviour from learning
traces will give the possibility to improve the system
to define the best strategy for each learner. We aim
to analyse deeper how learners use our recommenda-
tions, to consider students choices to improve the sys-
tem knowledge, and to try our system on other sub-
jects.
REFERENCES
Baker, F. B. (2001). The Basics of Item Response Theory.
ERIC Clearinghouse on Assessment and Evaluation.
Baker, R., Ma, W., Zhao, Y., Wang, S., and Ma, Z. (2020).
The results of implementing zone of proximal devel-
opment on learning outcomes. In The 13th Interna-
tional Conference on Educational Data Mining.
Bloom, B. (1956). Taxonomy of educational objectives: The
classification of educational goals.
Branth
ˆ
ome, M. (2022). Pyrates: A Serious Game De-
signed to Support the Transition from Block-Based to
Text-Based Programming. Educating for a New Fu-
ture: Making Sense of Technology-Enhanced Learn-
ing Adoption, 13450:31–44.
Corbett, A. T. and Anderson, J. R. (1994). Knowledge trac-
ing: Modeling the acquisition of procedural knowl-
edge. User Modeling and User-Adapted Interaction.
Daher, J. B., Brun, A., and Boyer, A. (2018). Multi-source
Data Mining for e-Learning. In 7th International Sym-
posium “From Data to Models and Back (DataMod)”.
De Maio, C., Fenza, G., Gaeta, M., Loia, V., Orciuoli, F.,
and Senatore, S. (2012). RSS-based e-learning rec-
ommendations exploiting fuzzy FCA for Knowledge
Modeling. Applied Soft Computing, 12(1):113–124.
Erdt, M., Fernandez, A., and Rensing, C. (2015). Evaluat-
ing Recommender Systems for Technology Enhanced
Learning: A Quantitative Survey. IEEE Transactions
on Learning Technologies, 8(4):326–344.
Guruge, D. B., Kadel, R., and Halder, S. J. (2021). The State
of the Art in Methodologies of Course Recommender
SystemsA Review of Recent Research. Data, 6(2):18.
Nguyen, C., Roussanaly, A., and Boyer, A. (2014). Learn-
ing Resource Recommendation: An Orchestration of
Content-Based Filtering, Word Semantic Similarity
and Page Ranking. In 9th European Conference on
Technology Enhanced Learning, EC-TEL 2014.
Nikou, S. and Economides, A. (2018). Mobile-Based
micro-Learning and Assessment: Impact on learning
performance and motivation of high school students.
Journal of Computer Assisted Learning, 34(3).
Reich, J. (2014). MOOC Completion and Retention in the
Context of Student Intent. Educause Review.
Rizun, M. (2019). Knowledge Graph Application in Educa-
tion: A Literature Review. Acta Universitatis Lodzien-
sis. Folia Oeconomica, 3(342):7–19.
Vaidhehi, V. and Suchithra, R. (2018). A Systematic Review
of Recommender Systems in Education. International
Journal of Engineering & Technology, 7:188.
Vie, J.-J. and Kashima, H. (2019). Knowledge Tracing Ma-
chines: Factorization Machines for Knowledge Trac-
ing. In Proceedings of the AAAI Conference on Artifi-
cial Intelligence.
Vygotski
˘
ı, L. S. and Cole, M. (1978). Mind in Society:
The Development of Higher Psychological Processes.
Harvard University Press.
Wauters, K., Desmet, P., and den Noortgate, W. V. (2021).
Adaptive item-based learning environments based on
the item response theory: Possibilities and challenges.
Journal of Computer Assisted Learning, 26(6).
Recommendation Model for an After-School E-learning Mobile Application
87