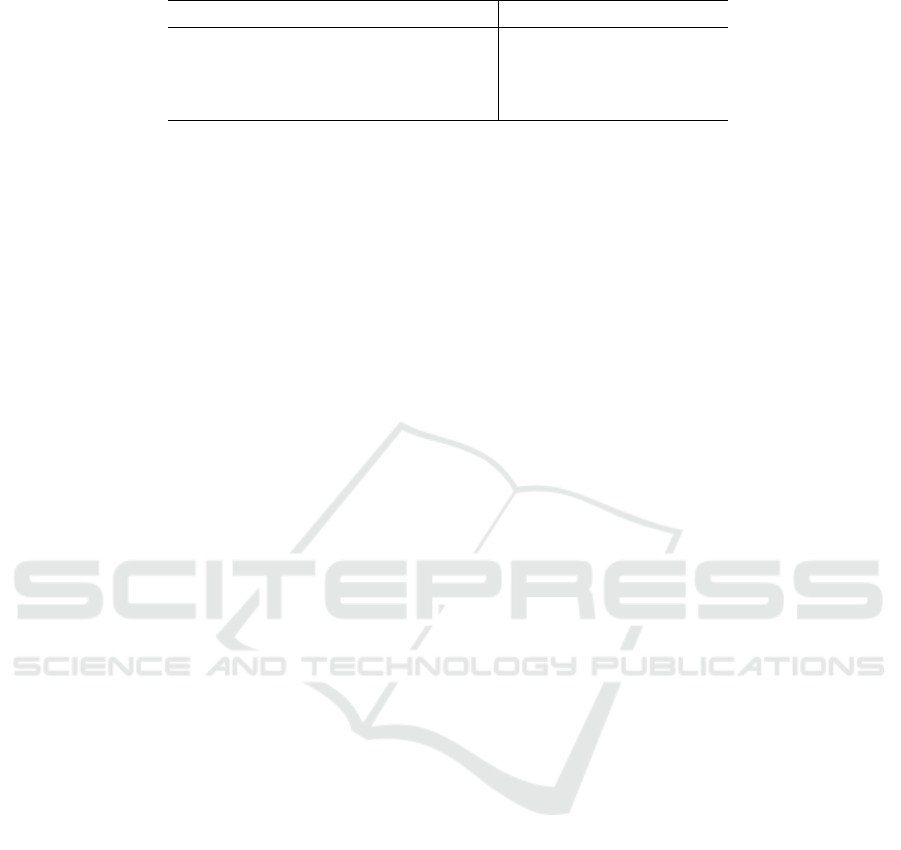
Table 3: Ablation study on Places2 dataset.†Lower is better. ∗Higher is better.
Basenet Structure LFN MFMN l
†
1
(%) SSIM
∗
PSNR
∗
✓ ✓ 1.6 0.766 26.01
✓ ✓ 1.4 0.825 27.52
✓ ✓ ✓ 1.5 0.81 27.77
✓ ✓ ✓ ✓ 1.3 0.835 27.98
REFERENCES
Ballester, C., Bertalmio, M., Caselles, V., Sapiro, G., and
Verdera, J. (2001). Filling-in by joint interpolation of
vector fields and gray levels. IEEE transactions on
image processing, 10(8):1200–1211.
Barnes, C., Shechtman, E., Finkelstein, A., and Goldman,
D. B. (2009). Patchmatch: A randomized correspon-
dence algorithm for structural image editing. ACM
Trans. Graph., 28(3):24.
Bertalmio, M., Sapiro, G., Caselles, V., and Ballester, C.
(2000). Image inpainting. In Proceedings of the 27th
annual conference on Computer graphics and inter-
active techniques, pages 417–424.
Chan, T. F. and Shen, J. (2001). Nontexture inpainting by
curvature-driven diffusions. Journal of visual commu-
nication and image representation, 12(4):436–449.
Criminisi, A., P
´
erez, P., and Toyama, K. (2004). Region
filling and object removal by exemplar-based image
inpainting. IEEE Transactions on image processing,
13(9):1200–1212.
Darabi, S., Shechtman, E., Barnes, C., Goldman, D. B., and
Sen, P. (2012). Image melding: Combining inconsis-
tent images using patch-based synthesis. ACM Trans-
actions on graphics (TOG), 31(4):1–10.
Esedoglu, S. and Shen, J. (2002). Digital inpainting based
on the mumford–shah–euler image model. European
Journal of Applied Mathematics, 13(4):353–370.
Guo, X., Yang, H., and Huang, D. (2021a). Image inpaint-
ing via conditional texture and structure dual genera-
tion. In Proceedings of the IEEE/CVF International
Conference on Computer Vision, pages 14134–14143.
Guo, X., Yang, H., and Huang, D. (2021b). Image inpaint-
ing via conditional texture and structure dual genera-
tion. In Proceedings of the IEEE/CVF International
Conference on Computer Vision, pages 14134–14143.
He, K., Zhang, X., Ren, S., and Sun, J. (2016). Deep resid-
ual learning for image recognition. In Proceedings of
the IEEE conference on computer vision and pattern
recognition, pages 770–778.
Iizuka, S., Simo-Serra, E., and Ishikawa, H. (2017). Glob-
ally and locally consistent image completion. ACM
Transactions on Graphics (ToG), 36(4):1–14.
Karras, T., Aila, T., Laine, S., and Lehtinen, J. (2017). Pro-
gressive growing of gans for improved quality, stabil-
ity, and variation. arXiv preprint arXiv:1710.10196.
Li, J., Wang, N., Zhang, L., Du, B., and Tao, D. (2020). Re-
current feature reasoning for image inpainting. In Pro-
ceedings of the IEEE/CVF Conference on Computer
Vision and Pattern Recognition, pages 7760–7768.
Liu, G., Reda, F. A., Shih, K. J., Wang, T.-C., Tao, A., and
Catanzaro, B. (2018). Image inpainting for irregular
holes using partial convolutions. In Proceedings of
the European conference on computer vision (ECCV),
pages 85–100.
Miyato, T., Kataoka, T., Koyama, M., and Yoshida, Y.
(2018). Spectral normalization for generative adver-
sarial networks. arXiv preprint arXiv:1802.05957.
Nazeri, K., Ng, E., Joseph, T., Qureshi, F., and Ebrahimi,
M. (2019). Edgeconnect: Structure guided image in-
painting using edge prediction. In Proceedings of the
IEEE/CVF International Conference on Computer Vi-
sion Workshops, pages 0–0.
Pathak, D., Krahenbuhl, P., Donahue, J., Darrell, T., and
Efros, A. A. (2016). Context encoders: Feature learn-
ing by inpainting. In Proceedings of the IEEE con-
ference on computer vision and pattern recognition,
pages 2536–2544.
Peng, J., Liu, D., Xu, S., and Li, H. (2021). Generating di-
verse structure for image inpainting with hierarchical
vq-vae. In Proceedings of the IEEE/CVF Conference
on Computer Vision and Pattern Recognition, pages
10775–10784.
Ren, Y., Yu, X., Zhang, R., Li, T. H., Liu, S., and
Li, G. (2019). Structureflow: Image inpainting via
structure-aware appearance flow. In Proceedings of
the IEEE/CVF International Conference on Computer
Vision, pages 181–190.
Ronneberger, O., Fischer, P., and Brox, T. (2015). U-net:
Convolutional networks for biomedical image seg-
mentation. In International Conference on Medical
image computing and computer-assisted intervention,
pages 234–241. Springer.
Shen, J. and Chan, T. F. (2002). Mathematical models for
local nontexture inpaintings. SIAM Journal on Ap-
plied Mathematics, 62(3):1019–1043.
Simonyan, K. and Zisserman, A. (2014). Very deep con-
volutional networks for large-scale image recognition.
arXiv preprint arXiv:1409.1556.
Suvorov, R., Logacheva, E., Mashikhin, A., Remizova, A.,
Ashukha, A., Silvestrov, A., Kong, N., Goka, H., Park,
K., and Lempitsky, V. (2022). Resolution-robust large
mask inpainting with fourier convolutions. In Pro-
ceedings of the IEEE/CVF Winter Conference on Ap-
plications of Computer Vision, pages 2149–2159.
Wang, T., Ouyang, H., and Chen, Q. (2021). Image inpaint-
ing with external-internal learning and monochromic
bottleneck. In Proceedings of the IEEE/CVF Con-
ference on Computer Vision and Pattern Recognition,
pages 5120–5129.
ICPRAM 2023 - 12th International Conference on Pattern Recognition Applications and Methods
154