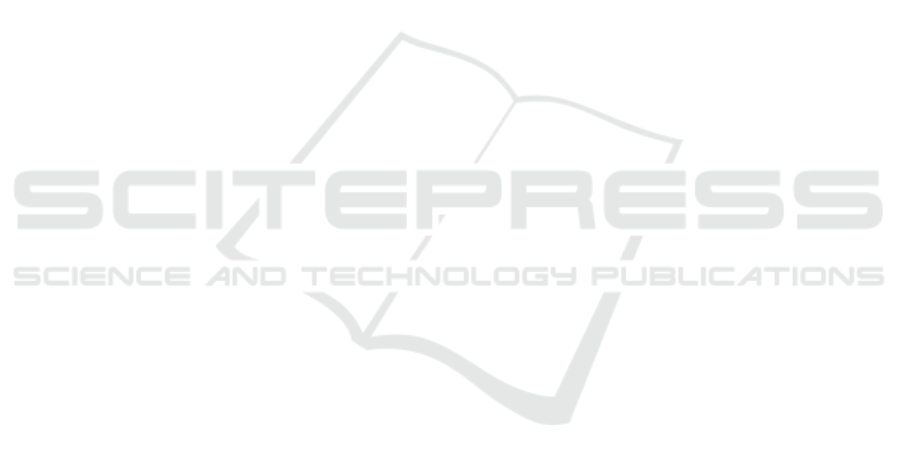
Katam, H. (2019). Blenderproc. arXiv preprint
arXiv:1911.01911.
Eigen, D. and Fergus, R. (2015). Predicting depth, surface
normals and semantic labels with a common multi-
scale convolutional architecture. 2015 IEEE Interna-
tional Conference on Computer Vision (ICCV), pages
2650–2658.
Engel, N., Belagiannis, V., and Dietmayer, K. C. J. (2021).
Point transformer. IEEE Access, 9:134826–134840.
Everingham, M., Gool, L. V., Williams, C. K. I., Winn,
J. M., and Zisserman, A. (2009). The pascal visual
object classes (voc) challenge. International Journal
of Computer Vision, 88:303–338.
Gao, Y., She, Q., Ma, J., Zhao, M., Liu, W., and Yuille,
A. L. (2019). Nddr-cnn: Layerwise feature fusing in
multi-task cnns by neural discriminative dimensional-
ity reduction. 2019 IEEE/CVF Conference on Com-
puter Vision and Pattern Recognition (CVPR), pages
3200–3209.
Geiger, A., Lenz, P., and Urtasun, R. (2012). Are we ready
for autonomous driving? the kitti vision benchmark
suite. 2012 IEEE Conference on Computer Vision and
Pattern Recognition, pages 3354–3361.
Griffiths, D. and Boehm, J. (2019). Synthcity: A large scale
synthetic point cloud. ArXiv, abs/1907.04758.
Gschwandtner, M., Kwitt, R., Uhl, A., and Pree, W. (2011).
Blensor: Blender sensor simulation toolbox. In In-
ternational Symposium on Visual Computing, pages
199–208. Springer.
Guo, M.-H., Cai, J., Liu, Z.-N., Mu, T.-J., Martin, R. R., and
Hu, S. (2021). Pct: Point cloud transformer. Comput.
Vis. Media, 7:187–199.
Huang, G. B., Mattar, M., Berg, T., and Learned-Miller,
E. (2008). Labeled faces in the wild: A database
for studying face recognition in unconstrained envi-
ronments. In Workshop on faces in’Real-Life’Images:
detection, alignment, and recognition.
Jiang, M., Wu, Y., Zhao, T., Zhao, Z., and Lu, C.
(2018). Pointsift: A sift-like network module for 3d
point cloud semantic segmentation. arXiv preprint
arXiv:1807.00652.
Kalayeh, M. M., Gong, B., and Shah, M. (2017). Improv-
ing facial attribute prediction using semantic segmen-
tation. 2017 IEEE Conference on Computer Vision
and Pattern Recognition (CVPR), pages 4227–4235.
Kendall, A., Gal, Y., and Cipolla, R. (2018). Multi-task
learning using uncertainty to weigh losses for scene
geometry and semantics. 2018 IEEE/CVF Conference
on Computer Vision and Pattern Recognition, pages
7482–7491.
Khan, S., Phan, B., Salay, R., and Czarnecki, K. (2019).
Procsy: Procedural synthetic dataset generation to-
wards influence factor studies of semantic segmenta-
tion networks. In CVPRW, pages 88–96.
Koch, S., Matveev, A., Jiang, Z., Williams, F., Artemov, A.,
Burnaev, E., Alexa, M., Zorin, D., and Panozzo, D.
(2019). Abc: A big cad model dataset for geometric
deep learning. CVPR, pages 9593–9603.
Kokkinos, I. (2017). Ubernet: Training a universal con-
volutional neural network for low-, mid-, and high-
level vision using diverse datasets and limited mem-
ory. 2017 IEEE Conference on Computer Vision and
Pattern Recognition (CVPR), pages 5454–5463.
Lawin, F. J., Danelljan, M., Tosteberg, P., Bhat, G., Khan,
F. S., and Felsberg, M. (2017). Deep projective 3d
semantic segmentation. In International Conference
on Computer Analysis of Images and Patterns, pages
95–107. Springer.
Le Hoang-An, Mensink, T., Das, P., Karaoglu, S., and Gev-
ers, T. (2021). Eden: Multimodal synthetic dataset
of enclosed garden scenes. 2021 IEEE Winter Con-
ference on Applications of Computer Vision (WACV),
pages 1578–1588.
Li, D., Chen, X., and Huang, K. (2015). Multi-attribute
learning for pedestrian attribute recognition in surveil-
lance scenarios. 2015 3rd IAPR Asian Conference on
Pattern Recognition (ACPR), pages 111–115.
Li, D., Chen, X., Zhang, Z., and Huang, K. (2018). Pose
guided deep model for pedestrian attribute recognition
in surveillance scenarios. 2018 IEEE International
Conference on Multimedia and Expo (ICME), pages
1–6.
Liang, Z., Yang, M., Li, H., and Wang, C. (2020). 3d in-
stance embedding learning with a structure-aware loss
function for point cloud segmentation. IEEE Robotics
and Automation Letters, 5:4915–4922.
Lin, T.-Y., Maire, M., Belongie, S. J., Hays, J., Perona, P.,
Ramanan, D., Doll
´
ar, P., and Zitnick, C. L. (2014).
Microsoft coco: Common objects in context. In
ECCV.
Liu, Y., Wei, F., Shao, J., Sheng, L., Yan, J., and Wang,
X. (2018). Exploring disentangled feature representa-
tion beyond face identification. 2018 IEEE/CVF Con-
ference on Computer Vision and Pattern Recognition,
pages 2080–2089.
Liu, Z., Luo, P., Wang, X., and Tang, X. (2015). Deep learn-
ing face attributes in the wild. 2015 IEEE Interna-
tional Conference on Computer Vision (ICCV), pages
3730–3738.
Maturana, D. and Scherer, S. (2015). Voxnet: A 3d con-
volutional neural network for real-time object recog-
nition. In 2015 IEEE/RSJ International Conference
on Intelligent Robots and Systems (IROS), pages 922–
928. IEEE.
Misra, I., Shrivastava, A., Gupta, A. K., and Hebert, M.
(2016). Cross-stitch networks for multi-task learning.
2016 IEEE Conference on Computer Vision and Pat-
tern Recognition (CVPR), pages 3994–4003.
Mo, K., Zhu, S., Chang, A. X., Yi, L., Tripathi, S., Guibas,
L. J., and Su, H. (2019). Partnet: A large-scale bench-
mark for fine-grained and hierarchical part-level 3d
object understanding. 2019 IEEE/CVF Conference
on Computer Vision and Pattern Recognition (CVPR),
pages 909–918.
Pierdicca, R., Mameli, M., Malinverni, E., Paolanti, M., and
Frontoni, E. (2019). Automatic generation of point
cloud synthetic dataset for historical building repre-
sentation. In AVR, pages 203–219.
Qi, C., Su, H., Mo, K., and Guibas, L. (2017a). Pointnet:
SynMotor: A Benchmark Suite for Object Attribute Regression and Multi-Task Learning
539