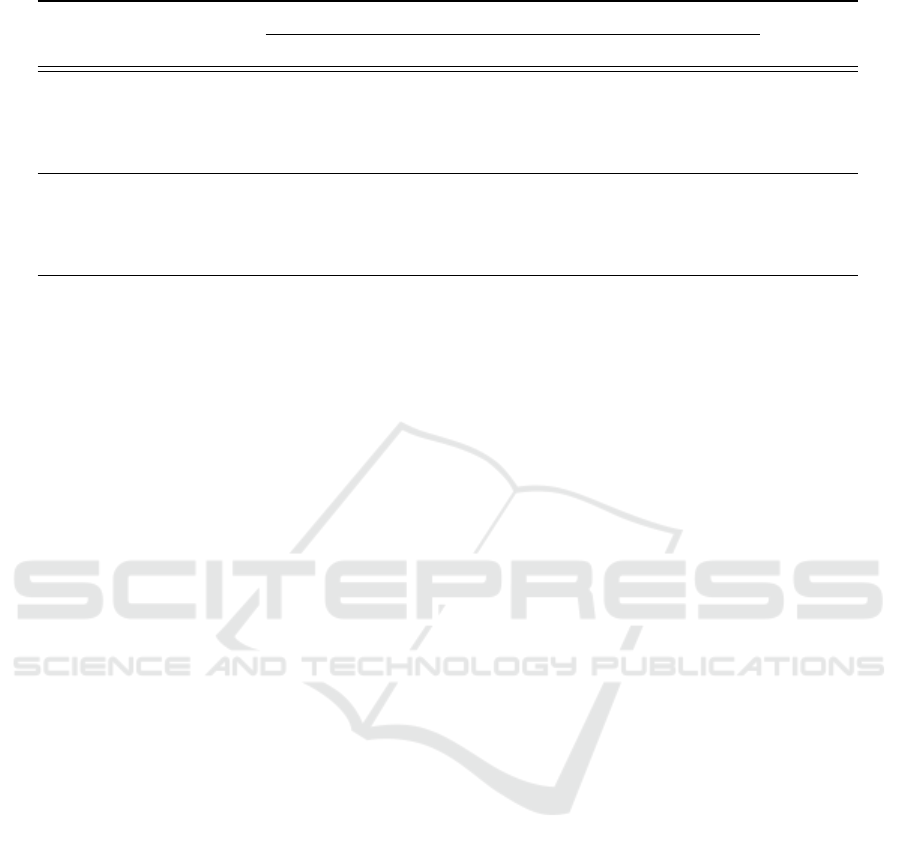
Table 3: Comparison our proposed method and baseline on COVID-19 dataset.
IoU(%)
Method Background Lungs other Ground glass Consolidations mIoU(%)
2 classes U-net(EN-b7)
93.14 - - - -
- 33.88 - - -
- - 49.22 - -
- - - 7.40 -
4 classes
U-net 92.41 30.18 41.23 2.44 41.57
U-net(EN-b7) 95.04 34.37 46.45 5.86 45.43
U-net + KD 96.11 37.83 47.72 0.00 45.42
U-net + ours 95.38 37.06 48.13 6.30 46.72
the proposed method is able to inference with higher
accuracy than the respective baselines. This results
show that our proposed method is effective even for
classes that are difficult to inference.
Experiments on two datasets demonstrated that
our proposed class-wise distillation method is more
effective in distilling knowledge for inference than
conventional knowledge distillation methods.
5 CONCLUSIONS
In this paper, we proposed a new class-wise knowl-
edge distillation method for multi-class semantic seg-
mentation. Specifically, knowledge is transferred
from teacher models specialized for each class to
a student model. This enables better knowledge
transmission than conventional knowledge distillation
methods. By using this method, the student model
achieved higher accuracy than the student model
trained by conventional knowledge distillation meth-
ods.
In the future, we would like to make learning more
effective without increasing computational resources.
ACKNOWLEDGEMENTS
This work is supported by SCAT Foundation and
KAKENHI Grant Number 22H04735.
REFERENCES
Badrinarayanan, V., Kendall, A., and Cipolla, R. (2017).
Segnet: A deep convolutional encoder-decoder archi-
tecture for image segmentation. IEEE transactions on
pattern analysis and machine intelligence.
Cao, Z., Hidalgo, G., Simon, T., Wei, S.-E., and Sheikh,
Y. (2018). Openpose: realtime multi-person 2d pose
estimation using part affinity fields. arXiv preprint
arXiv:1812.08008.
Chen, L.-C., Papandreou, G., Kokkinos, I., Murphy, K., and
Yuille, A. L. (2017). Deeplab: Semantic image seg-
mentation with deep convolutional nets, atrous convo-
lution, and fully connected crfs. IEEE transactions on
pattern analysis and machine intelligence.
Cordts, M., Omran, M., Ramos, S., Rehfeld, T., Enzweiler,
M., Benenson, R., Franke, U., Roth, S., and Schiele,
B. (2016). The cityscapes dataset for semantic urban
scene understanding. In Proceedings of the IEEE con-
ference on computer vision and pattern recognition.
Gerhard, S., Funke, J., Martel, J., Cardona, A., and Fet-
ter, R. (2013). Segmented anisotropic sstem dataset of
neural tissue. figshare.
Han, S., Mao, H., and Dally, W. J. (2015). Deep compres-
sion: Compressing deep neural networks with prun-
ing, trained quantization and huffman coding. arXiv
preprint arXiv:1510.00149.
He, K., Zhang, X., Ren, S., and Sun, J. (2016). Deep resid-
ual learning for image recognition. In Proceedings of
the IEEE conference on computer vision and pattern
recognition.
Hinton, G., Vinyals, O., Dean, J., et al. (2015). Distilling
the knowledge in a neural network. arXiv preprint
arXiv:1503.02531.
Hu, J., Shen, L., and Sun, G. (2018). Squeeze-and-
excitation networks. In Proceedings of the IEEE con-
ference on computer vision and pattern recognition.
Hubara, I., Courbariaux, M., Soudry, D., El-Yaniv, R., and
Bengio, Y. (2016). Binarized neural networks. Ad-
vances in neural information processing systems.
Liu, W., Anguelov, D., Erhan, D., Szegedy, C., Reed, S.,
Fu, C.-Y., and Berg, A. C. (2016). Ssd: Single shot
multibox detector. In European conference on com-
puter vision. Springer.
Liu, Y., Chen, K., Liu, C., Qin, Z., Luo, Z., and Wang, J.
(2019). Structured knowledge distillation for seman-
tic segmentation. In Proceedings of the IEEE/CVF
Conference on Computer Vision and Pattern Recogni-
tion.
Ronneberger, O., Fischer, P., and Brox, T. (2015). U-net:
Convolutional networks for biomedical image seg-
mentation. In International Conference on Medical
BIOSIGNALS 2023 - 16th International Conference on Bio-inspired Systems and Signal Processing
292